A Object Detection Algorithm Incorporates Lightweight Network and Dual Attention Mechanism
2022 International Conference on Computer Engineering and Artificial Intelligence (ICCEAI)(2022)
摘要
To solve the problems of the existing object detection algorithms, such as insufficient feature extraction ability and slow detection speed, we propose a object detection algorithm which incorporates lightweight network and dual attention mechanism. Firstly, the YOLOv3 object detection network was used as the basic detection network and EfficientNet was used to replace backbone network to reduce the number of parameters and computation. Secondly, the CBAM attention mechanism was added to improve the sensitivity of the model to object features, suppress interference information and improve the object recognition accuracy. Finally, the Soft-NMS is introduced to replace the traditional NMS, which not only improves the accuracy of localization but also reduces the missing rate of the model. Experimental results show that compared with the original model, the weight file of the object detection model is reduced by 74.22%, the detection speed is improved by 16.93%, and the mAP on the object data set reaches 93.27%, which is improved by 2.85% compared with the original YOLOv3 algorithm. The detection ability of the algorithm in this paper is significantly improved, which well promotes the requirements of real-time detection of complex scenes on mobile terminals.
更多查看译文
关键词
Object detection,Lightweight,EfficientNet,CBAM
AI 理解论文
溯源树
样例
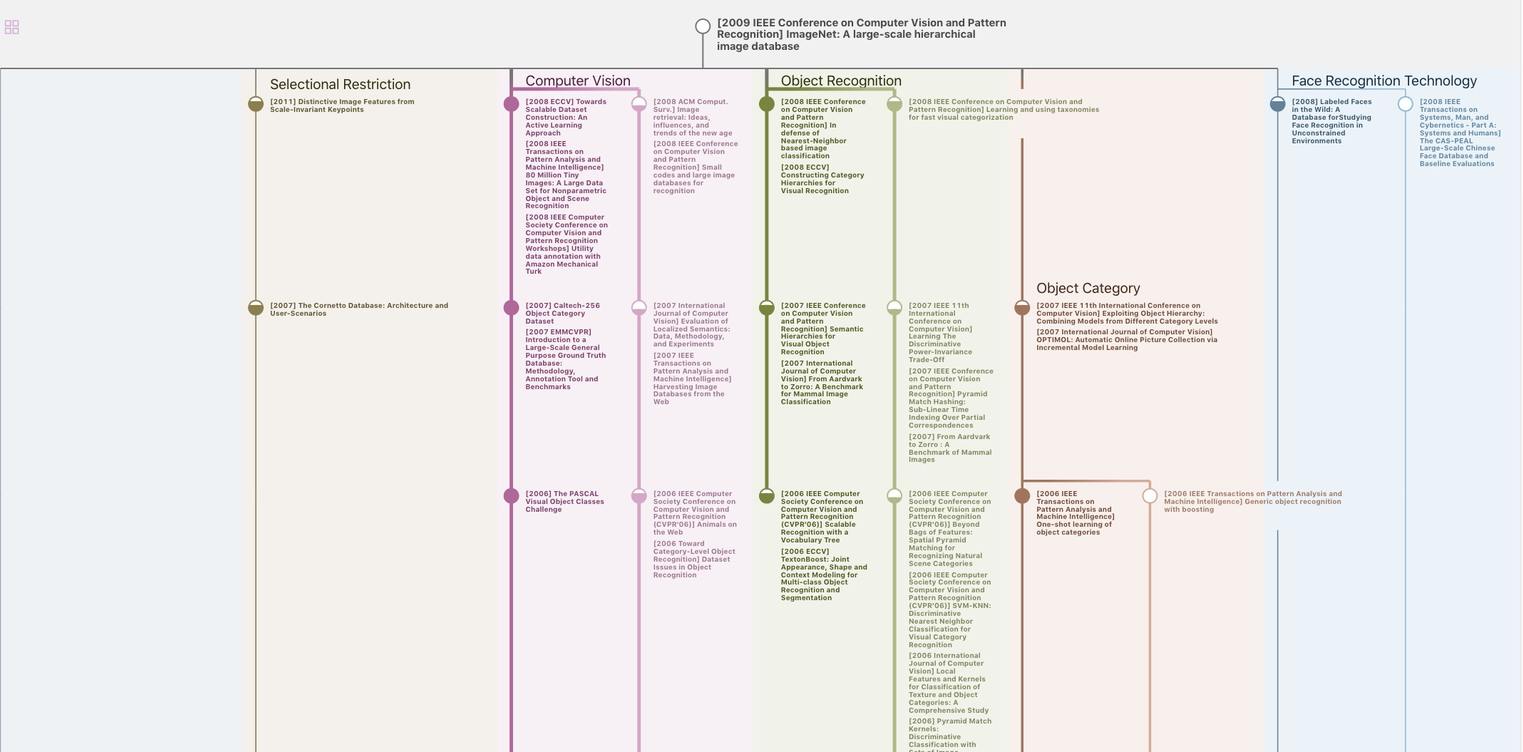
生成溯源树,研究论文发展脉络
Chat Paper
正在生成论文摘要