Feasibility of using convolutional neural networks for individual-identification of wild Asian elephants
Mammalian Biology(2022)
摘要
Individual identification is a basic requirement for research in behavior, ecology and conservation. Photographic records are commonly used in situations where individuals are visually distinct. However, keeping track of identities becomes challenging with increasing population sizes and corresponding datasets. There is growing interest in the potential of deep-learning methods for computer vision to assist with automating this task. Here we apply Convolutional Neural Networks, a popular architecture for Artificial Neural Networks used in image classification, to the problem of identifying individual Asian elephants through photographs. We evaluate the performance of five different types of CNN models used in facial recognition (VGG16, ResNet50, InceptionV3, Xception, and Alexnet), on datasets representing three different feature regions (the full body, face, and ears), trained with two techniques (transfer learning vs. training from scratch) for n = 56 elephants. We tested accuracy in matching the top candidate as well as top five candidates. We found that VGG16 trained with the transfer-learning technique outperformed other models on the body and face datasets with accuracies of 21.34% and 42.35%, respectively, in matching the top candidate. Nevertheless, the best performance was achieved by an Xception model trained from the scratch on the ear dataset, with an accuracy of 89.02% for matching the top candidate and 99.27% for including the correct individual among the top five. However, this impressive level of accuracy was obtained with a dataset of 3816 labeled training images of 56 elephants. There are more than 1000 wild elephants in the population under observation, requiring extensive human effort and skill to initially annotate the images used as training data. Therefore, we consider this approach impractical for monitoring large wild populations. Nevertheless this it could be very useful in record keeping and fraud prevention for large captive elephant populations, as well as monitoring animals that have been rehabilitated and released or moved for management purposes.
更多查看译文
关键词
Artificial Intelligence,Computer Vision,Image Classification,Individual-Identification,Machine Learning,Pattern Recognition
AI 理解论文
溯源树
样例
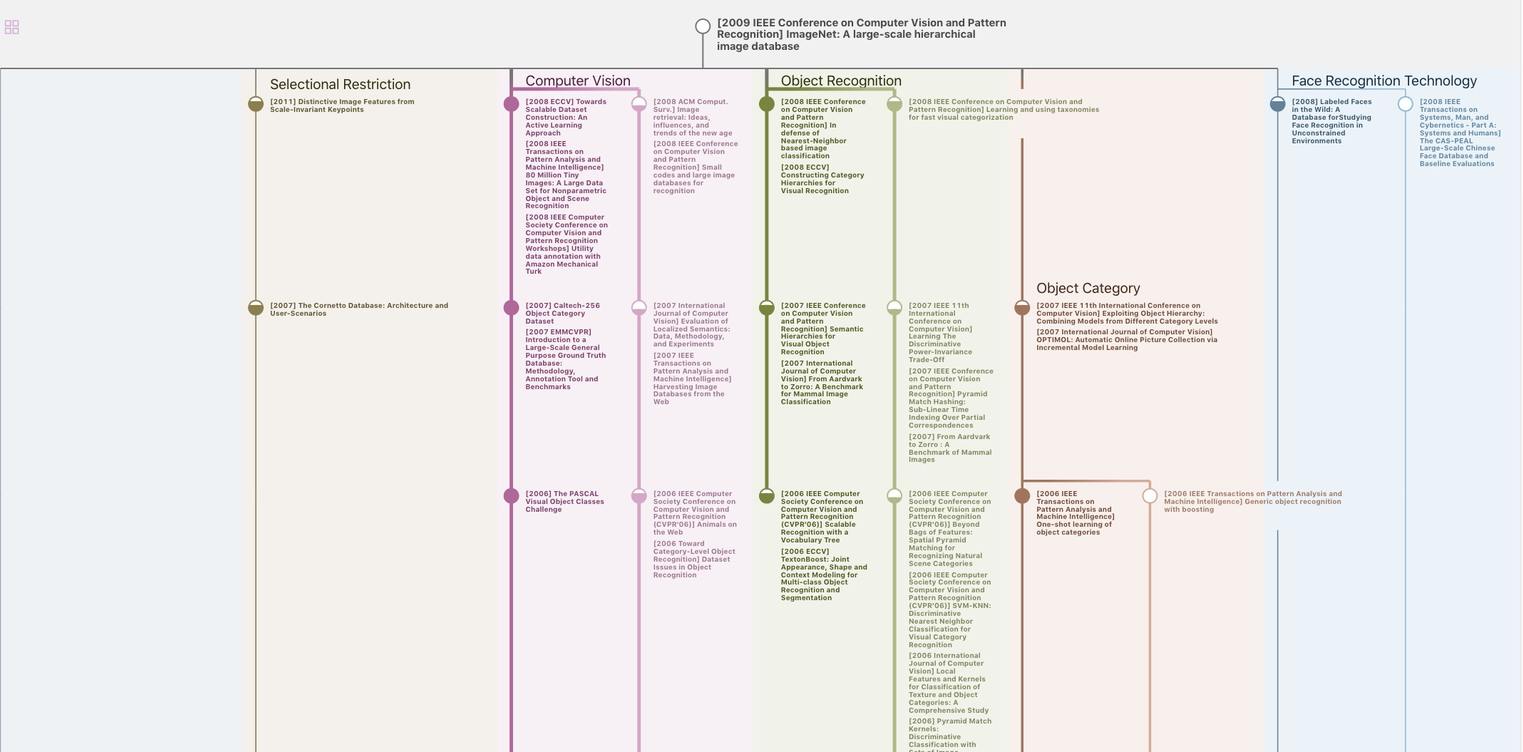
生成溯源树,研究论文发展脉络
Chat Paper
正在生成论文摘要