Anomaly Localization for Copy Detection Patterns Through Print Estimations
2022 IEEE International Workshop on Information Forensics and Security (WIFS)(2022)
Key words
copy detection patterns,anomaly localization,anomaly detection,unsupervised deep learning
AI Read Science
Must-Reading Tree
Example
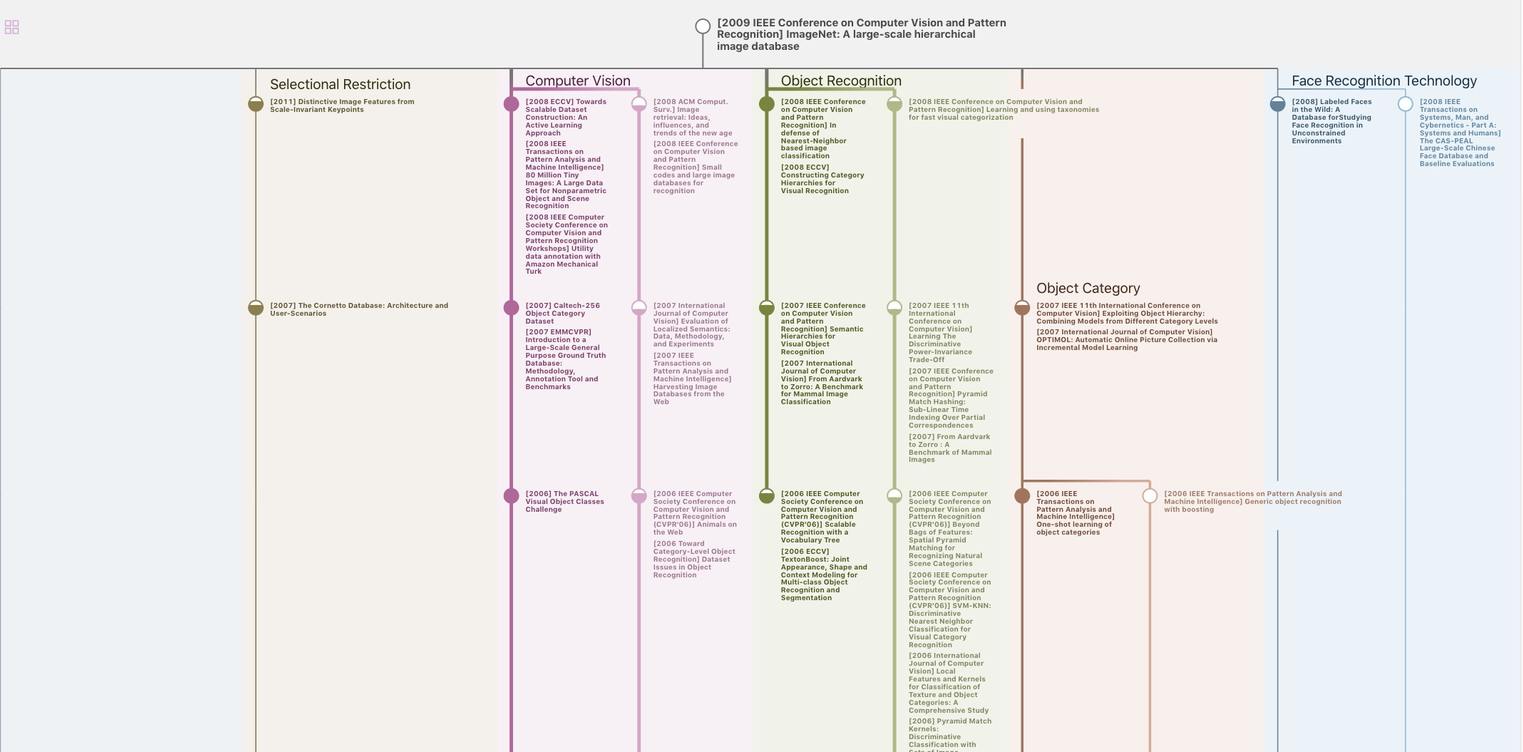
Generate MRT to find the research sequence of this paper
Chat Paper
Summary is being generated by the instructions you defined