Definition of immune molecular subtypes with distinct immune microenvironment, recurrence, and PANoptosis features to aid clinical therapeutic decision-making.
Frontiers in genetics(2022)
Abstract
Cervical cancer poses a remarkable health burden to females globally. Despite major advances in early detection and treatment modalities, some patients still relapse. The present study proposed a novel immune molecular classification that reflected distinct recurrent risk and therapeutic responses in cervical cancer. We retrospectively collected two cervical cancer cohorts: TCGA and GSE44001. Consensus clustering approach was conducted based on expression profiling of recurrence- and immune-related genes. The abundance of immune cells was inferred five algorithms. Immune functions and signatures were quantified through ssGSEA. Genetic mutations were analyzed by maftools package. Immunotherapeutic response was inferred tumor mutation burden (TMB), Tumor Immune Dysfunction and Exclusion (TIDE), and Submap methods. Finally, we developed a LASSO model for recurrence prediction. Cervical cancer samples were categorized into two immune subtypes (IC1, and IC2). IC2 exhibited better disease free survival (DFS), increased immune cell infiltration within the immune microenvironment, higher expression of immune checkpoints, higher activity of immune-relevant pathways (APC co-inhibition and co-stimulation, inflammation-promoting, MHC class I, IFN response, leukocyte and stromal fractions, macrophage regulation, and TCR Shannon), and higher frequencies of genetic mutations. This molecular classification exhibited a remarkable difference with existing immune subtypes, with diverse PANoptosis (pyroptosis, apoptosis and necroptosis) features. Patients in IC2 were more likely to respond to immunotherapy and targeted, and chemotherapeutic agents. The immune subtype-relevant signature was quantified to predict patients' recurrence risk. Altogether, we developed an immune molecular classification, which can be utilized in clinical practice to aid decision-making on recurrence management.
MoreTranslated text
Key words
PANoptosis,cervical cancer,immune microenvironment,immune subtypes,recurrence,therapeutic response
AI Read Science
Must-Reading Tree
Example
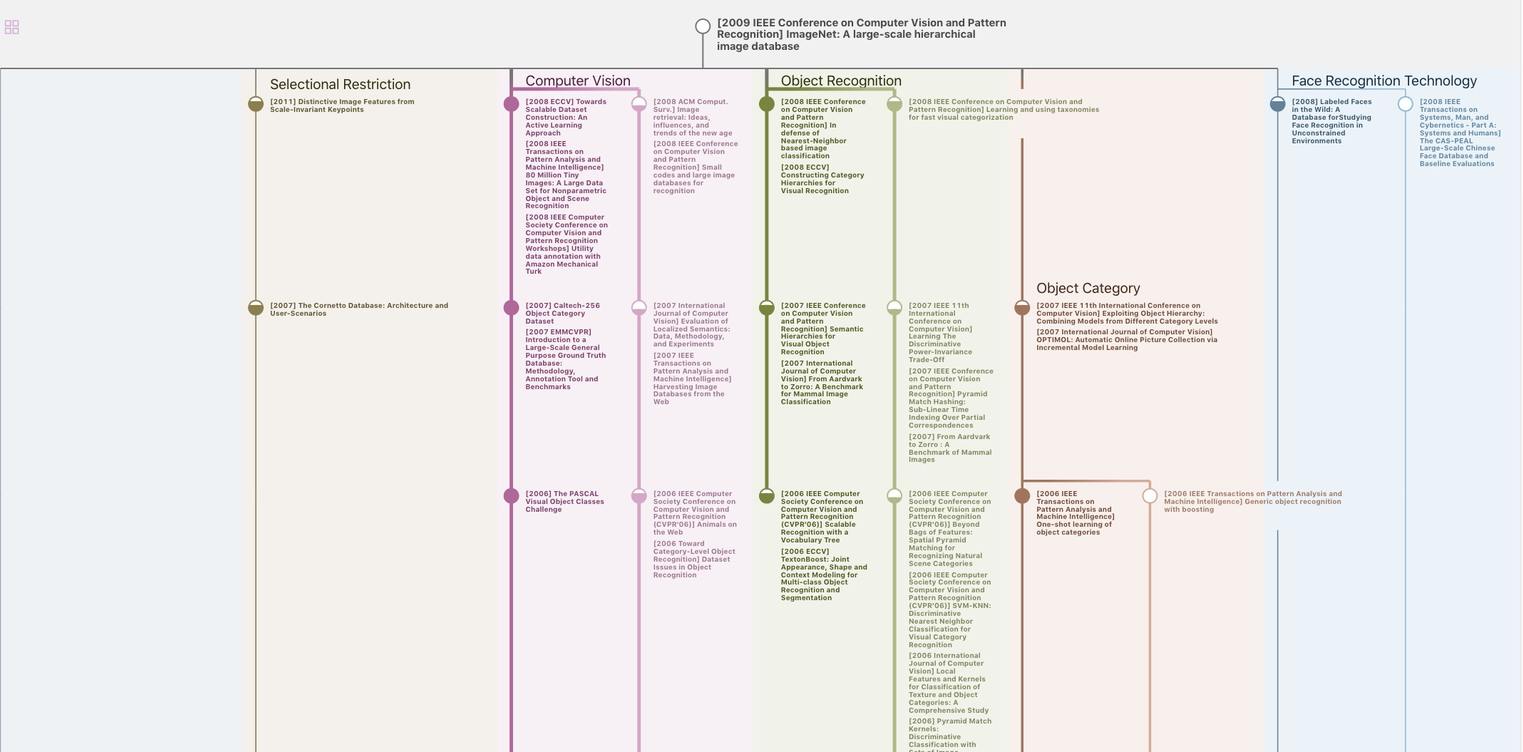
Generate MRT to find the research sequence of this paper
Chat Paper
Summary is being generated by the instructions you defined