Cross-Geography Generalization of Machine Learning Methods for Classification of Flooded Regions in Aerial Images
CoRR(2022)
摘要
Identification of regions affected by floods is a crucial piece of information required for better planning and management of post-disaster relief and rescue efforts. Traditionally, remote sensing images are analysed to identify the extent of damage caused by flooding. The data acquired from sensors onboard earth observation satellites are analyzed to detect the flooded regions, which can be affected by low spatial and temporal resolution. However, in recent years, the images acquired from Unmanned Aerial Vehicles (UAVs) have also been utilized to assess post-disaster damage. Indeed, a UAV based platform can be rapidly deployed with a customized flight plan and minimum dependence on the ground infrastructure. This work proposes two approaches for identifying flooded regions in UAV aerial images. The first approach utilizes texture-based unsupervised segmentation to detect flooded areas, while the second uses an artificial neural network on the texture features to classify images as flooded and non-flooded. Unlike the existing works where the models are trained and tested on images of the same geographical regions, this work studies the performance of the proposed model in identifying flooded regions across geographical regions. An F1-score of 0.89 is obtained using the proposed segmentation-based approach which is higher than existing classifiers. The robustness of the proposed approach demonstrates that it can be utilized to identify flooded regions of any region with minimum or no user intervention.
更多查看译文
关键词
flooded regions,machine learning methods,classification,machine learning,cross-geography
AI 理解论文
溯源树
样例
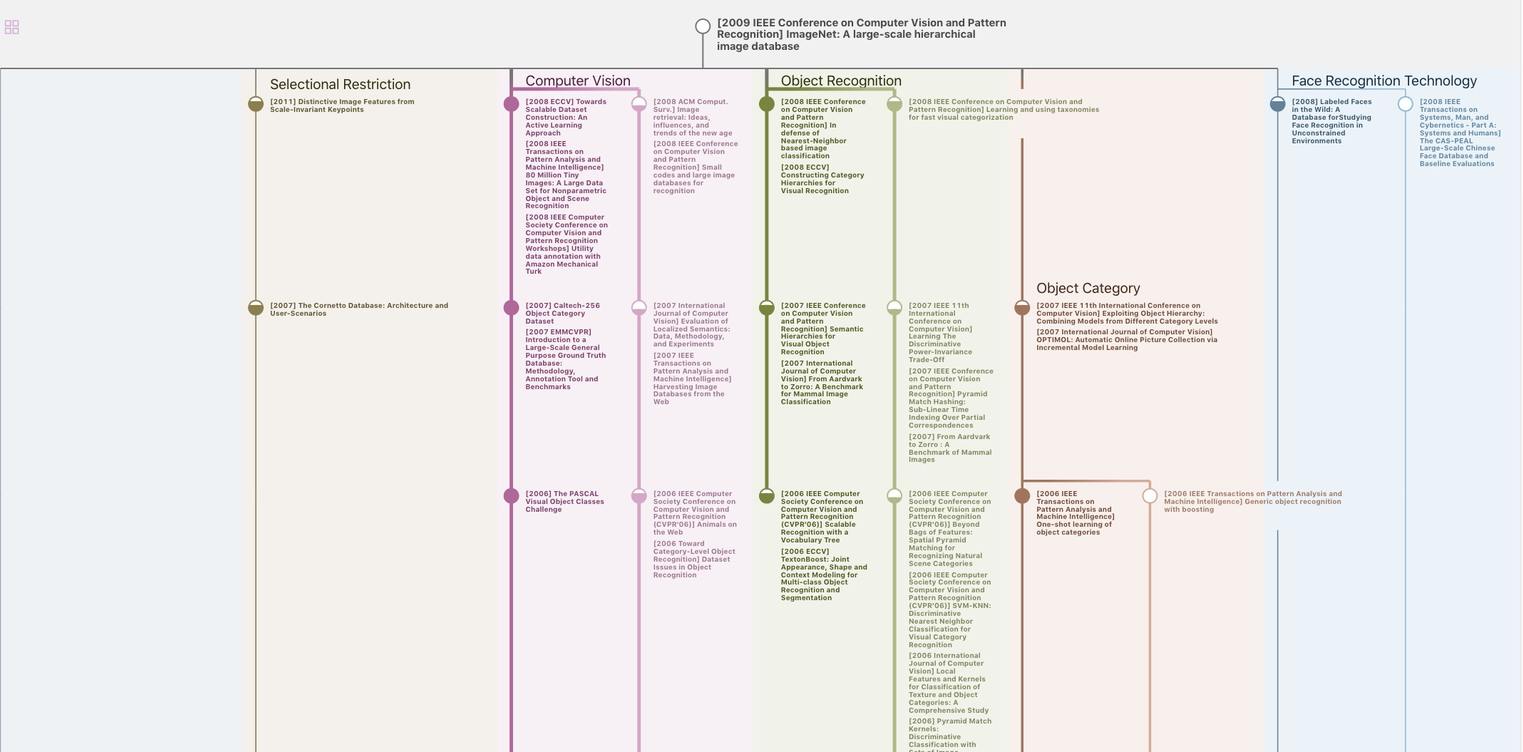
生成溯源树,研究论文发展脉络
Chat Paper
正在生成论文摘要