Denoising Latent Representation with SOMs for Unsupervised IoT Malware Detection
SN Computer Science(2022)
摘要
The autoencoder-based latent representations have been widely developed for unsupervised learning in cyber-security domain, and has shown remarkable performance. Our previous work has introduced a hybrid autoencoders (AEs) and self-organizing maps (SOMs) for unsupervised IoT malware detection. However, the paper has only examined the characteristics of the latent representation of ordinary AEs in comparison to that of principle component analysis (PCA) on various IoT malware scenarios. This paper extends the work by employing denoising AEs (DAEs) to enhance the generalization ability of latent representations as well as optimizing hyper-parameters of SOMs to improve the hybrid performance. Particularly, this aims to further examine the characteristics of AE-based structure models (i.e., DAE) for identifying unknown/new IoT attacks and transfer learning. Our model is evaluated and analyzed extensively in comparison with PCA and AEs by a number of experiments on the NBaIoT dataset. The experimental results demonstrate that the latent representation of DAEs is often superior to that of AEs and PCAs in the task of identifying IoT malware.
更多查看译文
关键词
AutoEncoders, Denoising autoencoders, Self-organizing maps, IoT malware, IoT anomaly detection, Transfer learning
AI 理解论文
溯源树
样例
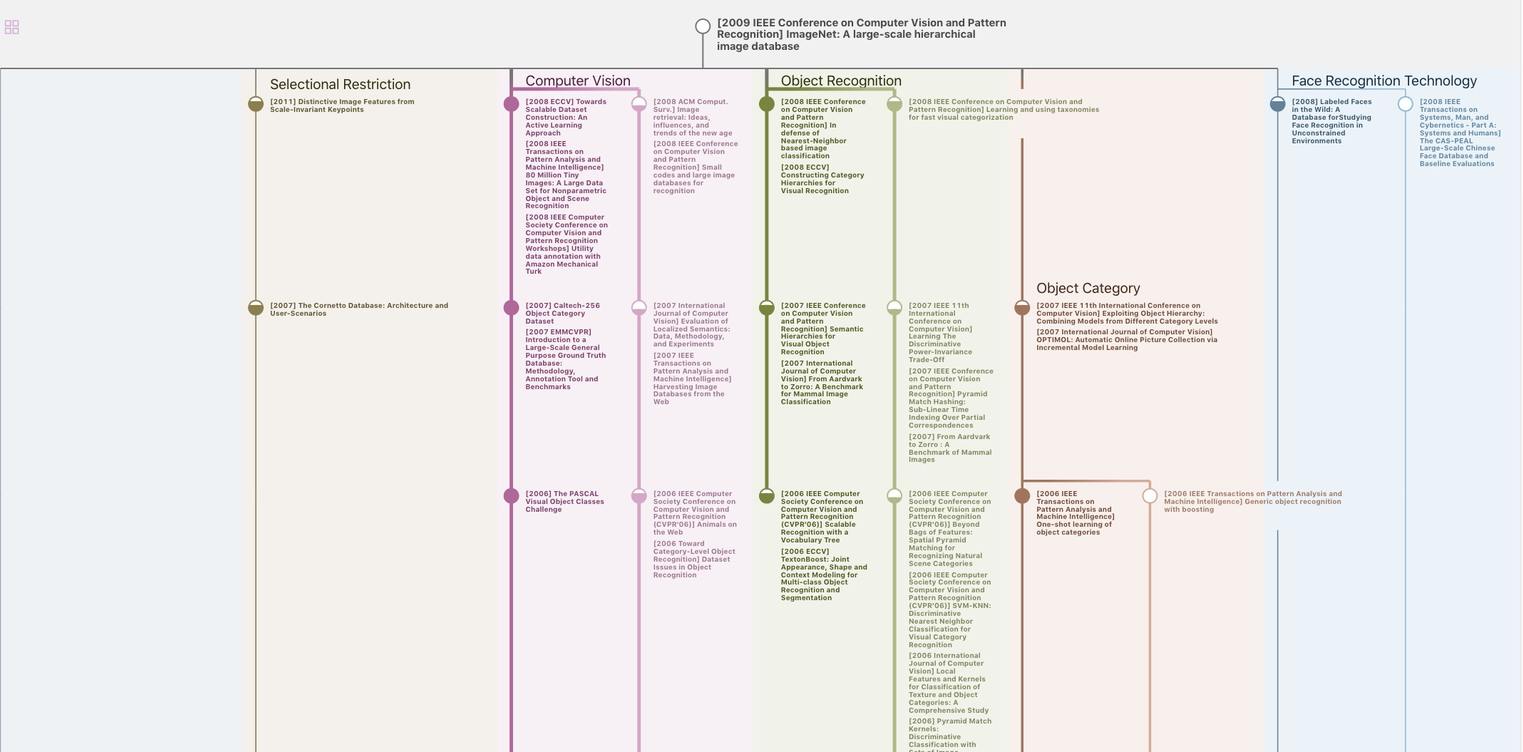
生成溯源树,研究论文发展脉络
Chat Paper
正在生成论文摘要