Warehouse LSTM-SVM-Based ECG Data Classification With Mitigated Device Heterogeneity
IEEE Transactions on Computational Social Systems(2022)
摘要
Device heterogeneity is a social concern, especially in healthcare domain. In this work, we mitigate the problem of device heterogeneity and further classify the healthcare electrocardiogram (ECG) data with improved performance using a proposed variant of long short-term memory (LSTM). ECG data sensed from different devices are used in this work for experimentation. Device heterogeneity is addressed using the proposed multiplicative convergence-based heterogeneity mitigation (MCHM) method. The proposed warehouse LSTM, in addition to support vector machine (SVM), is leveraged in this work for healthcare data classification. The warehouse LSTM keeps a track of the data that are considered as insignificant in the initial epoch. We mitigate heterogeneity in medical devices and reduce the root-mean-squared error to the order of 10−6–10−5. Using the warehouse, the LSTM-SVM attains the classification accuracies of 98.34% and 96.27% during training on the MIT-BIH and PTB datasets, respectively. The proposed MCHM method increases the reliability on the usage of devices from multiple manufacturers. The novel warehouse LSTM-SVM model also outperforms the existing methods for classification of data.
更多查看译文
关键词
Device heterogeneity,healthcare,long short-term memory (LSTM),machine learning,support vector machine (SVM)
AI 理解论文
溯源树
样例
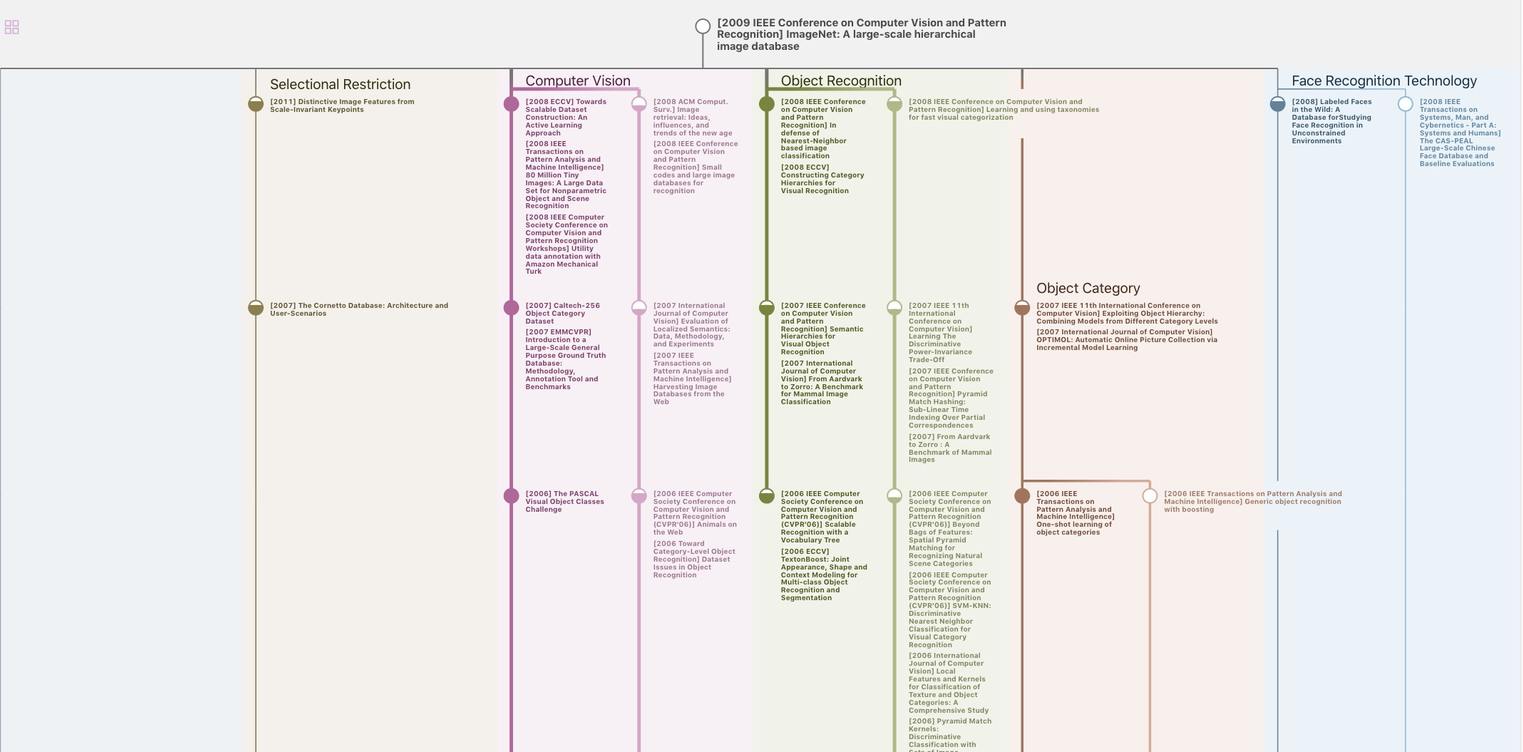
生成溯源树,研究论文发展脉络
Chat Paper
正在生成论文摘要