Multi-scale graph learning for ovarian tumor segmentation from CT images
Neurocomputing(2022)
Abstract
Ovarian cancer is the gynecological malignant tumor with low early diagnosis rate and high mortality. Automated and reliable segmentation of ovarian tumor plays an essential role in ovarian cancer radiotherapy, which assists radiologists to assess cancer progresses and make fast therapeutic schedules. However, ovarian cancer is characterized by polycentric tumors and the tumors are irregular in size, which makes the tumor segmentation task challenging. In this paper, a multi-scale graph learning U-Net (MGLU-Net) is proposed for ovarian tumor segmentation. The network adopts an encoder-decoder architecture as the basic backbone. The feature maps of different scales generated by the encoder are projected into graphs with different sizes. On this basis, the graph learning module is designed to jointly exploit local information and long-range dependencies among ovarian tumors at different locations. Meanwhile, multiple graphs with different sizes are constructed to handle the size variation problem. A channel attention module is further designed to highlight the informative features from the channel domain. Extensive experiments are conducted on a real ovarian CT image dataset and Sliver07 challenge dataset. Experiments demonstrate that the proposed method shows superior performance than the state-of-the-art methods.
MoreTranslated text
Key words
Graph convolutional network,Ovarian cancer,Tumor segmentation,CT images
AI Read Science
Must-Reading Tree
Example
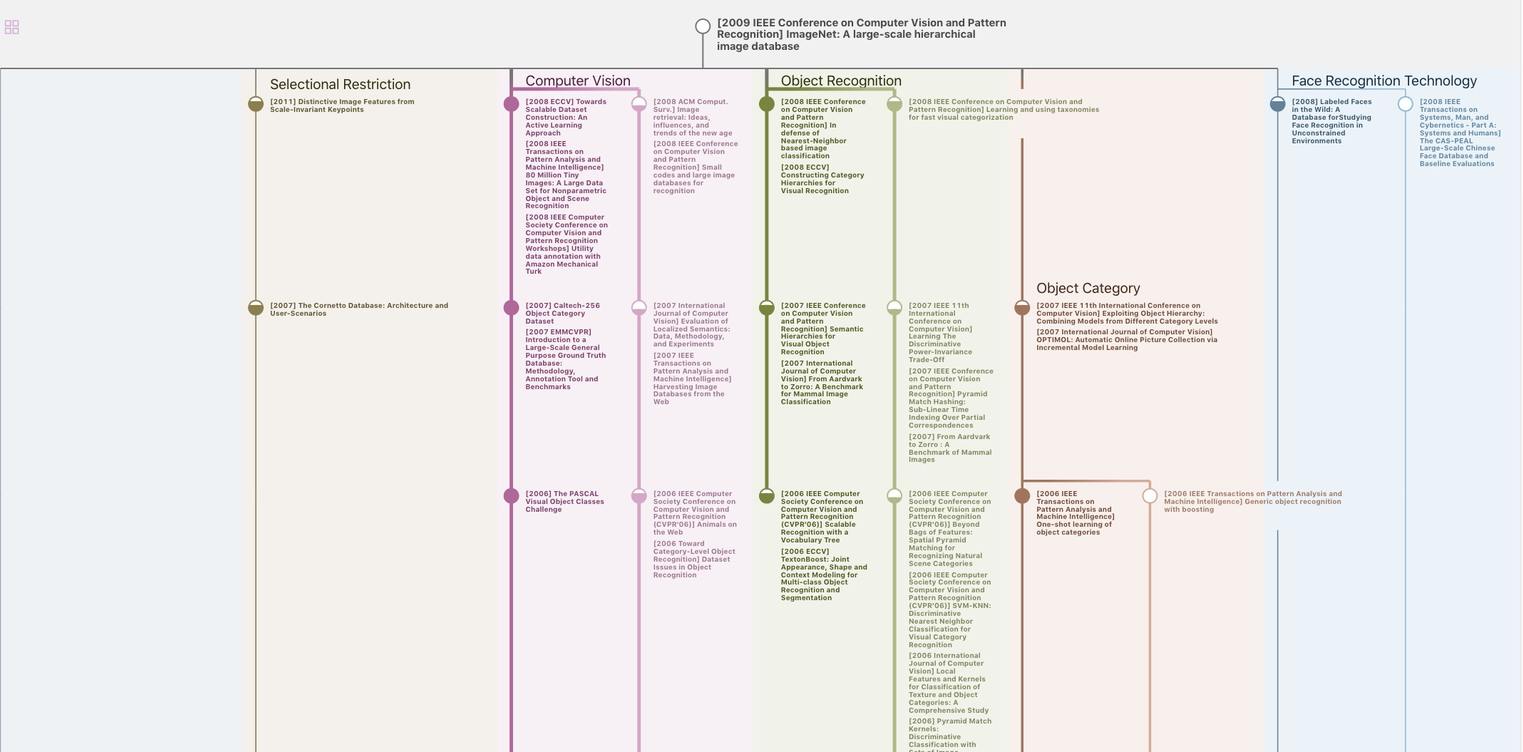
Generate MRT to find the research sequence of this paper
Chat Paper
Summary is being generated by the instructions you defined