Machine Learning Based Invariant Generation: A Framework and Reproducibility Study
2022 IEEE Conference on Software Testing, Verification and Validation (ICST)(2022)
摘要
Software verification is the task of proving correct-ness of programs against specified requirements. Key to software verification is the automatic generation of loop invariants. In recent years, template- and logic-based approaches to invariant generation have been complemented by machine learning (ML) techniques. A number of proposals for such techniques exist today. Although all authors perform experimental evaluations of their proposals, comparability of the core techniques is nev-ertheless hindered by differing benchmarks, specific tunings of hyperparameters, missing public availability as well as specialized preprocessings and runtime environments. In this paper, we present the modular framework MIG
ML
for experimentation with and comparison of ML invariant generators. MIG
ML
contains the core ingredients of ML based invariant generators (i.e. a teacher and a learner) as instantiable components with clear-cut interfaces. This conceptually novel framework allows for a reproducibility study of four existing ML invariant generators: we re-implement the teacher and learner components of the four techniques within our framework which permits a comparison on equal grounds. We are able to successfully reproduce and partially confirm the reported results. We furthermore experiment with novel combinations of components, e.g. employ the data generator within the teacher of technique A together with the learner of technique B. As a result, we observe that such combinations can lead to an overall enhanced effectiveness.
更多查看译文
关键词
Software verification,invariant generation,machine learning,reproducibility study
AI 理解论文
溯源树
样例
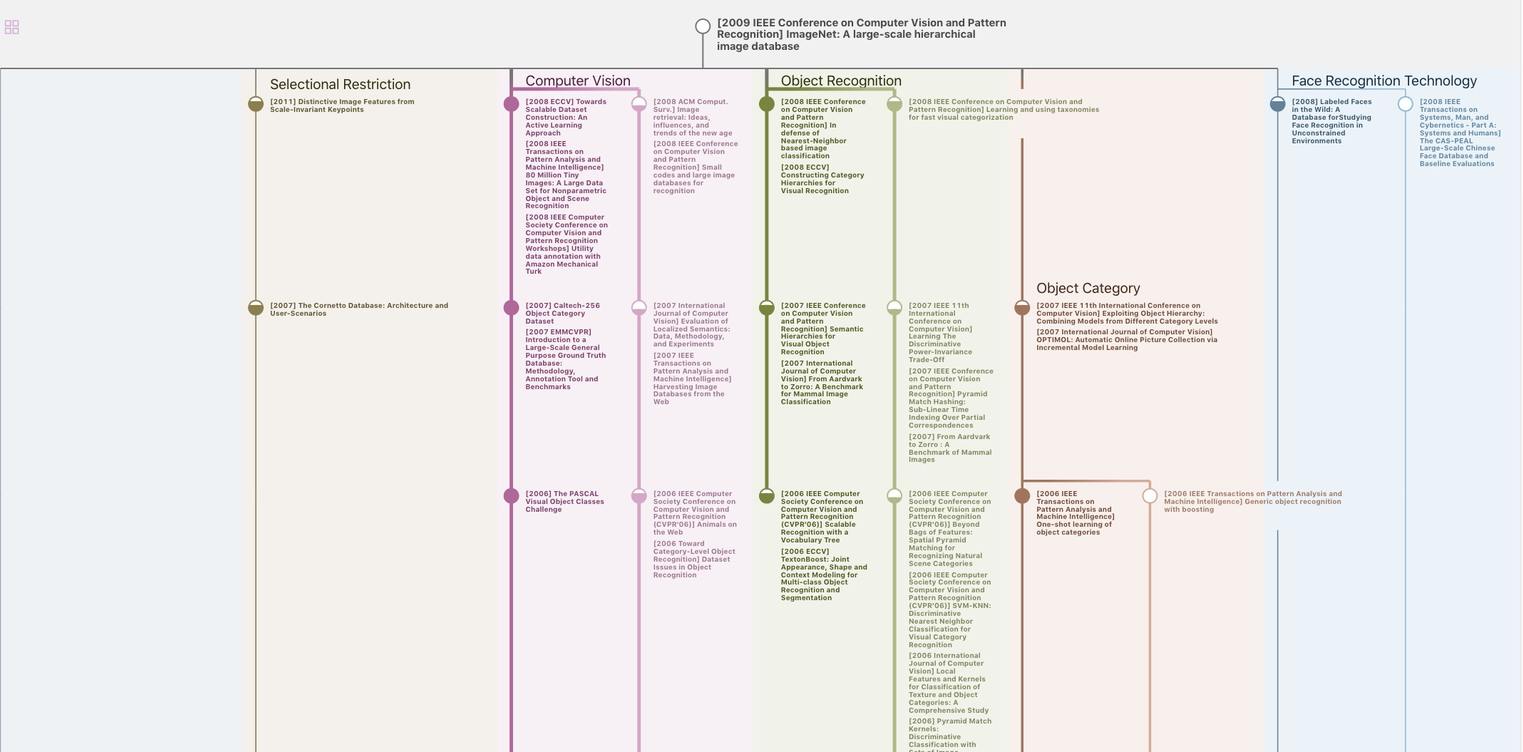
生成溯源树,研究论文发展脉络
Chat Paper
正在生成论文摘要