SQuARe: A Large-Scale Dataset of Sensitive Questions and Acceptable Responses Created Through Human-Machine Collaboration
PROCEEDINGS OF THE 61ST ANNUAL MEETING OF THE ASSOCIATION FOR COMPUTATIONAL LINGUISTICS, ACL 2023, VOL 1(2023)
摘要
The potential social harms that large language models pose, such as generating offensive content and reinforcing biases, are steeply rising. Existing works focus on coping with this concern while interacting with ill-intentioned users, such as those who explicitly make hate speech or elicit harmful responses. However, discussions on sensitive issues can become toxic even if the users are well-intentioned. For safer models in such scenarios, we present the Sensitive Questions and Acceptable Response (SQuARe) dataset, a large-scale Korean dataset of 49k sensitive questions with 42k acceptable and 46k non-acceptable responses. The dataset was constructed leveraging HyperCLOVA in a human-in-the-loop manner based on real news headlines. Experiments show that acceptable response generation significantly improves for HyperCLOVA and GPT-3, demonstrating the efficacy of this dataset.
更多查看译文
关键词
sensitive questions,responses,dataset,large-scale large-scale,human-machine
AI 理解论文
溯源树
样例
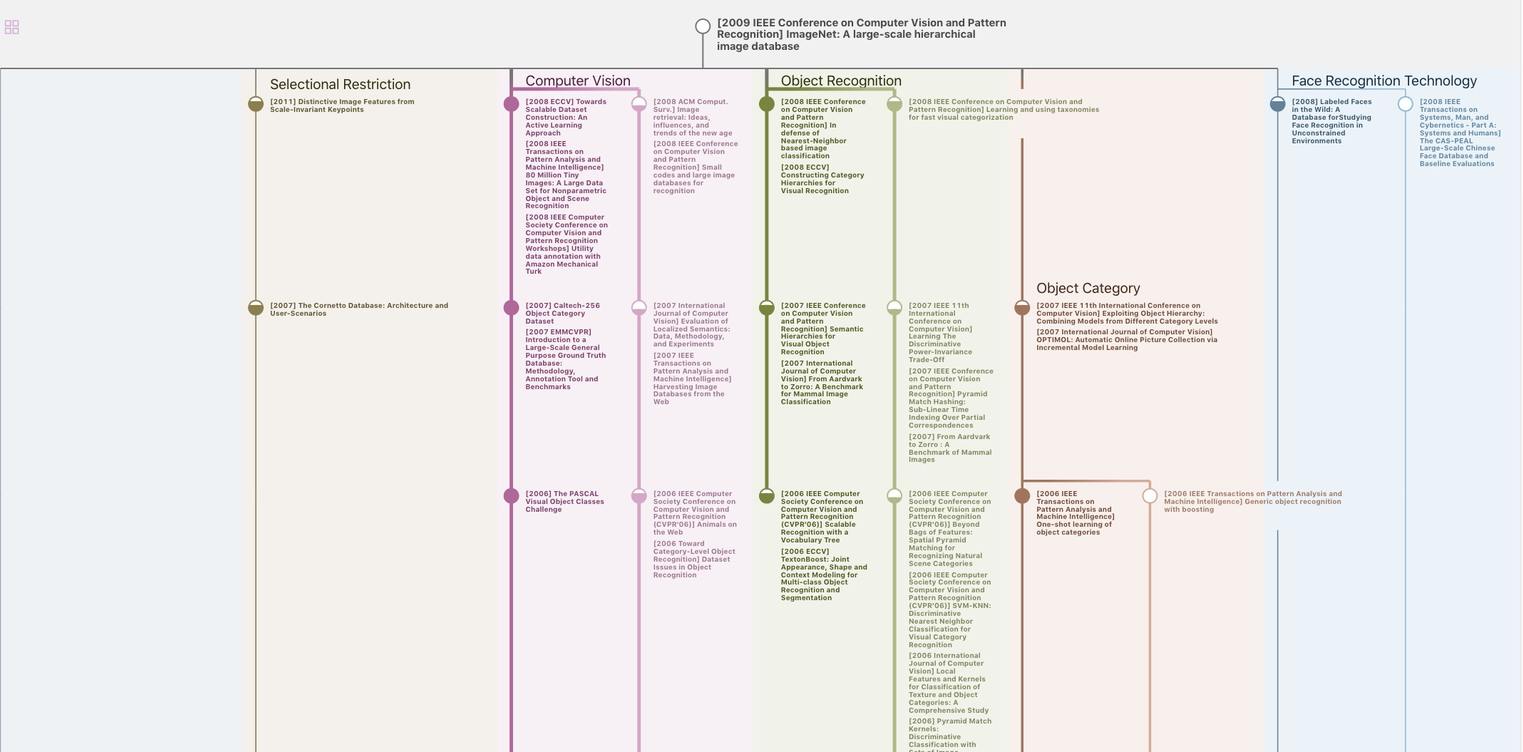
生成溯源树,研究论文发展脉络
Chat Paper
正在生成论文摘要