DEEP-FEL: Decentralized, Efficient and Privacy-Enhanced Federated Edge Learning for Healthcare Cyber Physical Systems
IEEE Transactions on Network Science and Engineering(2022)
摘要
The rapid development of Internet of Things (IoT) stimulates the innovation for the health-related devices such as remote patient monitoring, connected inhalers and ingestible sensors. Simultaneously, with the aid of numerous equipments, a great number of collected data can be used for disease prediction or diagnosis model establishment. However, the potential patient data leak will also bring privacy and security issues in the interaction period. To deal with these existing issues, we propose a decentralized, efficient, and privacy-enhanced federated edge learning system called DEEP-FEL, which enables medical devices in different institutions to collaboratively train a global model without raw data mutual exchange. Firstly, we design a hierarchical ring topology to alleviate centralization of the conventional training framework, and formulate the ring construction as an optimization problem, which can be solved by an efficient heuristic algorithm. Subsequently, we design an efficient parameter aggregation algorithm for distributed medical institutions to generate a new global model, and the total amount of data transmitted by N nodes is only
$2/N$
times that of traditional algorithm. In addition, data security among different medical institutions is enhanced by adding artificial noise to the edge model. Finally, experimental results on three medical datasets demonstrate the superiority of our system.
更多查看译文
关键词
Cyber physical systems,mobile healthcare,federated learning,decentralized system,differential privacy
AI 理解论文
溯源树
样例
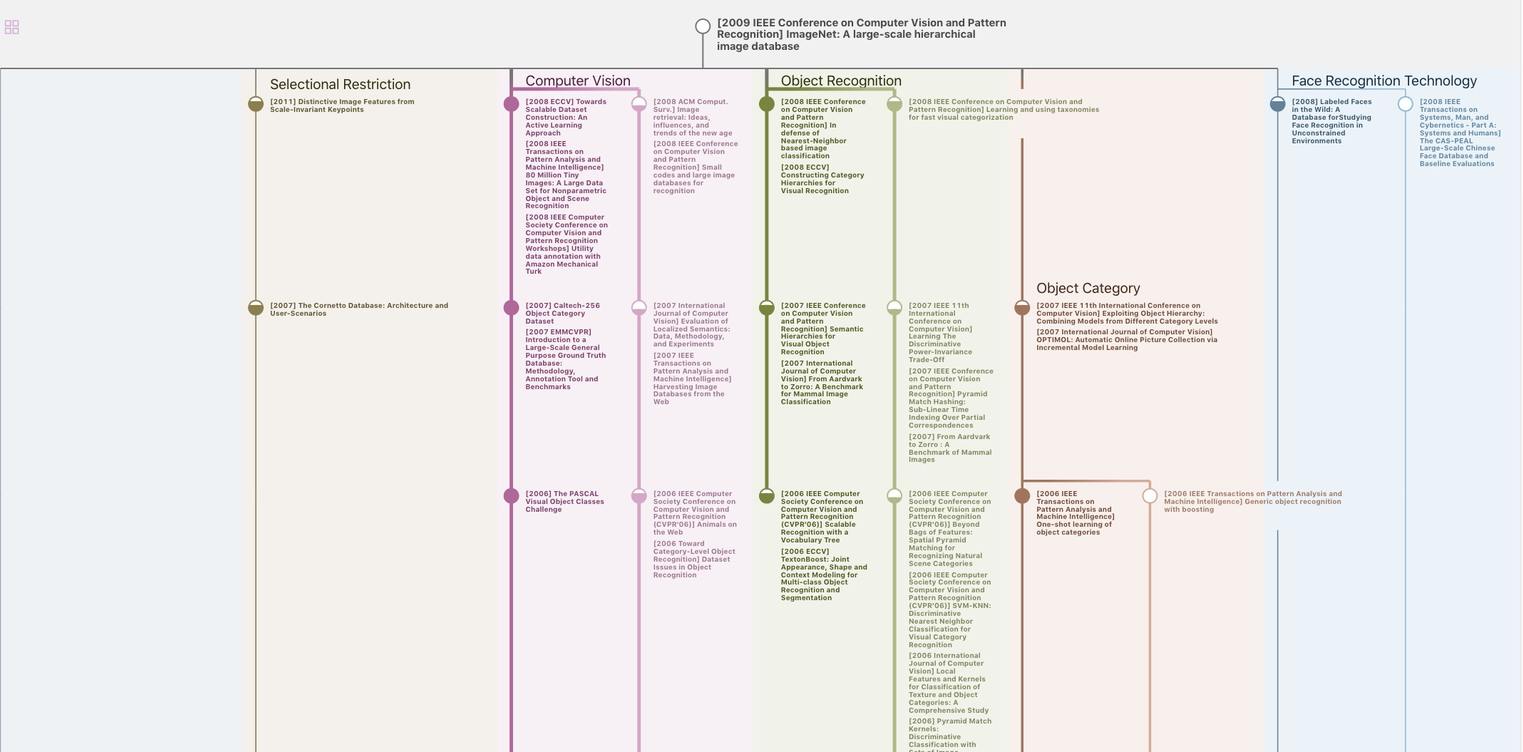
生成溯源树,研究论文发展脉络
Chat Paper
正在生成论文摘要