Assessment of deep learning methods for classification of cereal crop growth stage pre and post canopy closure
SSRN Electronic Journal(2023)
Abstract
Growth stage (GS) is an important crop growth metric commonly used in commercial farms. We focus on wheat and barley GS classification based on in-field proximal images using convolutional neural networks (ConvNets). For comparison purposes, use of a conventional machine learning algorithm was also investigated. The research includes extensive data collection of images of wheat and barley crops over a 3-year period. During data collection, videos were recorded during field walks at two camera views: downward looking and 45 deg angled. The resulting dataset contains 110,000 images of wheat and 106,000 of barley taken over 34 and 33 GS classes, respectively. Three methods were investigated as candidate technologies for the problem of GS classification. These methods were: (I) feature extraction and support vector machine, (II) ConvNet with learning from scratch, and (III) ConvNet with transfer learning. The methods were assessed for classification accuracy using test images taken (a) in fields on days imagined in the training data (i.e., seen field-days GS classification) and (b) in fields on days not imagined in the training data (i.e., unseen field-days principal GS classification). Of the three methods investigated, method III achieved the best accuracy for both classification tasks. The model achieved 97.3% and 97.5% GS classification accuracy for seen field-day test data for wheat and barley, respectively. The model also achieved accuracies of 93.5% and 92.2% for the principal GS classification task for wheat and barley, respectively. We provide a number of key research contributions: the collection curation and exposure of a unique GS labeled proximal image dataset of wheat and barley crops, GS classification, and principal GS classification of cereal crops using three different machine learning methods as well as a comprehensive evaluation and comparison of the obtained results. (C) The Authors. Published by SPIE under a Creative Commons Attribution 4.0 International License. Distribution or reproduction of this work in whole or in part requires full attribution of the original publication, including its DOI.
MoreTranslated text
Key words
convolutional neural network,transfer learning,deep learning,image processing,crop growth metric
AI Read Science
Must-Reading Tree
Example
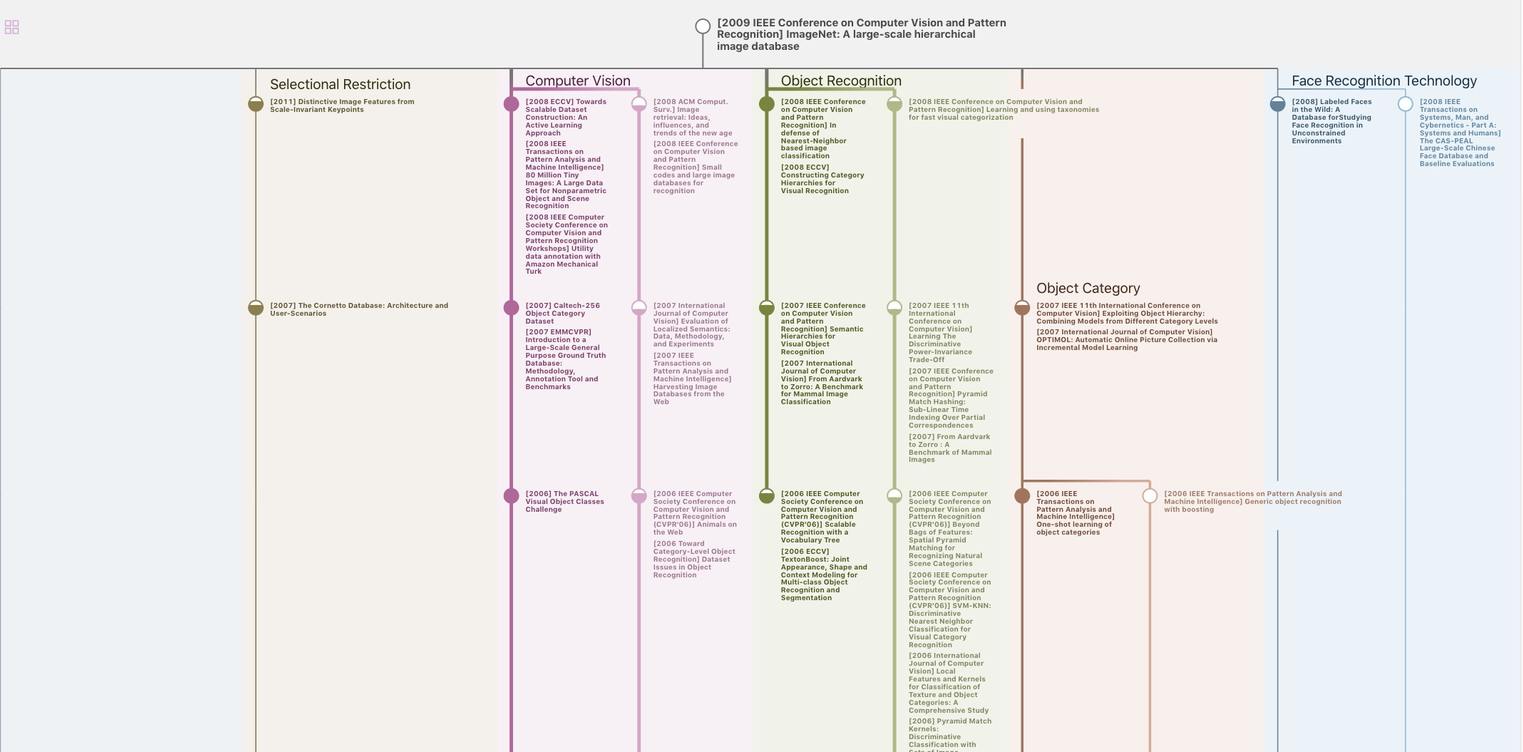
Generate MRT to find the research sequence of this paper
Chat Paper
Summary is being generated by the instructions you defined