Forestry Crane Automation using Learning-based Visual Grasping Point Prediction
2022 IEEE Sensors Applications Symposium (SAS)(2022)
摘要
This paper presents an approach to automate the log-grasping of a forestry crane. A common hydraulic actuated log-crane is converted into a robotic device by retrofitting it with various sensors yielding perception of internal and environmental states. The approach uses a learning-based visual grasp detection. Once a suitable grasping candidate is determined, the crane starts its kinematic controlled operation. The system’s design process is based on a real-sim-real transfer to avoid possibly harmful, to humans and itself, crane behavior. Firstly, the grasping position prediction network is trained with real-world images. Secondly, an accurate simulation model of the crane, including photo-realistic synthetic images, is established. Note that in simulation, the prediction network trained on real-world data can be used without re-training. The simulation is used to design and verify the crane’s control- and the path planning scheme. In this stage, potentially dangerous maneuvers or insufficient quality of sensory information become visible. Thirdly, the elaborated closed-loop system configuration is transferred to the real-world forestry crane. The pick and place capabilities are verified in simulation as well as experimentally. A comparison shows that simulation and real-world scenarios perform equally well, validating the proposed real-sim-real design procedure.
1
更多查看译文
关键词
learning-based visual grasping point prediction,log-grasping,common hydraulic actuated log-crane,robotic device,sensors yielding perception,internal states,environmental states,suitable grasping candidate,kinematic controlled operation,real-sim-real transfer,crane behavior,grasping position prediction network,real-world images,accurate simulation model,photo-realistic synthetic images,real-world data,closed-loop system configuration,real-world forestry crane,real-sim-real design procedure,forestry crane automation
AI 理解论文
溯源树
样例
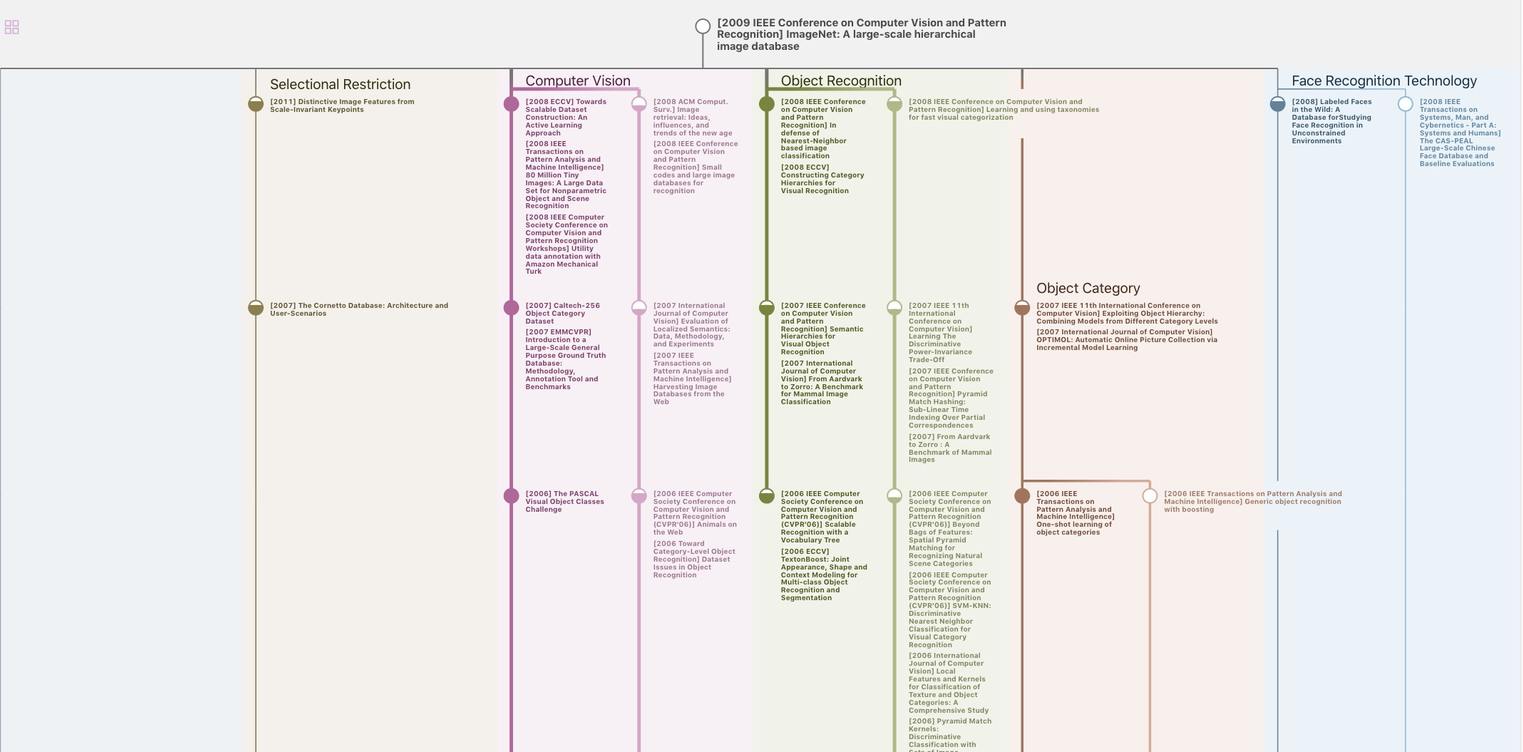
生成溯源树,研究论文发展脉络
Chat Paper
正在生成论文摘要