A Benchmark Comparison of Imitation Learning-based Control Policies for Autonomous Racing
arxiv(2022)
摘要
Autonomous racing with scaled race cars has gained increasing attention as an effective approach for developing perception, planning and control algorithms for safe autonomous driving at the limits of the vehicle's handling. To train agile control policies for autonomous racing, learning-based approaches largely utilize reinforcement learning, albeit with mixed results. In this study, we benchmark a variety of imitation learning policies for racing vehicles that are applied directly or for bootstrapping reinforcement learning both in simulation and on scaled real-world environments. We show that interactive imitation learning techniques outperform traditional imitation learning methods and can greatly improve the performance of reinforcement learning policies by bootstrapping thanks to its better sample efficiency. Our benchmarks provide a foundation for future research on autonomous racing using Imitation Learning and Reinforcement Learning.
更多查看译文
关键词
autonomous driving,autonomous racing,bootstrapping,control policies,interactive imitation learning,planning algorithm,race cars,reinforcement learning policies
AI 理解论文
溯源树
样例
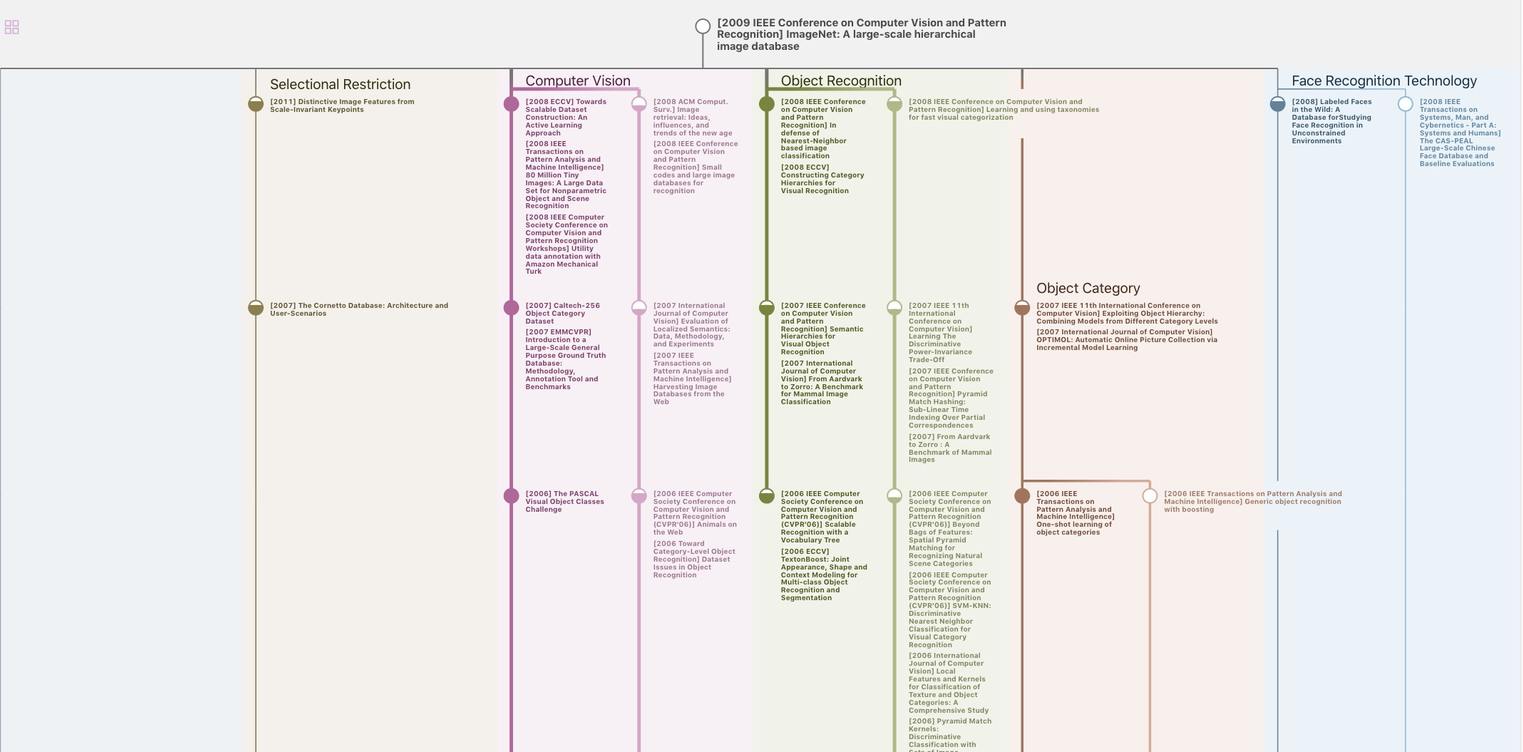
生成溯源树,研究论文发展脉络
Chat Paper
正在生成论文摘要