Real-Time Object Detection Based on YOLO-v2 for Tiny Vehicle Object
SN Computer Science(2022)
摘要
Object detection plays an essential role in automatic driving system (ADS) and driver assistance system (DAS). However, existing real-time detection models for tiny vehicle objects have the problems of low precision and poor performance. To solve these issues, a novel real-time object detection model based on You Only Look Once Version 2 (YOLO-v2) deep learning framework is proposed for tiny vehicle objects, called Optimized You Only Look Once Version 2 (O-YOLO-v2). In the proposed model, a new architecture is introduced by adding the convolution layers at different locations to enhance the feature extraction ability of network. At the same time, the residual modules are added to solve the problem of gradient disappearance or dispersion caused by the increase of network depth. Furthermore, the low-level features and high-level features of the network are combined to improve the detection accuracy of tiny vehicle objects. Experimental results on the KITTI dataset show that the model not only improves the accuracy of tiny vehicle object detection but also improves the accuracy of vehicle detection (the accuracy reaches 94%) without decreasing the detection speed.
更多查看译文
关键词
Tiny vehicle detection, Deep learning, YOLO-v2, Residual module, Feature fusion
AI 理解论文
溯源树
样例
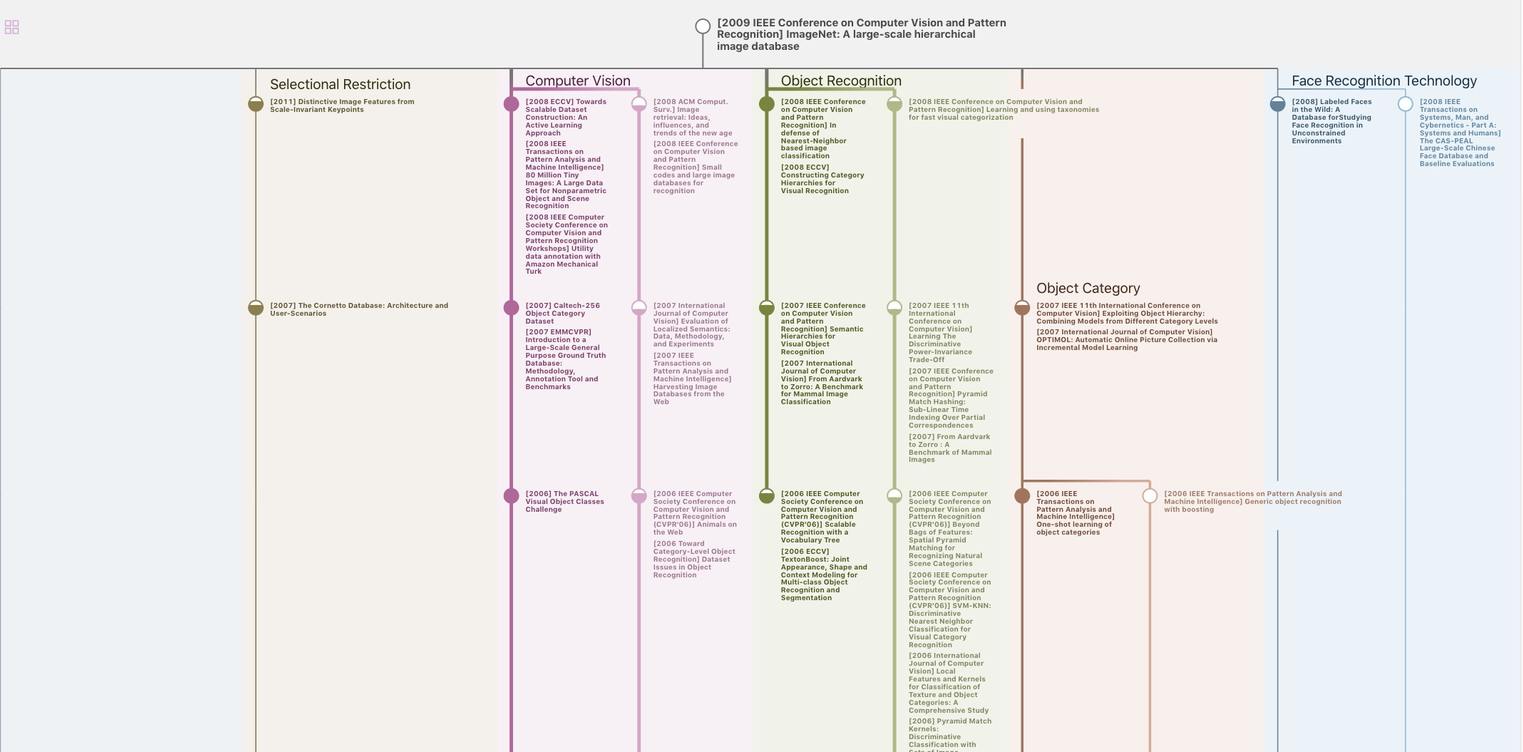
生成溯源树,研究论文发展脉络
Chat Paper
正在生成论文摘要