TPSN: Transformer-based multi-Prototype Search Network for few-shot semantic segmentation
Computers and Electrical Engineering(2022)
Abstract
Few-shot semantic segmentation aims to segment new objects in the image with limited annotations. Typically, in metric-based few-shot learning, the expression of categories is obtained by averaging global support object information. However, a single prototype cannot accurately describe a category. Meanwhile, simple foreground averaging operations also ignore the dependencies between objects and their surroundings. In this paper, we propose a novel Transformer-based Prototype Search Network (TPSN) for few-shot segmentation. We use the transformer encoder to integrate information between different image regions and then use the decoder to express a category in terms of multiple prototypes. The multi-prototype approach can effectively alleviate the feature fluctuation caused by limited annotation data. Moreover, we use adaptive prototype search during multi-prototype extraction instead of the ordinary averaging operation compared with the previous few-shot prototype framework. This helps the network integrate the different image regions’ information and fuse object features with their dependent background information, obtaining more reasonable prototype expressions. In addition, to encourage the category’s prototypes to focus on different parts and maintain consistency in high-level semantics, we use the diversity and consistency loss to constrain the multi-prototype training. Experiments show that our algorithm achieves state-of-the-art performance in few-shot segmentation on two datasets: PASCAL-5i and COCO-20i.
MoreTranslated text
Key words
Semantic segmentation,Few-shot learning,Vision transformer,Multiple prototypes
AI Read Science
Must-Reading Tree
Example
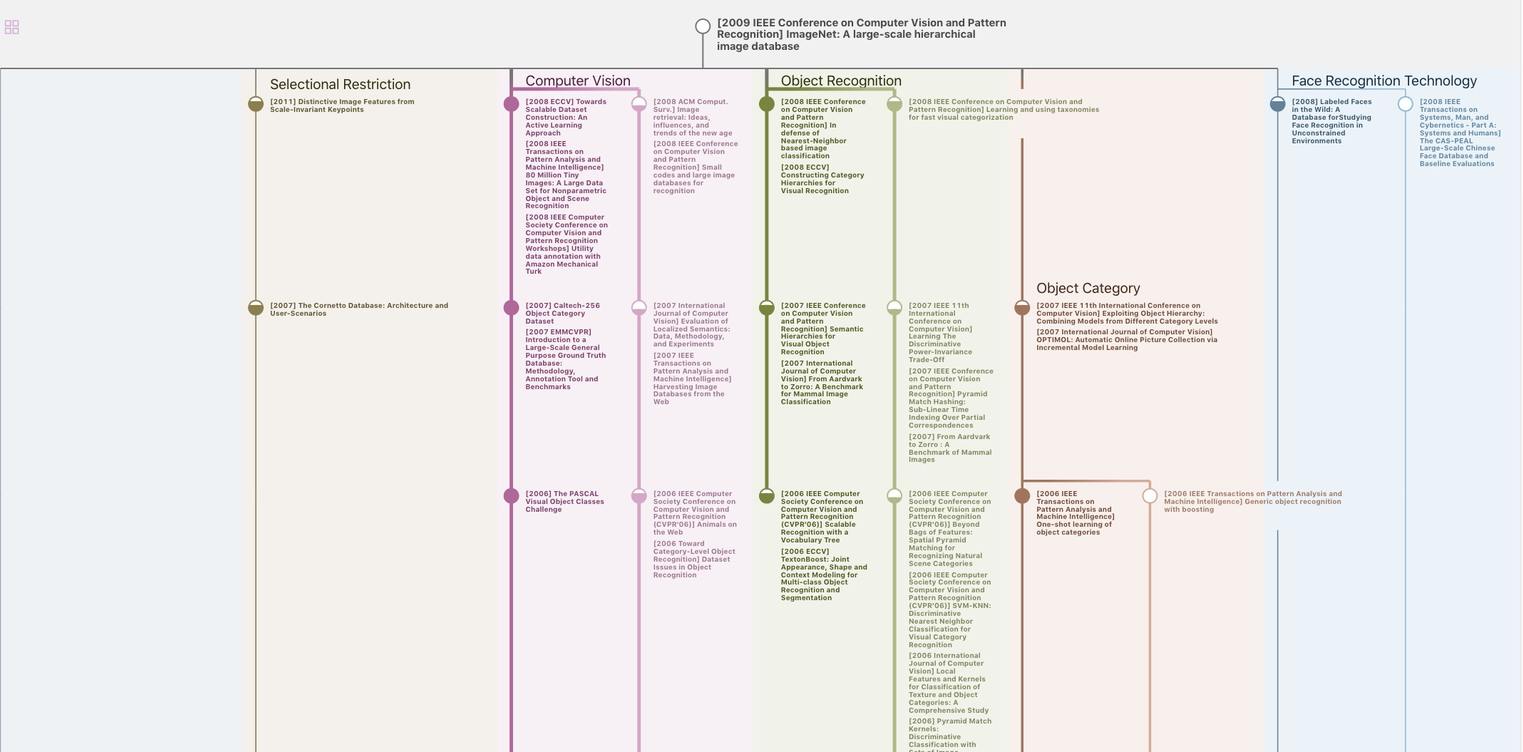
Generate MRT to find the research sequence of this paper
Chat Paper
Summary is being generated by the instructions you defined