Self-Attention-Based Machine Theory of Mind for Electric Vehicle Charging Demand Forecast
IEEE Transactions on Industrial Informatics(2022)
摘要
The popularization of electric vehicles (EVs) and charging stations has been threatening the distribution network’s reliability and efficiency. The prediction of EV charging demand can benefit the optimization of the operation of energy-transportation nexus and improve social welfare toward a low carbon future. In this article, a short-term probabilistic charging demand forecast model is proposed to estimate the quantiles of future charging demand of a charging station 15 min ahead, i.e., the self-attention-based machine theory of mind (SAMToM). The SAMToM has considered both the users’ historical charging habits (schedules) and the current trend of charging demand variation using the framework of machine theory of mind (MToM), and real-world-data-based case studies have verified its superiority in EV charging demand forecast over state-of-the-arts. Moreover, analyses show that the advantage of SAMToM lies in the following aspects. 1) The self-attention layers have mitigated the long-range forgetting in SAMToM. 2) The MToM architecture enables SAMToM to balance historical charging habits and current charging demand variation trends well. 3) Using a quantile forecast evaluation metric as the loss function, i.e., the continuous ranked probability score (CRPS), enables SAMToM to aim directly at the highest quality of forecasted quantiles.
更多查看译文
关键词
Attention mechanism,charging demand forecast,deep learning,electric vehicles (EVs),energy and transportation informatics
AI 理解论文
溯源树
样例
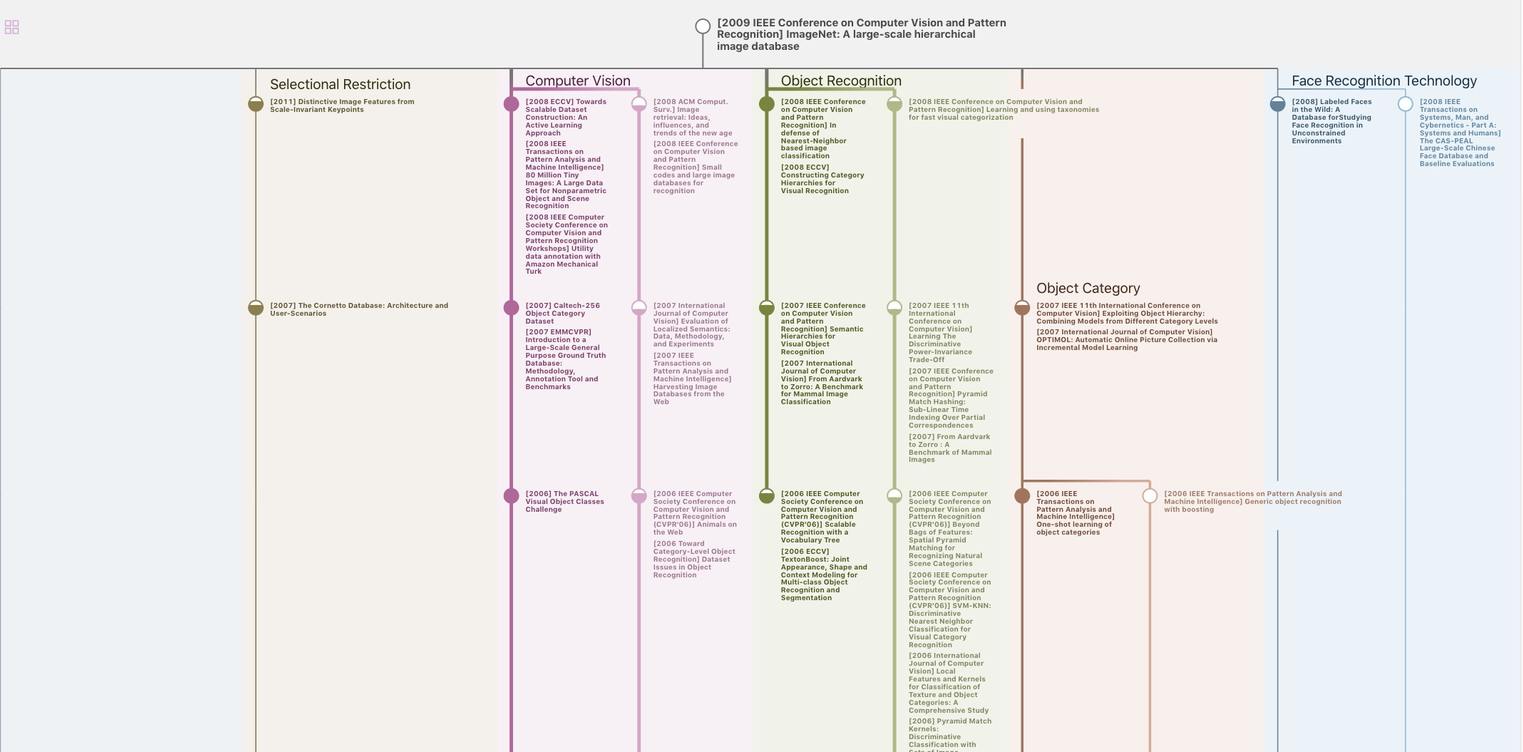
生成溯源树,研究论文发展脉络
Chat Paper
正在生成论文摘要