Medical Question Understanding and Answering with Knowledge Grounding and Semantic Self-Supervision.
International Conference on Computational Linguistics(2022)
摘要
Current medical question answering systems have difficulty processing long, detailed and informally worded questions submitted by patients, called Consumer Health Questions (CHQs). To address this issue, we introduce a medical question understanding and answering system with knowledge grounding and semantic self-supervision. Our system is a pipeline that first summarizes a long, medical, user-written question, using a supervised summarization loss. Then, our system performs a two-step retrieval to return answers. The system first matches the summarized user question with an FAQ from a trusted medical knowledge base, and then retrieves a fixed number of relevant sentences from the corresponding answer document. In the absence of labels for question matching or answer relevance, we design 3 novel, self-supervised and semantically-guided losses. We evaluate our model against two strong retrieval-based question answering baselines. Evaluators ask their own questions and rate the answers retrieved by our baselines and own system according to their relevance. They find that our system retrieves more relevant answers, while achieving speeds 20 times faster. Our self-supervised losses also help the summarizer achieve higher scores in ROUGE, as well as in human evaluation metrics.
更多查看译文
关键词
knowledge grounding
AI 理解论文
溯源树
样例
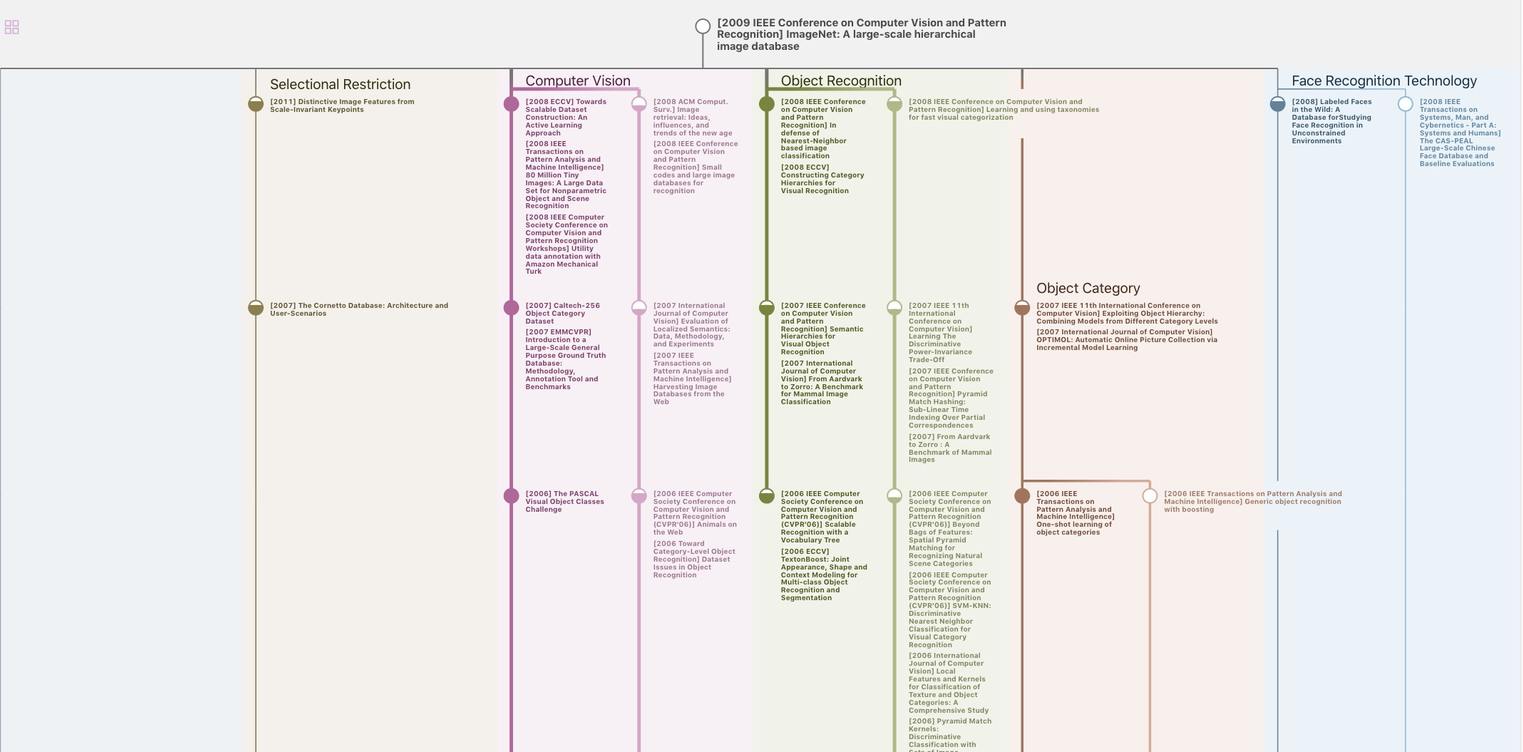
生成溯源树,研究论文发展脉络
Chat Paper
正在生成论文摘要