Learning-based Distributed Control for UAVs to Achieve Fully Connected Effect using Local Information
2022 International Conference on Unmanned Aircraft Systems (ICUAS)(2022)
摘要
Centralized controllers are not scalable because they require high computational cost and communication bandwidth (full connectivity). Furthermore, the entire group will also be vulnerable if the centralized agent is under a cyber attack. So, the need for distributed controllers becomes a necessity. However, finding efficient distributed controllers is challenging. Hence the primary goal of this work is to learn controllers that emulate centralized controllers but only use local information. We use SVM-regression, Gaussian process regression, and neural networks to learn the centralized controllers applied to two problems, a one-dimensional aerial platooning and synchronized convergence of multiple UAVs onto a stationary target. We discuss different learning models, i.e., single and unique learning models. Also, we investigate two types of learning-based control approaches, Full Learning Control (FLC) and Partial Learning Control (PLC). Further, from simulations, we show the effectiveness of the proposed approach to these problems.
更多查看译文
AI 理解论文
溯源树
样例
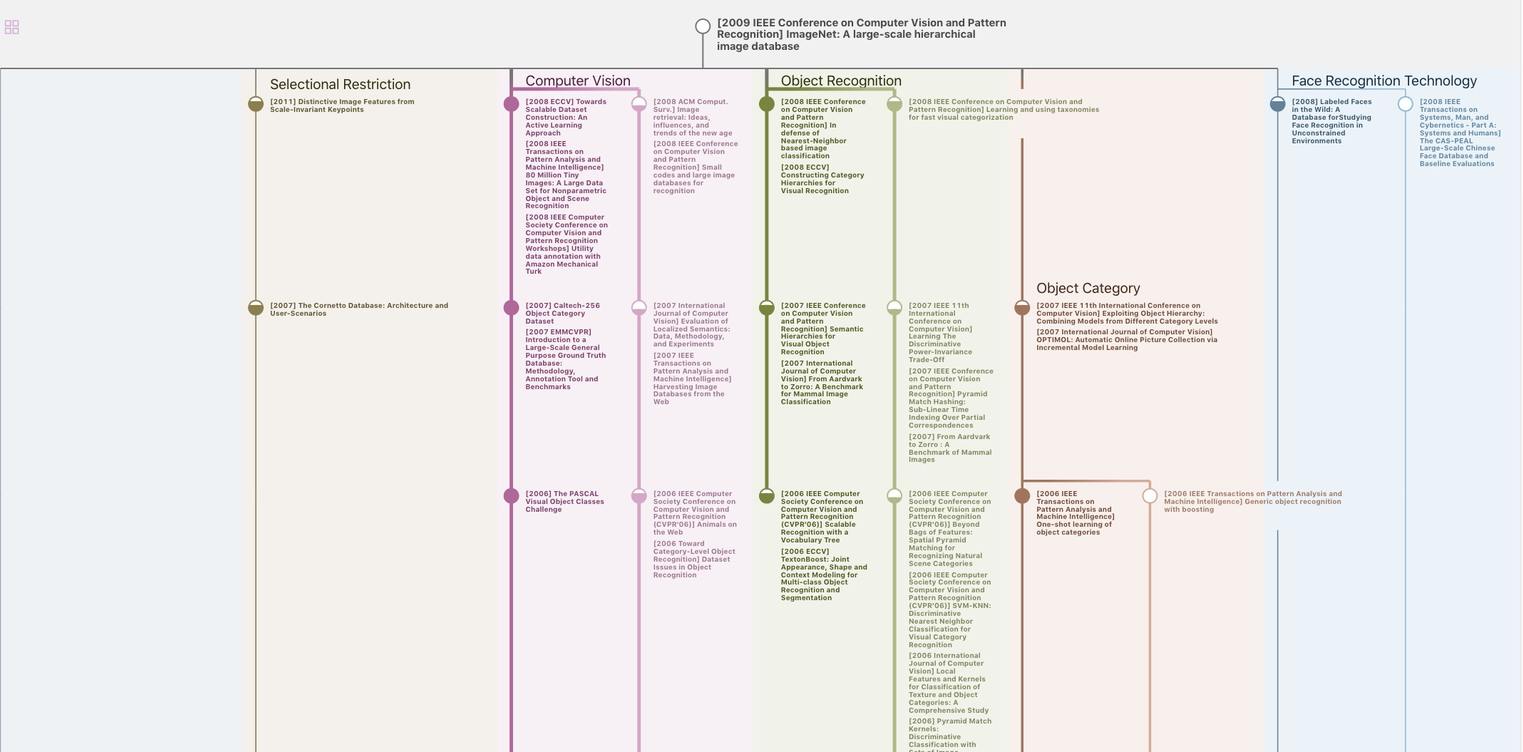
生成溯源树,研究论文发展脉络
Chat Paper
正在生成论文摘要