Image classification of sugarcane aphid density using deep convolutional neural networks
SMART AGRICULTURAL TECHNOLOGY(2023)
摘要
Sugarcane aphid, Melanaphis sacchari (Zehntner), has caused significant yield loss across the sorghum (Sorghum bicolor L. Moench) production region in the U.S. Adequate management of sugarcane aphid depends on pest monitoring and economic threshold levels to spray insecticides. However, scouting this pest under field conditions is time-consuming and inefficient. To assist pest monitoring, we propose the use of deep learning models to automatically classify sugarcane aphid infestation on leaves according to different density levels in images. We used a total of 5,048 images collected during field scouting events and evaluated the performance of four deep learning classification models: Inception v3, DenseNet 121, Resnet 50, and Xception. We trained the models to classify aphid densities into 6 classes based on established standard threshold levels for spraying: no aphids present (0 sugarcane aphids/leaf), no threat or below an action/treatment threshold (1-10, and 11-39 sugarcane aphids/leaf), and infested above an economic threshold where an insecticide should be applied (40-125, 126-500, and > 500 sugarcane aphids/leaf) to manage sugarcane aphid in field conditions. Among these models, Inception v3 and Xception performed best with an overall accuracy score of 86% and a lower number of misclassified images. Importantly, the models correctly classified aphids as above or below threshold spray density over 97% of the time. The methodology developed and the models tested in this study can be used in sampling protocols and further mobile applications or remote sensing technologies. These technologies can assist sorghum growers and researchers to scout and screen sugarcane aphid in susceptible and resistant sorghum varieties automatically and provide accurate recommendations on whether or not to apply pesticides.
更多查看译文
关键词
Sorghum,Integrated pest management,Sugarcane aphid,Automation,Classification
AI 理解论文
溯源树
样例
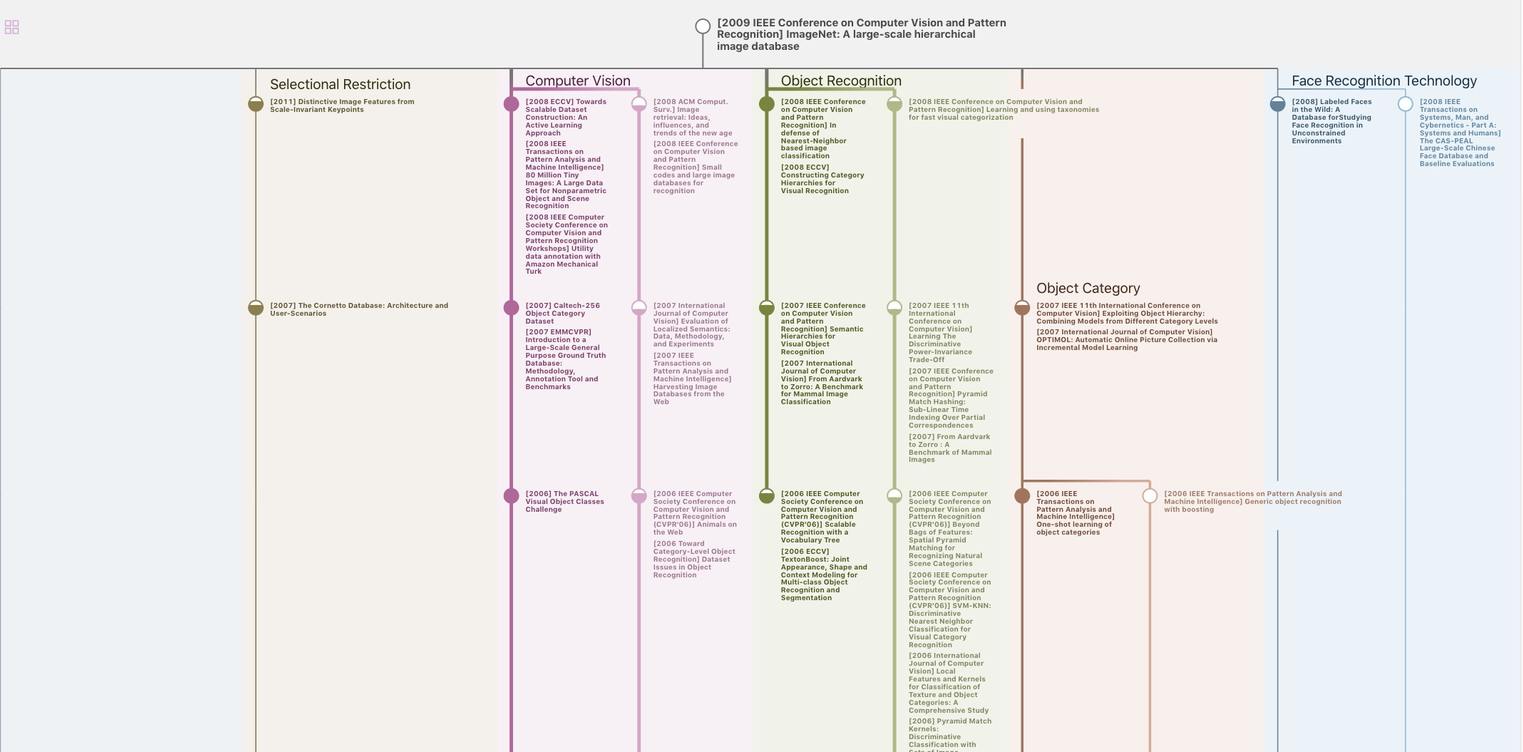
生成溯源树,研究论文发展脉络
Chat Paper
正在生成论文摘要