Literature Classification Research on Fusion of Pretraining Model and Bidirectional Gated Recurrent Unit
2022 5th International Conference on Pattern Recognition and Artificial Intelligence (PRAI)(2022)
摘要
At present, manual literature classification methods are inefficient and rely on expert knowledge, while traditional machine learning literature classification methods need to construct complex feature engineering, and usually use static word embedding methods such as TF-IDF or Word2Vec to represent document text, ignoring context information and the degree of influence of each part of the literature text on the classification results. In response to the above problems, the ERNIE-BiGRU-Attention model is proposed. The model first preprocesses the document index data and then fuses the processed titles, abstracts and keywords into the ERNIE pretraining model to generate corresponding. Then, the model uses the bidirectional gated recurrent unit to extract features and learn semantics. Finally, the attention mechanism is introduced to assign different weights according to the influence of each part of the text on the classification results to complete the classification task. The experiment compares the macro precision rate, macro recall rate, and macro F1 value under different models using the feature input of titles, abstracts, and fusion of titles, abstracts, and keywords respectively. The results show that the proposed model improves the accuracy of literature classification and provides literature classification research with a new idea.
更多查看译文
关键词
literature classification,ERNIE,pre-training model,bidirectional gated recurrent unit,attention mechanism
AI 理解论文
溯源树
样例
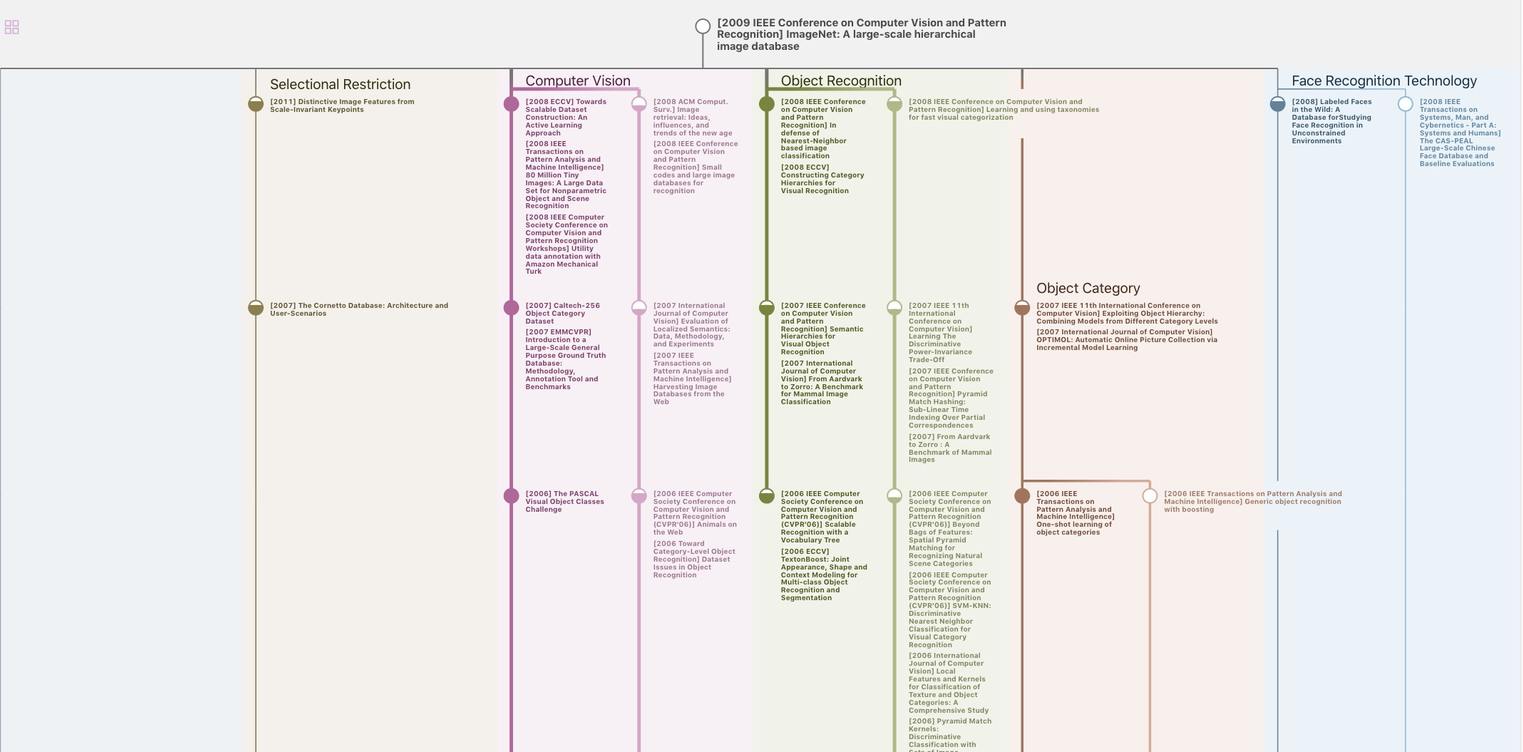
生成溯源树,研究论文发展脉络
Chat Paper
正在生成论文摘要