Spatiotemporal context-aware network for video salient object detection
Neural Computing and Applications(2022)
摘要
It has been witnessed that there is an increasing interest in video salient object detection (VSOD) in computer vision field. Different from image salient object detection (ISOD), VSOD not only requires appearance information but also needs motion cues. Thus, it is essential to exploit spatiotemporal information to generate accurate saliency results. Existing VSOD models mainly combine an ISOD model with long short-term memory (LSTM) or flow-estimation modules to integrate saliency cues estimated from spatial and temporal domain. However, flow-estimation modules heavily rely on optical flow images; the generation process of which is rather time-consuming and severely limits its applications in practice. Besides, the LSTM can only exploit motion cues via a step-by-step propagation in the time domain and is hard to realize the multi-scale spatiotemporal interaction. In this paper, we propose the SCANet to solve the above problems. Specifically, we develop the pyramid dilated 3D convolutional (PD3C) module to generate rich temporal features by leveraging context information. Besides, a feature aggregation module is designed to effectively integrate spatial and temporal features. Equipped with these modules, the SCANet is capable of generating high-quality saliency maps at more than real-time inference speed (41 FPS on a single Titan Xp GPU). Extensive experimental results on six widely used benchmark datasets prove that SCANet outperforms state-of-the-art methods in terms of three standard evaluation metrics. Our code will be publicly available at https://github.com/clelouch/SCANet .
更多查看译文
关键词
Deep learning,Multi-level feature integration,Spatiotemporal deep features,Video salient object detection
AI 理解论文
溯源树
样例
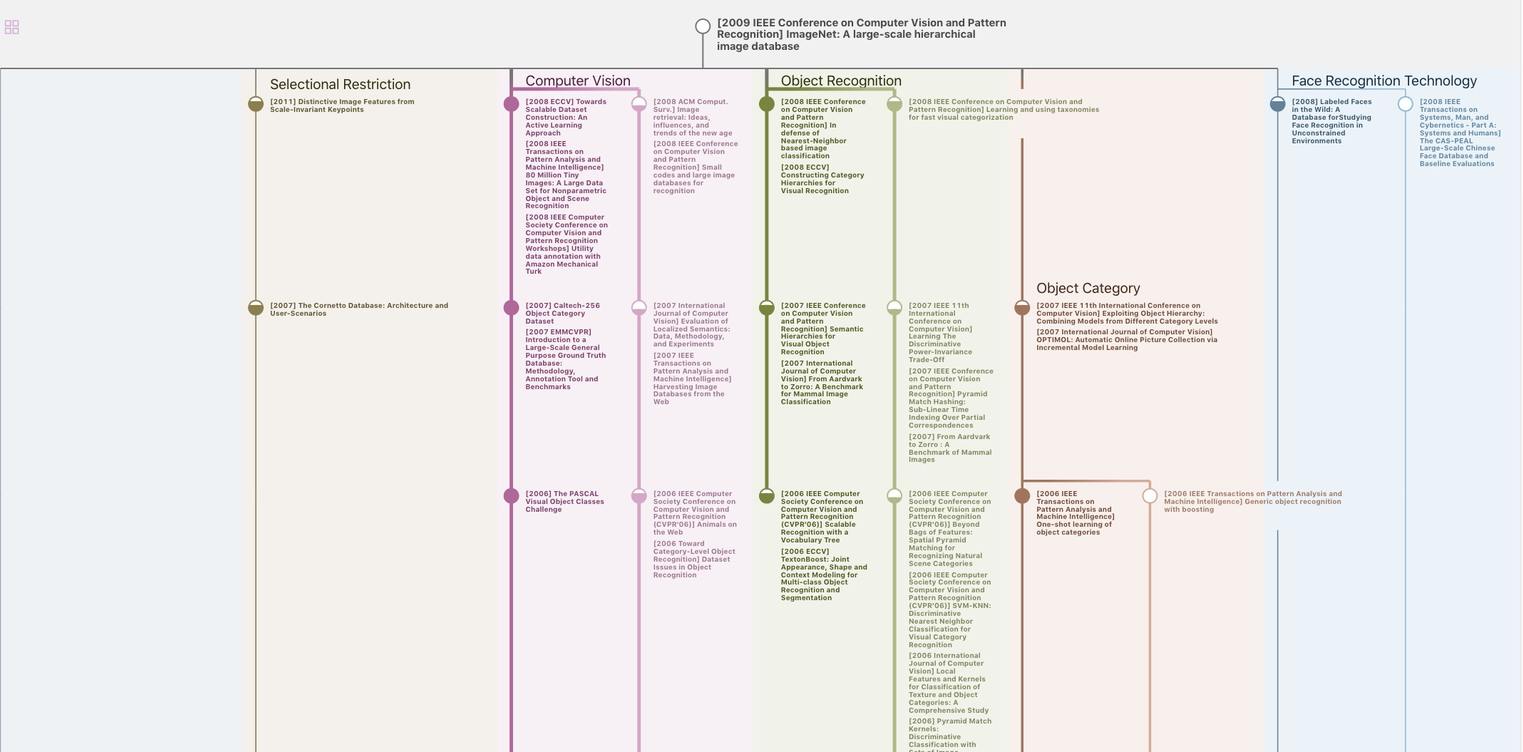
生成溯源树,研究论文发展脉络
Chat Paper
正在生成论文摘要