dGG, dRNG, DSC: New Degree-based Shape-based Faithfulness Metrics for Large and Complex Graph Visualization
2022 IEEE 15th Pacific Visualization Symposium (PacificVis)(2022)
摘要
Shape-based metrics measure how faithfully a drawing D of a large graph G shows the structure of graph, by comparing the similarity between G and a proximity graph S computed from D. Although these metrics can successfully evaluate drawings of large graphs, they are limited to relatively sparse graphs, since existing metrics use planar proximity graphs GG (Gabriel Graph) and RNG (Relative Neighbourhood Graph). This paper presents new shape-based faithfulness metrics for evaluating drawings of large and complex graphs, using high-order prox-imity graphs k-GG and k-RNG. Extensive experiments demonstrate that our new shape-based metrics using degree-based proximity graphs dGG and dRNG can more accurately measure the faithful-ness of drawings of large and complex graphs, with a significant improvement of over 100% better, on average, than the existing shape-based metrics using GG and RNG. Moreover, we present a new shape change faithfulness metric DSC for evaluating drawings of dynamic graphs, by measuring how proportional the geometric shape change in the drawings of dynamic graphs is to the ground truth change in dynamic graphs. Validation using deformation experiments support that DSC can accurately measure shape change faithfulness in dynamic graph drawing. Furthermore, we present extensive comparison experiments of ten popular graph layouts using our new shape-based metrics dGG, dRNG and DSC, to recommend which layouts can give a better shape-faithful graph drawing for large and complex graphs.
更多查看译文
关键词
Human-centered computing,Visualization,Visualization design and evaluation methods
AI 理解论文
溯源树
样例
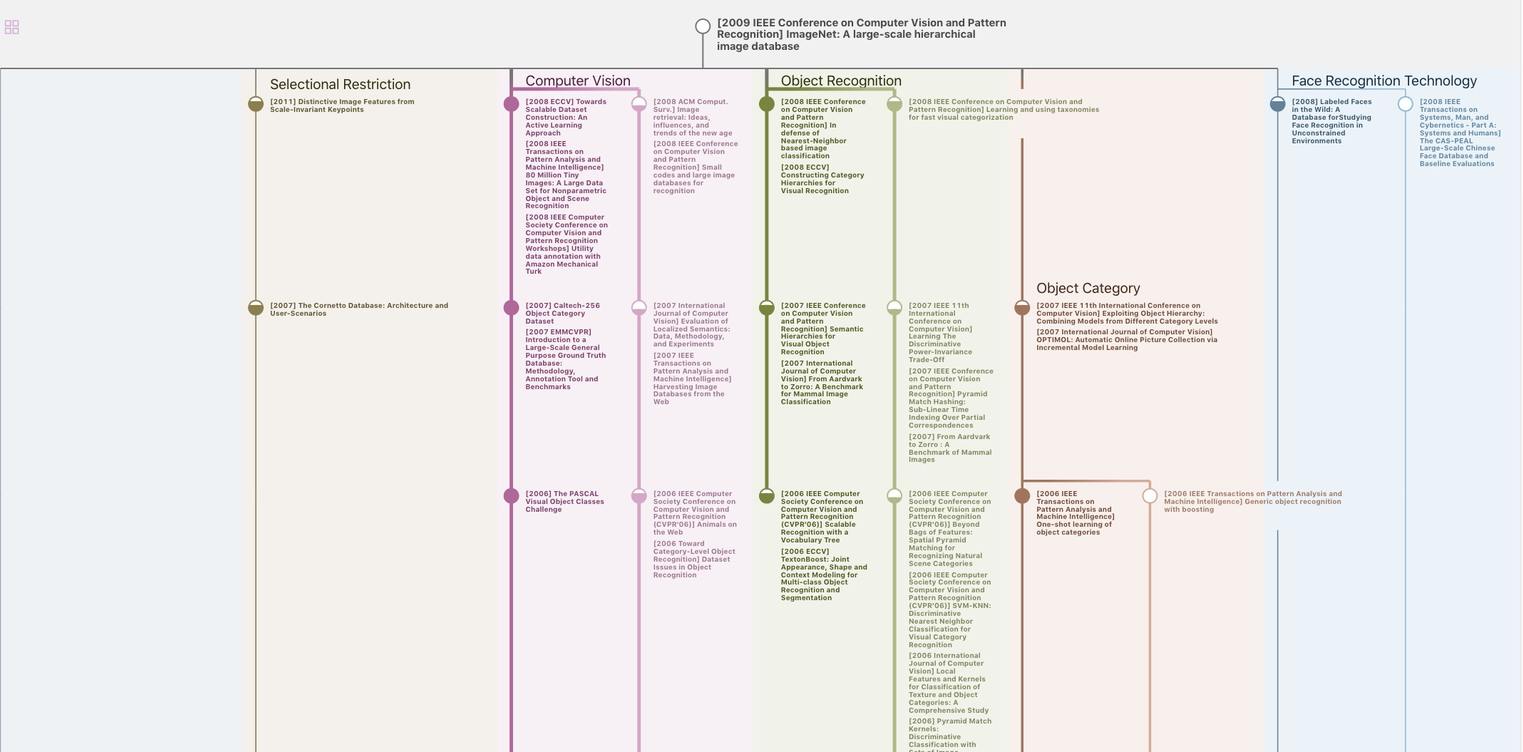
生成溯源树,研究论文发展脉络
Chat Paper
正在生成论文摘要