Surface electromyography classification using extreme learning machines and echo state networks
Research on Biomedical Engineering(2022)
摘要
Purpose Loss of movement in the upper limb is a limitation that affects the lives of many individuals. This problem motivates the creation of several solutions, from intelligent biomechanical prostheses to new physical therapy approaches. However, one of the main limitations of these applications is in the detection and recognition of signal patterns for movement classification, considering both accuracy and computational complexity. The main hypothesis of this work is that extreme learning machines and echo state machines can combine acceptable accuracy with the low memory usage and processing typical of these machine learning models, in order to promote greater autonomy to users. In this work, we proposed a simple method for electromyography (EMG) classification, disregarding feature selection and post-processing algorithms. Method In this work, we propose the use of extreme learning machines and echo state machines as a low computational cost alternative for motion recognition from myoelectric signals. Other classifier models, such as the multilayer perceptron and linear discriminant analysis, were investigated. To validate the proposal, we used two datasets that represent two scenarios: an ideal environment, with no data on amputees; and a large dataset with myoelectric signals from patients with amputation. The results were evaluated as a function of accuracy and kappa index. Results Using an extreme learning machine, the results for the dataset of healthy individuals reached 99.10% accuracy, with a processing time per instance of 0.09 ms. Considering the amputee dataset, the best results were obtained with the use of extreme learning machine augmentation, with an accuracy of 75.60% and a kappa of 0.7507. Conclusion We showed that Extreme Learning Machines without pre- and post-data processing is feasible for real-time application, presenting good performance and increased velocity when compared to usual algorithms. Also, data augmentation appears to be a good approach for robustly classifying unbalanced EMG datasets.
更多查看译文
关键词
Electromyography,Echo State Machines,Extreme Learning Machines,Feature Extraction,Hand Gestures
AI 理解论文
溯源树
样例
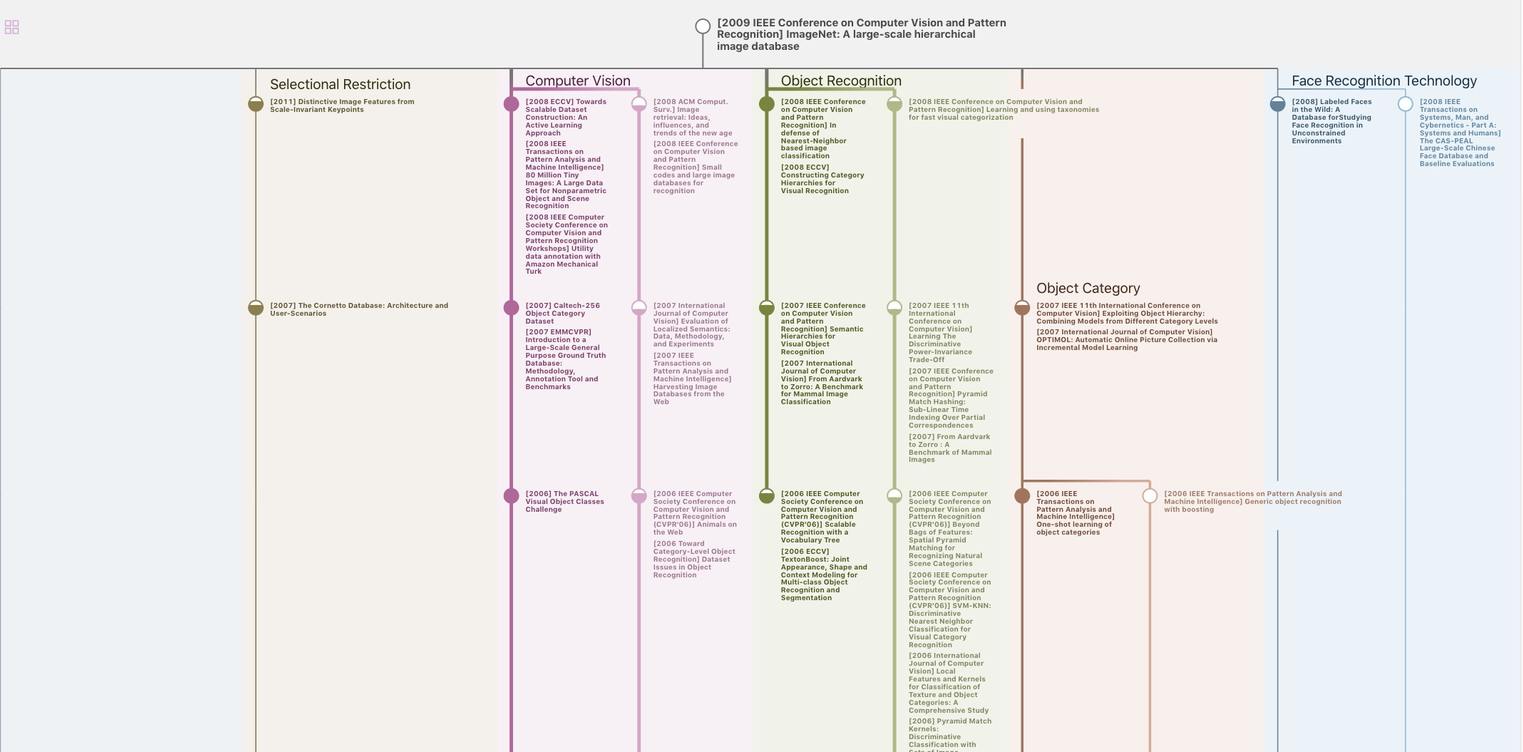
生成溯源树,研究论文发展脉络
Chat Paper
正在生成论文摘要