Explanation of HRV Features for Detecting Atrial Fibrillation
SN Computer Science(2022)
摘要
ial fibrillation is a heart condition that is known to be affecting approximately 33 million people in the world and cause a wide range of complications, that may at times be fatal if not properly treated. Many studies try to address this condition by either training highly accurate artificial intelligence detector models or by explaining the physiological significance of certain features used to train these detector models. However, the problem with these kind of studies is that it leaves its users to either blindly trust the calls of a black box model, or merely inform themselves of various features. In this study, we try to address this problem by revealing which features are most frequently chosen by artificial intelligence models to detect atrial fibrillation and also try to explain why they might be so significant. To achieve this, heart rate variability features are extracted from the ‘PhysioNet/Computing in Cardiology (CinC) Challenge 2017’ dataset and are used to train machine learning models like Random Forest and XGBoost, with Recursive Feature Elimination. We find that many machine learning algorithms find the ‘hr_std’, ‘sd_ratio’, ‘nni_mean’, and ‘pnn20’ features to be most important in detecting atrial fibrillation. We achieve a mean F _1 -score of 98.13
更多查看译文
关键词
Artificial intelligence, Atrial fibrillation, Explainability, Heart rate variability, Machine learning
AI 理解论文
溯源树
样例
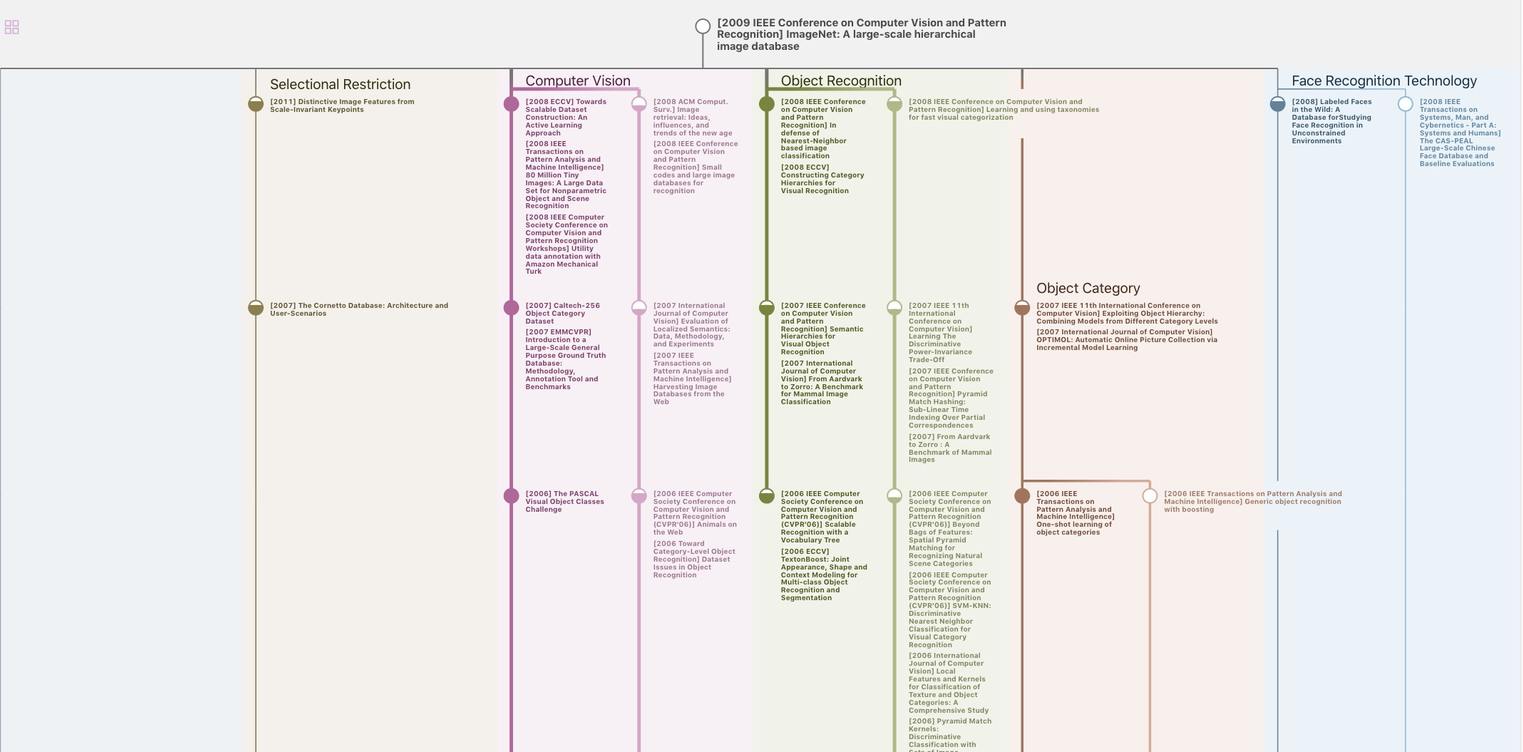
生成溯源树,研究论文发展脉络
Chat Paper
正在生成论文摘要