An intelligent feature selection approach with systolic tree structures for efficient association rules in big data environment
Computers and Electrical Engineering(2022)
摘要
•The Kernel Possibilistic Fuzzy Local Information C-Means (KPFLICM) clustering and K-means clustering-based feature selection algorithm for association rules (KCFSAR) is used where FPMs are based on Systolic Tree Structures (STS).•Chaotic Butterfly Optimization Method (CBOA) is implemented to attain the optimal minimum support value. The proposed systolic tree structure frequent pattern growth (STSFPG) approach is deployed to mine association rules and evaluates the data attributes using large, tiny file processing.•The proposed work has been easily applied to larger dataset because of Hadoop technology and gives better results for FPG mining due to optimal threshold via CBOA algorithm.•The proposed algorithm has been experimented via the Retail, Kosarak, and Adult has been collected from UCI machine learning repository.•The proposed system's outputs are compared in terms of rule efficiency, running time, accuracy, and memory consumption to other association algorithms that are found satisfactory.•The results of these metrics are measured by varying support count from 0% to 100%.•From the results it concludes that the proposed algorithm gives highest accuracy of 98.14%, and needs lesser running time of 58 s for Adult dataset.
更多查看译文
关键词
Big data,Systolic tree structure,Frequent pattern mining,Frequent pattern growth,Association rule mining,Kernel possibilistic fuzzy local information C-means clustering,Chaotic butterfly optimization algorithm
AI 理解论文
溯源树
样例
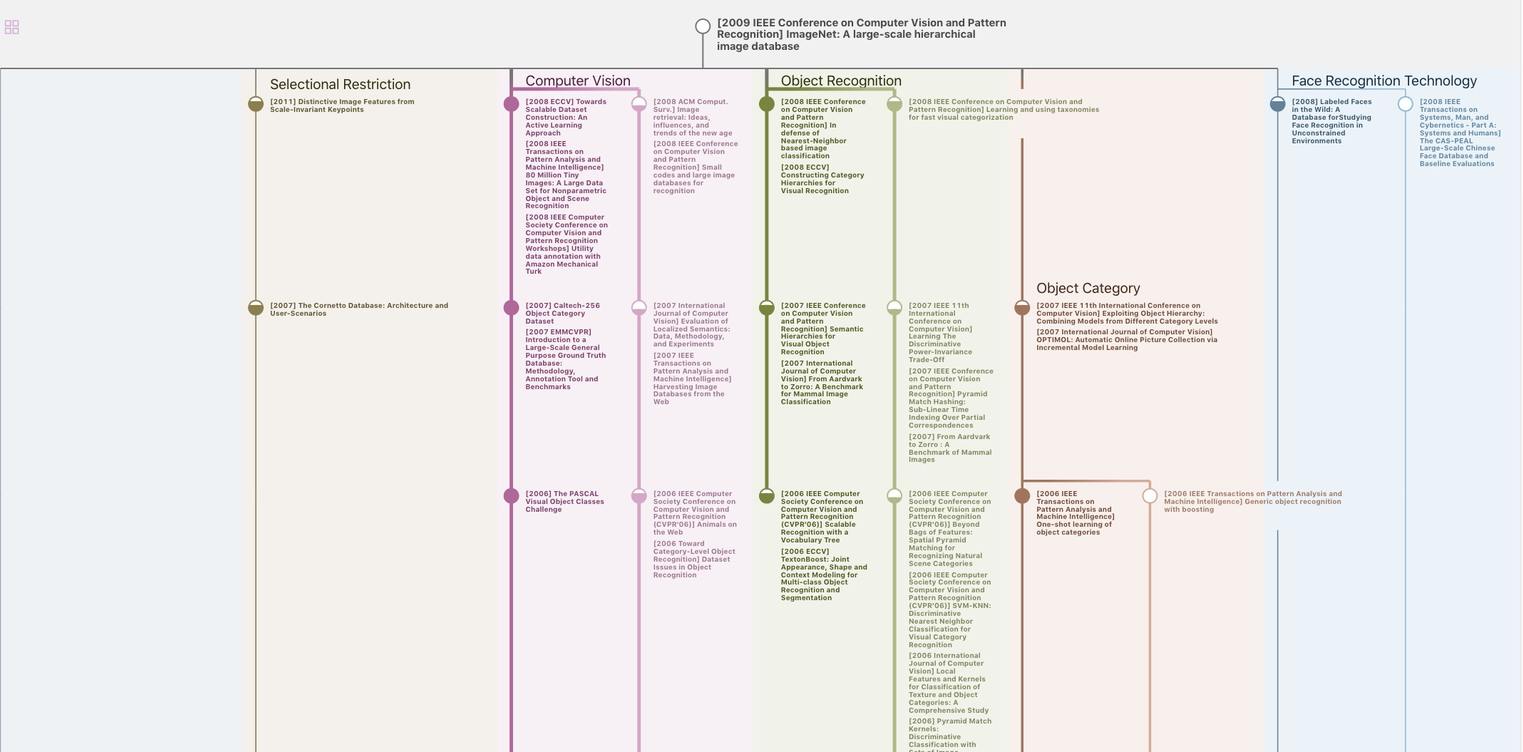
生成溯源树,研究论文发展脉络
Chat Paper
正在生成论文摘要