Bi-directional gated recurrent unit recurrent neural networks for failure prognosis of proton exchange membrane fuel cells
International Journal of Hydrogen Energy(2022)
Abstract
With zero pollution, low noise and high power density, proton exchange membrane fuel cells (PEMFC) are widely used in automobiles, ships, mobile power and other applications. However, the lifetime of the PEMFC is constrained by degradation. In this paper, a bi-directional gated recursive unit (Bi-GRU) recurrent neural network is proposed for power reactor failure prediction in both dynamic and static cases. In performing degradation prediction, the hyperparameters of the model are adjusted after the selection of variables to obtain the combination of hyperparameters that gives the best results. The proposed model was compared with gated recursive units (GRU), superimposed gated recursive units (St-GRU) and existing studies for better performance. It has better performance compared to other models when one model is used to predict both dynamic and static datasets. And it's very suitable for fault prediction with very high accuracy and the relative error in remaining life prediction is about 0.029%–0.12%.
MoreTranslated text
Key words
PEMFC,Bi-GRU,Degradation,Life prediction,Hyperparameters
AI Read Science
Must-Reading Tree
Example
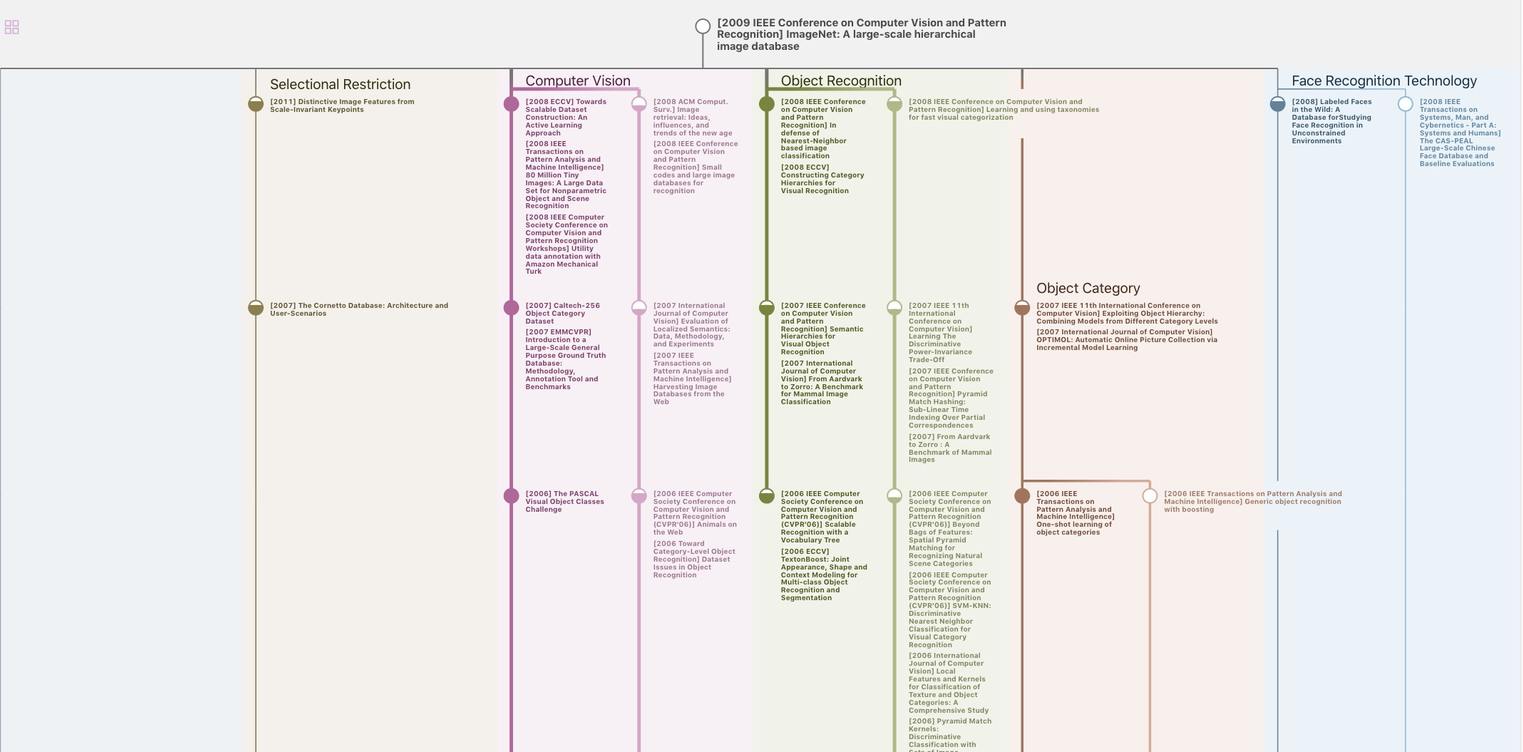
Generate MRT to find the research sequence of this paper
Chat Paper
Summary is being generated by the instructions you defined