Seismic Internal Multiple Suppression Based on Convolutional Neural Network
IEEE GEOSCIENCE AND REMOTE SENSING LETTERS(2022)
摘要
Internal multiple suppression remains a key challenge in seismic processing due to the tiny velocity and periodicity difference between primary reflections and internal multiples. Existing methods suppress internal multiples with poor adaptivity and efficiency. Internal multiple suppression based on artificial intelligence (AI) faces challenges about highly demanded computing resources and the lack of sufficient labels. This letter redesigns a convolutional neural network (CNN) scheme for internal multiple suppression in terms of training set construction and input strategy to alleviate these problems. We adopt the virtual event (VE) method to generate a few primary labels and develop an internal multiple augmentation strategy to expand the training set and improve the network performance based on small data set. The U-shaped CNN is trained based on augmented datasets and then applied to new seismic data. During the training and prediction process, the entire seismic data are split into vertical patches as network inputs for less computing resources and better demultipling performance. Tests on synthetic and field data demonstrate that our method effectively suppresses internal multiples and exhibits a competitive performance in comparison with existing methods and original demultipling CNN in efficiency, memory cost, and primary retention ability.
更多查看译文
关键词
Training,Convolutional neural networks,Reflection,Convolution,Feature extraction,Geoscience and remote sensing,Signal to noise ratio,Convolutional neural network (CNN),internal multiple,multiple augmentation,patch strategy,virtual event (VE)
AI 理解论文
溯源树
样例
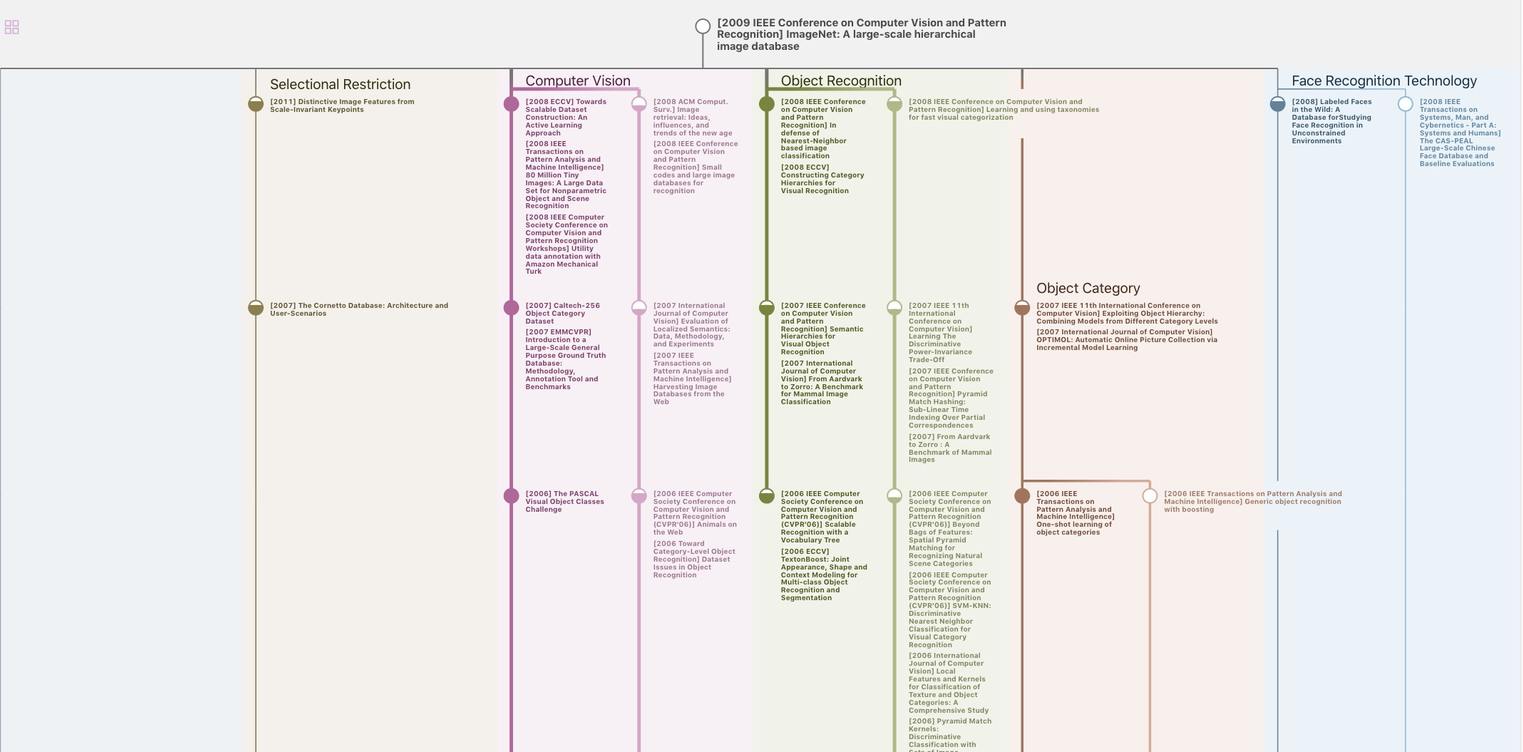
生成溯源树,研究论文发展脉络
Chat Paper
正在生成论文摘要