Learning Bayesian network parameters with soft-hard constraints
Neural Computing and Applications(2022)
Abstract
In Bayesian network parameter learning, it is difficult to obtain accurate parameters when the data are insufficient, and overfitting easily occurs. However, underfitting is prone to happen when the learning results are blindly close to the constraints generated by expert knowledge. A soft-hard constraint parameter learning method is proposed to balance the overfitting and underfitting problems in parameter learning. In this paper, the constraints applied to the parameters are called hard constraints, while those used to the prior are called soft constraints. A partial maximum entropy prior quantization method is proposed for the soft constraint to obtain proper parameters. The equivalent sample threshold is proposed to limit the hyperparameters based on the actual data size for the hard constraint. To combine soft and hard constraints effectively, the soft constraint maximization and hard constraint minimization model is proposed. Experimental results show that this method can significantly improve the accuracy of parameter learning and actually balance overfitting and underfitting.
MoreTranslated text
Key words
Bayesian networks,Parameter learning,Overfitting,Underfitting
AI Read Science
Must-Reading Tree
Example
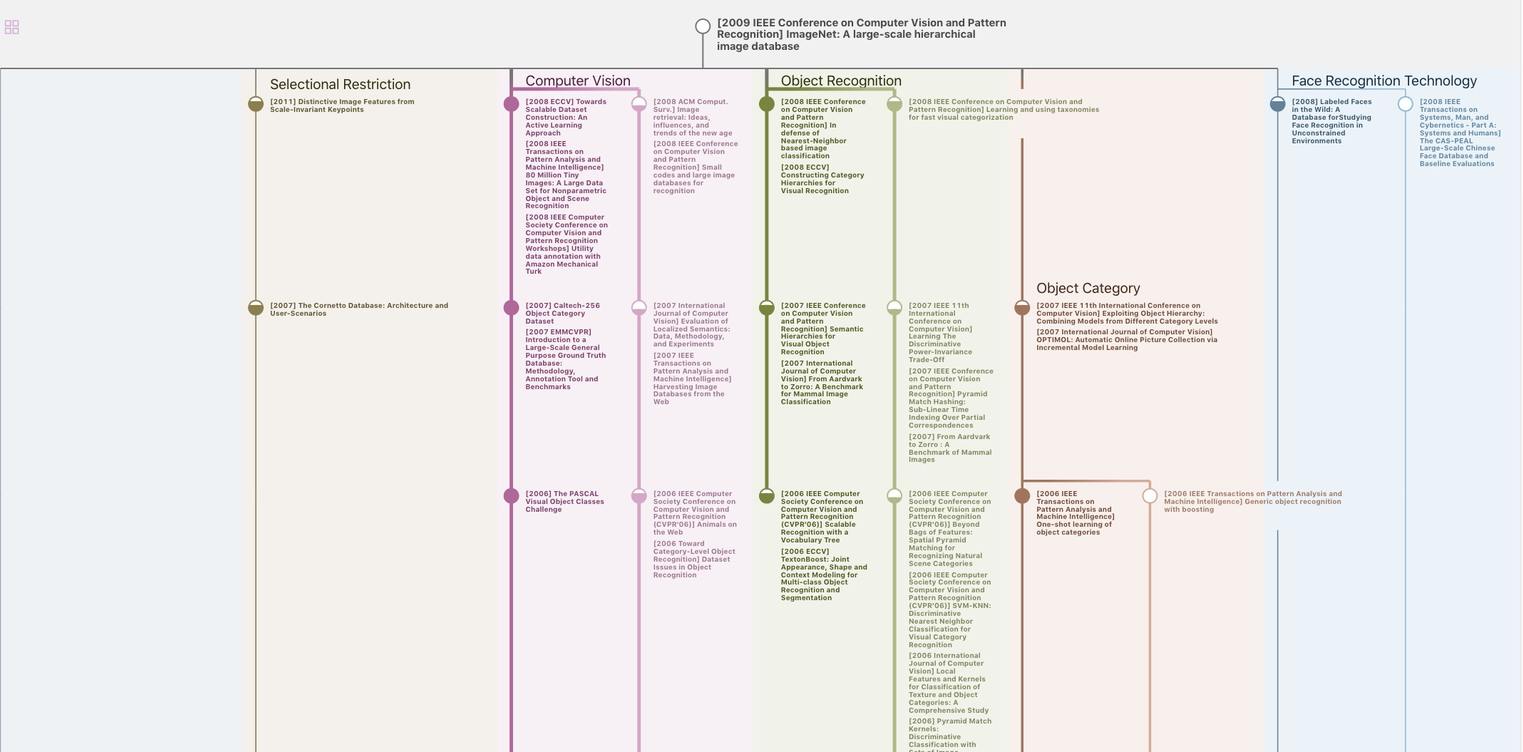
Generate MRT to find the research sequence of this paper
Chat Paper
Summary is being generated by the instructions you defined