Background Noise Suppression for DAS-VSP Records Using GC-AB-Unet
IEEE GEOSCIENCE AND REMOTE SENSING LETTERS(2022)
摘要
Distributed acoustic sensing (DAS) is one of the most popular sensors for seismic acquisition. Compared with traditional seismic acquisition technology, DAS has the advantages of full-well coverage, high density, high efficiency, high sensitivity, low cost, strong resistance to high temperature and high pressure, and anti-electromagnetic field interference. However, the DAS vertical seismic profile (VSP) data are contaminated by strong noise interference, which brings challenges for practical applications and difficulties to seismic inversion and interpretation. A deep learning method named U-net with Global Context Block and Attention Block (GC-AB-Unet) is proposed to suppress the background noise and increase the data quality for DAS-VSP records without knowing any prior information. In GC-AB-Unet, several dropout layers are added to the U-net to avoid overfitting. Meanwhile, to speed up the network training, the residual units are set as the output of the network. Furthermore, a global context block (GC-Block) is introduced for better capturing shallow and deep features by extracting global context information. In addition, an Attention Block is used to emphasize seismic event features and restrain irrelevant details in seismic data. We also construct a training dataset by utilizing the synthetic data and real noise of DAS-VSP data. The denoising results for both synthetic data and field DAS-VSP data show that compared to the original U-net and Damped Rank Reduction (DRR) method, the GC-AB-Unet network is able to preserve the effective signals with almost no energy leakage while suppressing a large amount of background noise.
更多查看译文
关键词
Noise reduction,Training,Background noise,Convolution,Sensors,Noise measurement,Time-domain analysis,Distributed acoustic sensing (DAS),noise suppression,U-net,vertical seismic profile (VSP)
AI 理解论文
溯源树
样例
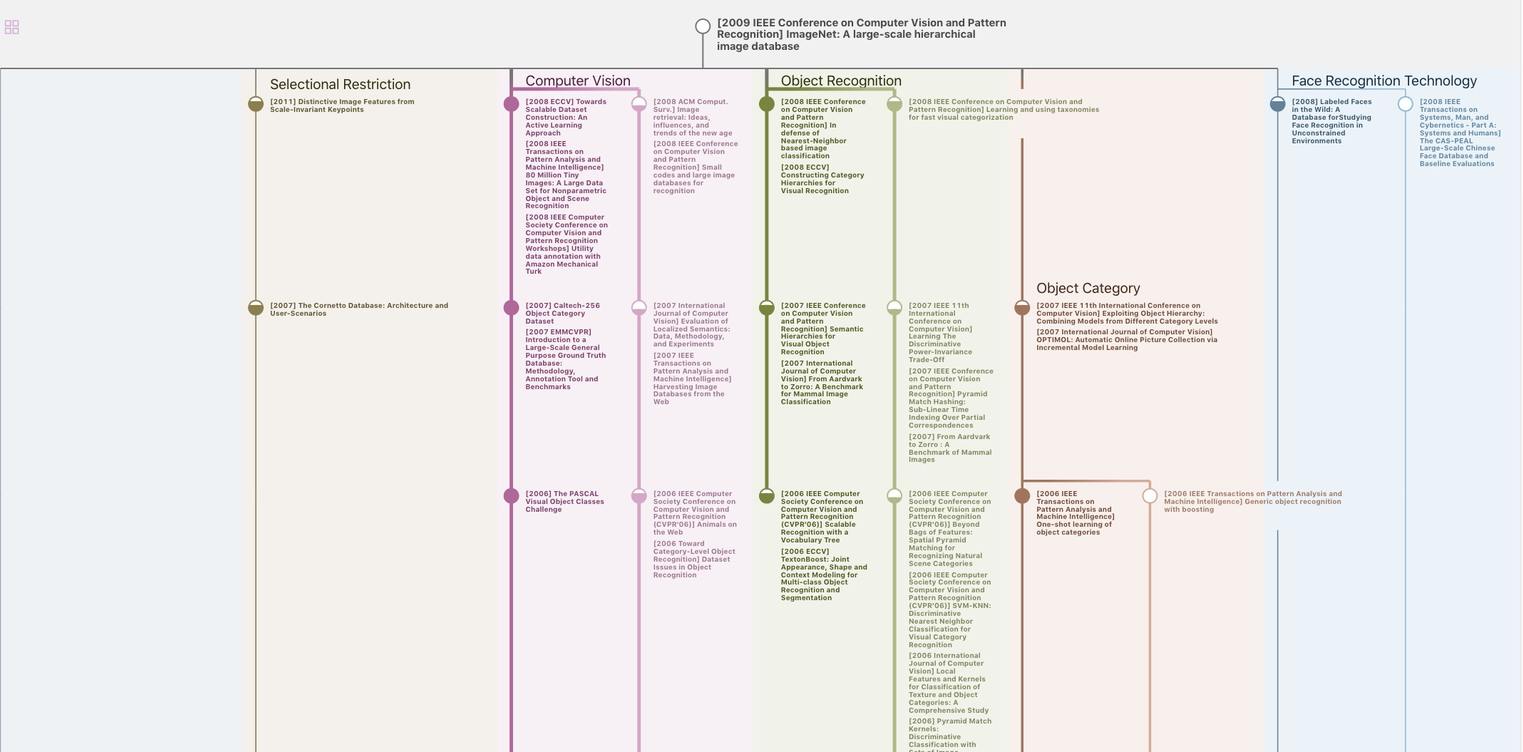
生成溯源树,研究论文发展脉络
Chat Paper
正在生成论文摘要