Predicting Data Scientist Stuckness During the Development of Machine Learning Classifiers
2022 IEEE Symposium on Visual Languages and Human-Centric Computing (VL/HCC)(2022)
摘要
The success of data scientists in developing machine learning models is contingent on an iterative development process for detecting patterns in data, finding and extracting useful features, and maximizing their model’s performance. However, it is often the case that they struggle during model development and become stuck and unable to make significant progress. We collected qualitative and quantitative data from the workflow of data scientists that allow us to learn from and examine such moments of stuckness. We used this data to develop a model for predicting stuckness based on real-time indicators, such as code artifacts, and then used the model to develop an innovative algorithm that determines precisely when a potential stuckness intervention should occur: as close as possible to the beginning of actual stuckness. Our algorithm’s performance indicates the potential efficacy of predicting data scientist stuckness algorithmically under real-world circumstances and for real-world needs.
更多查看译文
AI 理解论文
溯源树
样例
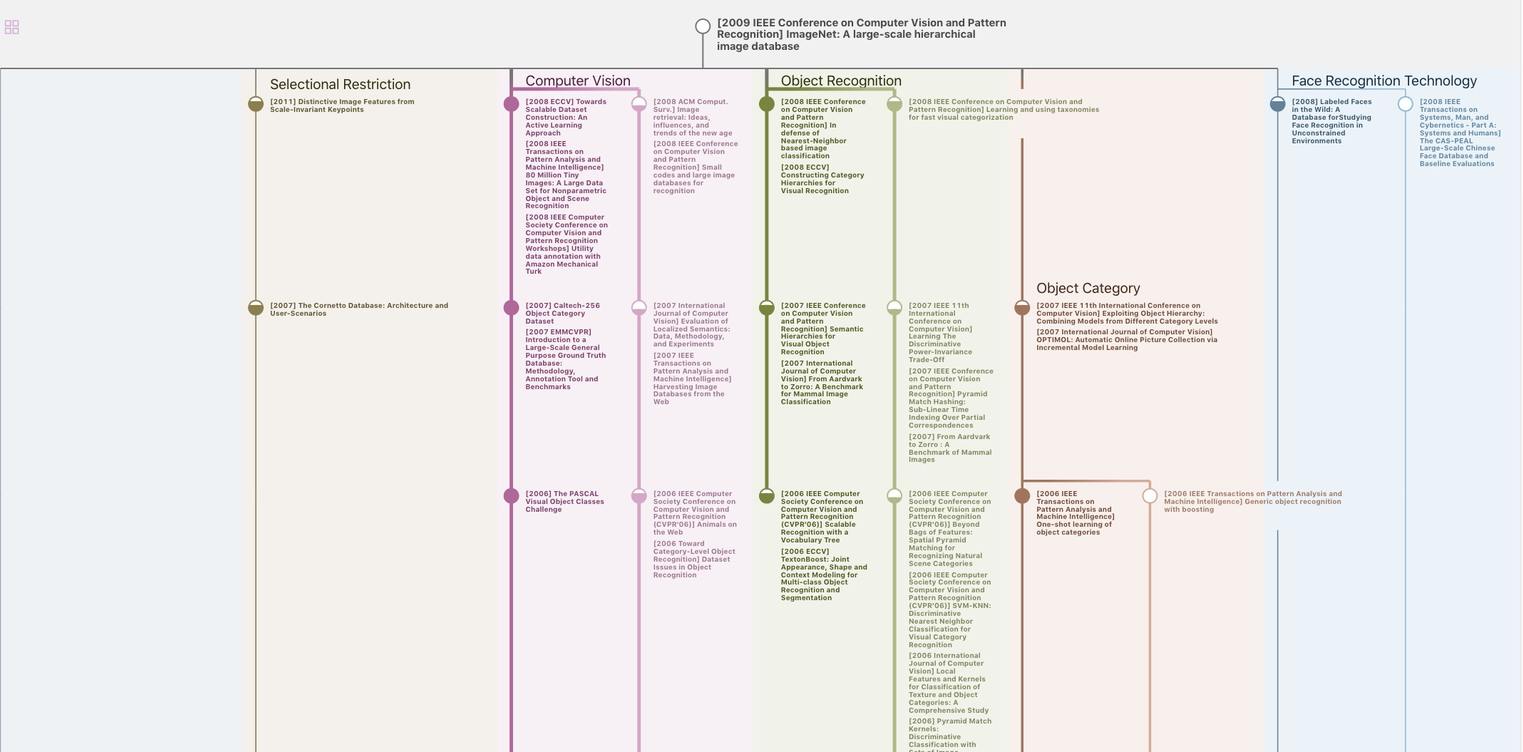
生成溯源树,研究论文发展脉络
Chat Paper
正在生成论文摘要