Radial basis function-assisted adaptive differential evolution using cooperative dual-phase sampling for high-dimensional expensive optimization problems
Structural and Multidisciplinary Optimization(2022)
摘要
To improve the global convergence and optimization efficiency for solving high-dimensional expensive black-box optimization problems, a radial basis function-assisted adaptive differential evolution using cooperative dual-phase sampling (RBF-ADE) is proposed in this paper. In RBF-ADE, the radial basis function is integrated with the differential evolution framework to approximate the expensive functions. During the evolutionary process, a population-based construction region identification mechanism is developed to select training sample points from the database for RBF construction, which simultaneously improves the modeling efficiency and prediction accuracy of RBF surrogates. RBF is adaptively refined during the optimization via a cooperative dual-phase sampling mechanism, which produces infill samples in the vicinity of the global optimum to improve the competitiveness of the current population. Several high-dimensional numerical benchmarks are used to test the performance of RBF-ADE. Comparison results show that RBF-ADE has better global convergence than the competitive surrogate-assisted evolutionary algorithms with the same computational budget. Finally, RBF-ADE is successfully applied to a 50-dimensional airfoil aerodynamic design optimization problem and an all-electric geostationary orbit satellite multidisciplinary design optimization problem to demonstrate its practicality and effectiveness in engineering practices.
更多查看译文
关键词
Radial basis function, Differential evolution, Surrogate-based optimization, High-dimensional optimization, Design optimization
AI 理解论文
溯源树
样例
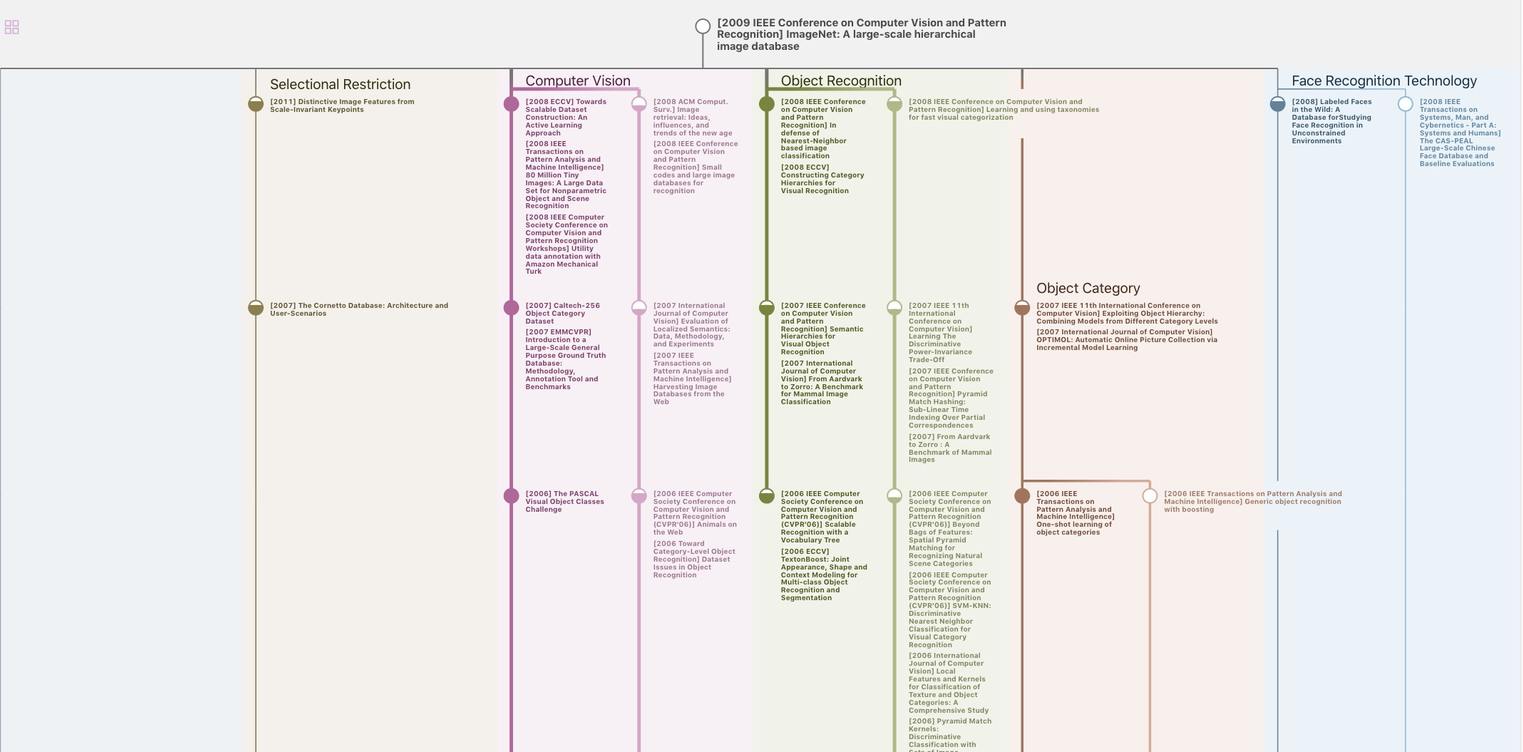
生成溯源树,研究论文发展脉络
Chat Paper
正在生成论文摘要