Completed sample correlations and feature dependency-based unsupervised feature selection
Multimedia Tools and Applications(2022)
Abstract
Sample correlations and feature relations are two pieces of information that are needed to be considered in the unsupervised feature selection, as labels are missing to guide model construction. Thus, we design a novel unsupervised feature selection scheme, in this paper, via considering the completed sample correlations and feature dependencies in a unified framework. Specifically, self-representation dependencies and graph construction are conducted to preserve and select the important neighbors for each sample in a comprehensive way. Besides, mutual information and sparse learning are designed to consider the correlations between features and to remove the informative features, respectively. Moreover, various constraints are constructed to automatically obtain the number of important neighbors and to conduct graph partition for the clustering task. Finally, we test the proposed method and verify the effectiveness and the robustness on eight data sets, comparing with nine state-of-the-art approaches with regard to three evaluation metrics for the clustering task.
MoreTranslated text
Key words
Unsupervised learning,Sample correlation,Unsupervised feature selection,Graph learning,Self-representation,Mutual information,Sparse learning
AI Read Science
Must-Reading Tree
Example
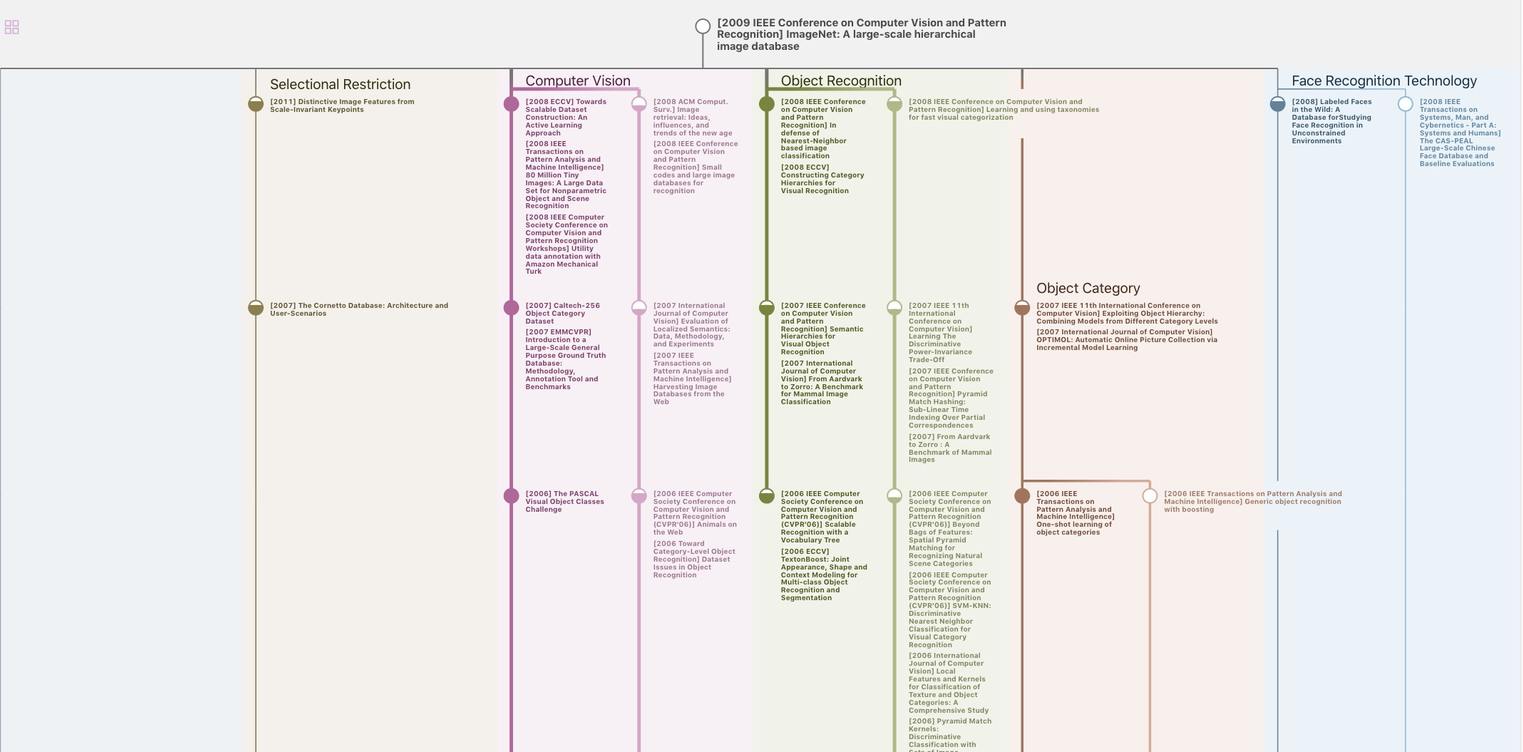
Generate MRT to find the research sequence of this paper
Chat Paper
Summary is being generated by the instructions you defined