Cooperative Self-Training for Multi-Target Adaptive Semantic Segmentation
WACV(2023)
摘要
In this work we address multi-target domain adaptation (MTDA) in semantic segmentation, which consists in adapting a single model from an annotated source dataset to multiple unannotated target datasets that differ in their underlying data distributions. To address MTDA, we propose a self-training strategy that employs pseudo-labels to induce cooperation among multiple domain-specific classifiers. We employ feature stylization as an efficient way to generate image views that forms an integral part of selftraining. Additionally, to prevent the network from overfitting to noisy pseudo-labels, we devise a rectification strategy that leverages the predictions from different classifiers to estimate the quality of pseudo-labels. Our extensive experiments on numerous settings, based on four different semantic segmentation datasets, validates the effectiveness of the proposed self-training strategy and shows that our method outperforms state-of-the-art MTDA approaches. https://github.com/Mael-zys/CoaST.
更多查看译文
关键词
self-training,multi-target
AI 理解论文
溯源树
样例
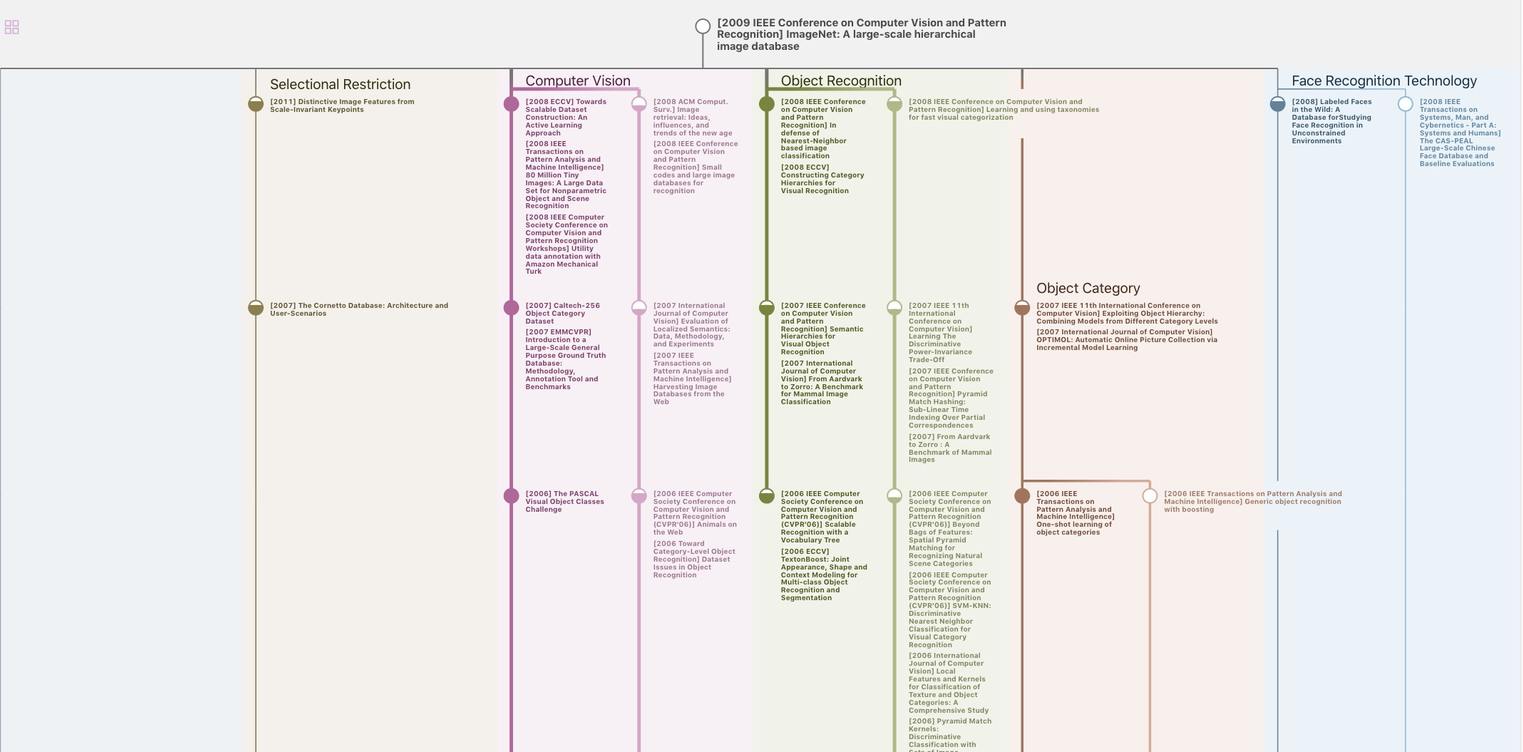
生成溯源树,研究论文发展脉络
Chat Paper
正在生成论文摘要