Imbalance domain adaptation network with adversarial learning for fault diagnosis of rolling bearing
Signal, Image and Video Processing(2022)
Abstract
Domain adaptation has been widely used in industrial fault diagnosis. However, it requires a balance of data distribution between the source domain and the target domain. Unfortunately, cross-domain balanced distribution is not common in actual applications, bringing difficulties to the practical application of domain adaptation. In the article, we focus on this difficult problem and propose a new imbalance domain adaptation network with adversarial learning (IDAL). The model applies adversarial learning to data augmentation of the target domain and uses the domain adaptation based on a neural network to narrow the feature distribution discrepancy between the source and target domains. Ultimately, the parameters are transferred to the target domain and fine-tuned with a small number of labeled samples so as to achieve fault diagnosis. The accuracy of the proposed method on two data sets is more than 98%. It is worth noting that common deep learning networks can be embedded in IDAL, so the model can be widely used in different industrial scenarios.
MoreTranslated text
Key words
Adversarial learning, Deep learning, Fault diagnosis, Imbalance domain adaptation
AI Read Science
Must-Reading Tree
Example
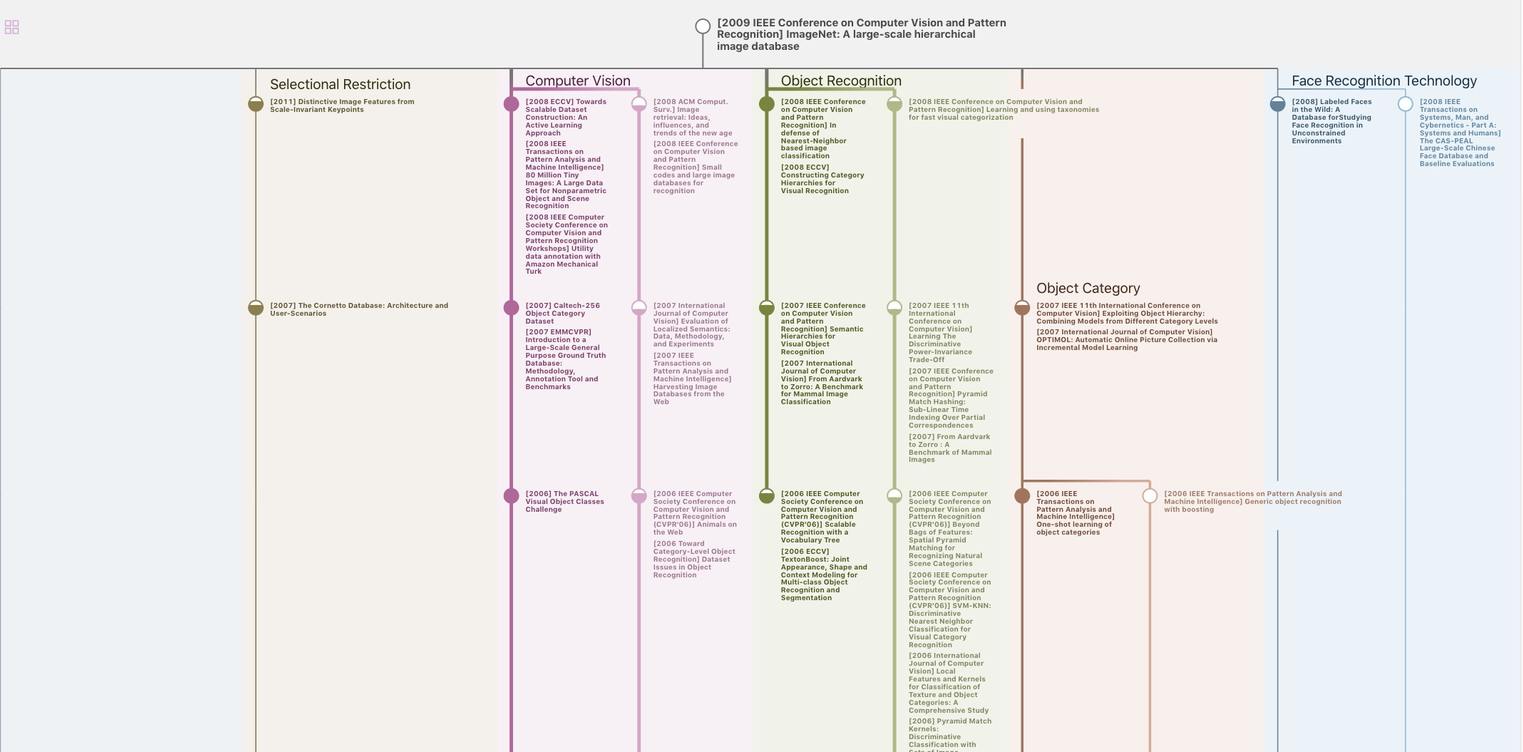
Generate MRT to find the research sequence of this paper
Chat Paper
Summary is being generated by the instructions you defined