Benchmarks of Cuda-Based GMRES Solver for Toeplitz and Hankel Matrices and Applications to Topology Optimization of Photonic Components
Computational Mathematics and Modeling(2022)
摘要
Generalized Minimal Residual Method (GMRES) was benchmarked on many types of GPUs for solving linear systems based on dense and sparse matrices. However, there are still no GMRES implementation benchmarks on Tesla V100 compared to GTX 1080 Ti ones or even for Toeplitz-like matrices. The introduced software consists of a Python module and a C++ library which enable to manage streams for concurrent computations of separated linear systems on a GPU (and GPUs). The GMRES solver is parallelized for running on a NVIDIA GPGPU accelerator. The parallelization efficiency is explored when GMRES is applied to solve (Helmholtz equation) linear systems based on the use of Green’s Function Integral Equation Method (GFIEM) for computing electric field distribution in the design domain. The proposed implementation shew the maximal speedup of 55 ( t=0.017 s ) and of 125 ( t=0.77 s ) for 1024 × 1024 (on GTX 1080 Ti) and 8192 × 8192 (on Tesla V100) dense Toeplitz matrices generated from GFIEM. 1024 × 1024 resolution provides accuracy 6.1
更多查看译文
关键词
GPU,performance,CUDA C++,parallelization,GMRES,Toeplitz-like matrix,Python module,Helmholtz equation,topology optimization
AI 理解论文
溯源树
样例
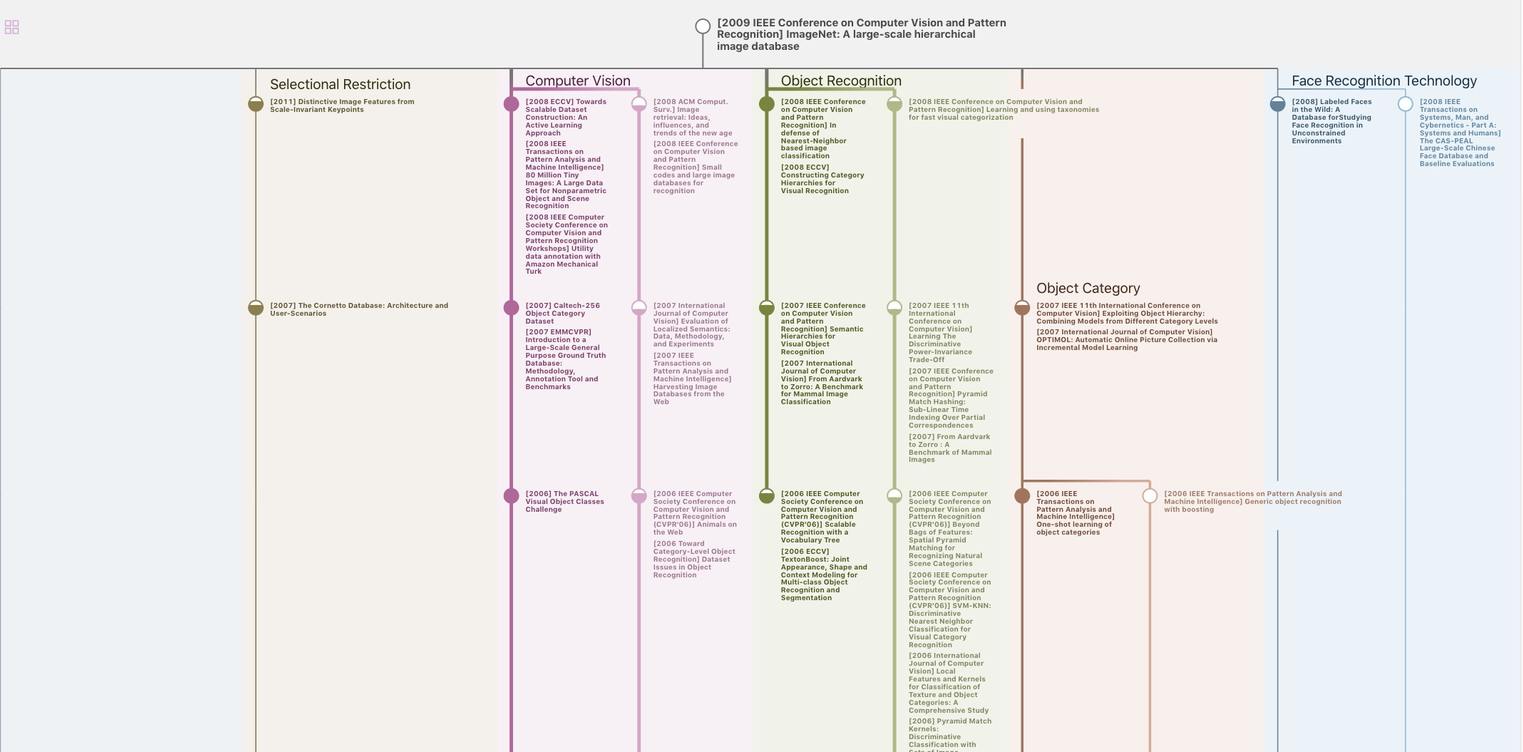
生成溯源树,研究论文发展脉络
Chat Paper
正在生成论文摘要