Rolling horizon policies for multi-stage stochastic assemble-to-order problems
INTERNATIONAL JOURNAL OF PRODUCTION RESEARCH(2023)
摘要
Assemble-to-order approaches deal with randomness in demand for end items by producing components under uncertainty, but assembling them only after demand is observed. Such planning problems can be tackled by stochastic programming, but true multistage models are computationally challenging and only a few studies apply them to production planning. Solutions based on two-stage models are often short-sighted and unable to effectively deal with non-stationary demand. A further complication may be the scarcity of available data, especially in the case of correlated and seasonal demand. In this paper, we compare different scenario tree structures. In particular, we enrich a two-stage formulation by introducing a piecewise linear approximation of the value of the terminal inventory, to mitigate the two-stage myopic behaviour. We compare the out-of-sample performance of the resulting models by rolling horizon simulations, within a data-driven setting, characterised by seasonality, bimodality, and correlations in the distribution of end item demand. Computational experiments suggest the potential benefit of adding a terminal value function and illustrate interesting patterns arising from demand correlations and the level of available capacity. The proposed approach can provide support to typical MRP/ERP systems, when a two-level approach is pursued, based on master production and final assembly scheduling.
更多查看译文
关键词
Stochastic programming,end-of-horizon effect,scenario tree generation,assemble-to-order production,data-driven optimisation
AI 理解论文
溯源树
样例
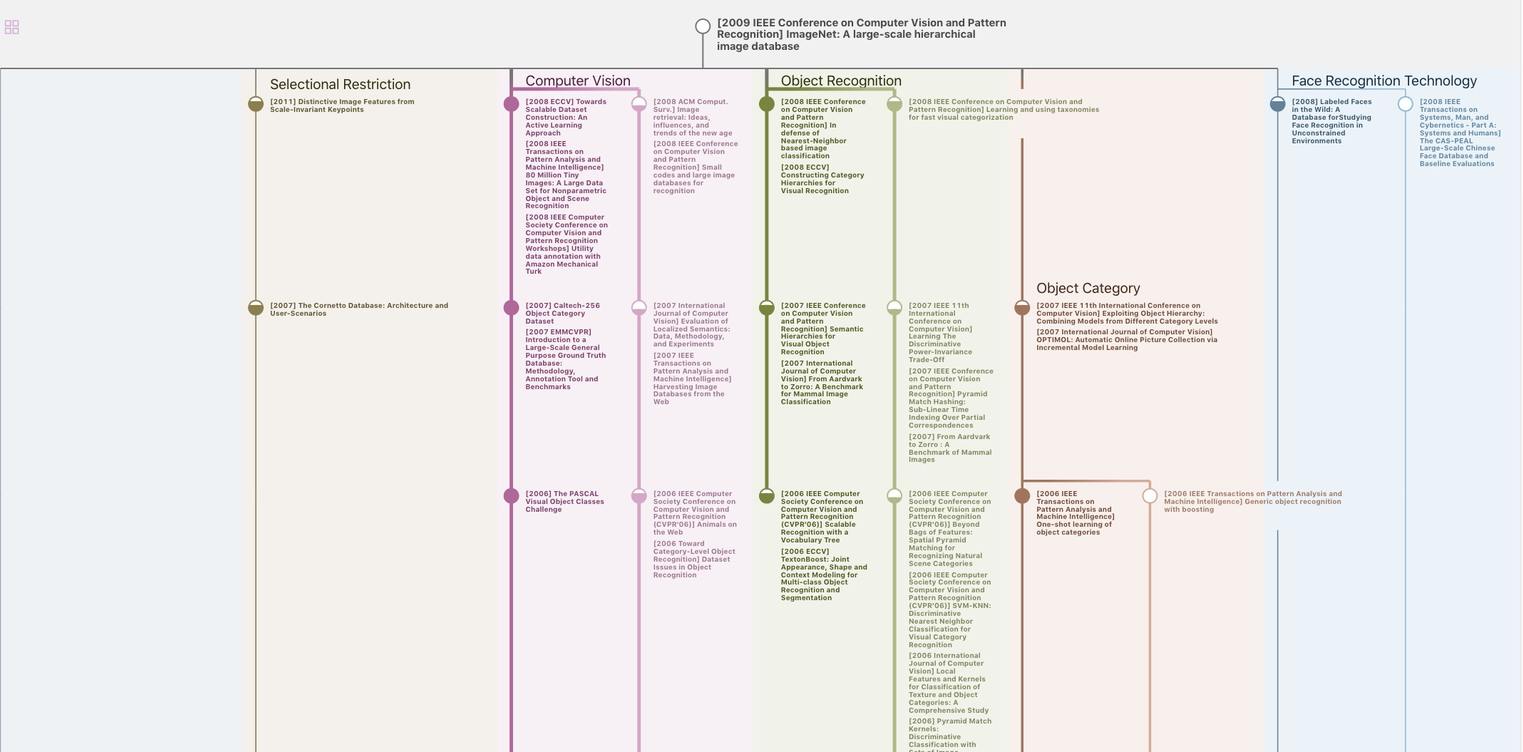
生成溯源树,研究论文发展脉络
Chat Paper
正在生成论文摘要