Probabilistic Volumetric Fusion for Dense Monocular SLAM
WACV(2023)
摘要
We present a novel method to reconstruct 3D scenes from images by leveraging deep dense monocular SLAM and fast uncertainty propagation. The proposed approach is able to 3D reconstruct scenes densely, accurately, and in real-time while being robust to extremely noisy depth estimates coming from dense monocular SLAM. Differently from previous approaches, that either use ad-hoc depth filters, or that estimate the depth uncertainty from RGB-D cameras' sensor models, our probabilistic depth uncertainty derives directly from the information matrix of the underlying bundle adjustment problem in SLAM. We show that the resulting depth uncertainty provides an excellent signal to weight the depth-maps for volumetric fusion. Without our depth uncertainty, the resulting mesh is noisy and with artifacts, while our approach generates an accurate 3D mesh with significantly fewer artifacts. We provide results on the challenging Euroc dataset, and show that our approach achieves 92% better accuracy than directly fusing depths from monocular SLAM, and up to 90% improvements compared to the best competing approach.
更多查看译文
关键词
probabilistic volumetric fusion
AI 理解论文
溯源树
样例
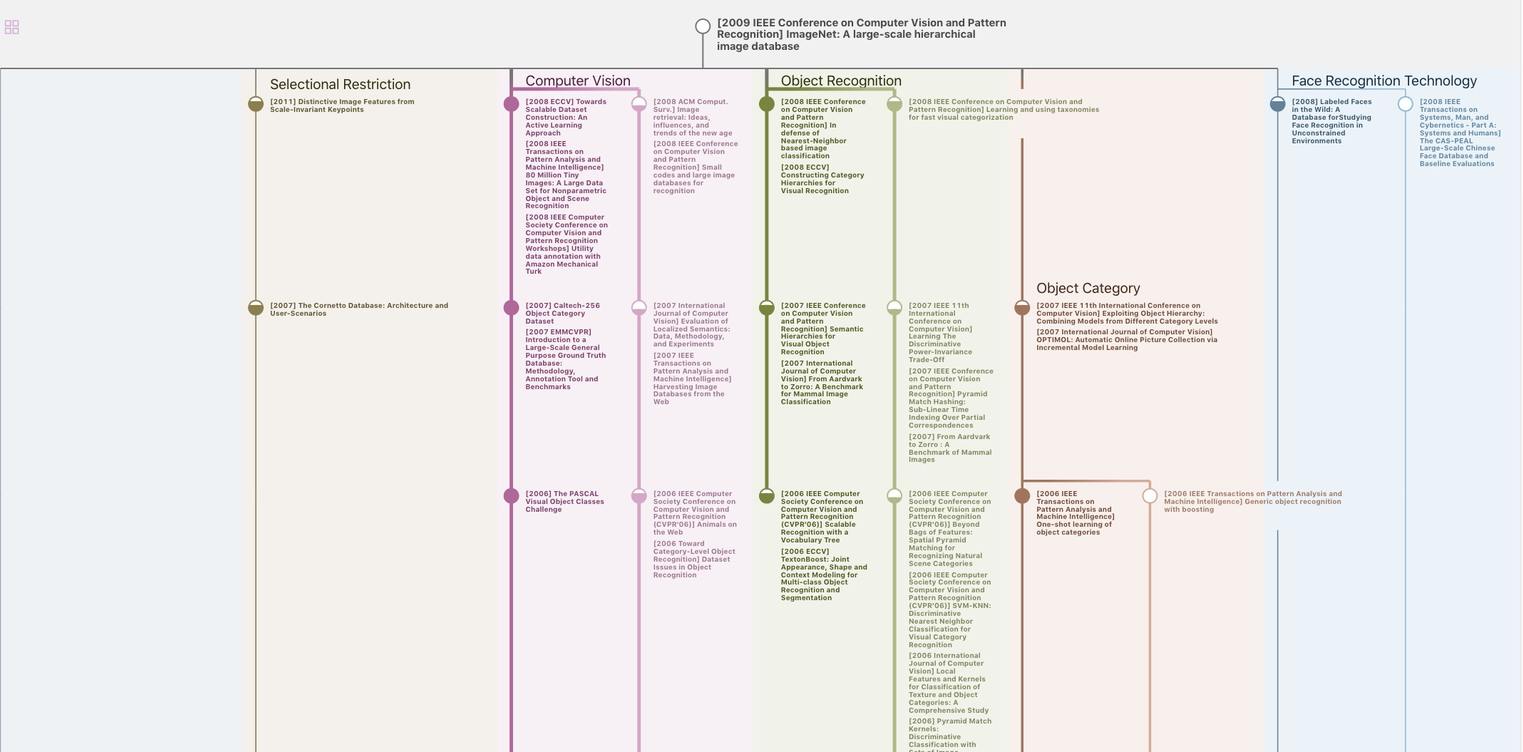
生成溯源树,研究论文发展脉络
Chat Paper
正在生成论文摘要