Multi-view Learning for EEG Signal Classification of Imagined Speech
Pattern Recognition(2022)
摘要
Multi-view Learning (MVL) has the objective of combining the information that describes an object from different groups of features. This machine learning paradigm has proven useful to improve generalization performance of classifiers by taking advantage of the complementary information from different views of the same object. This work explores the use of three Co-training-based methods and three Co-regularization techniques to perform supervised learning to classify electroencephalography signals (EEG) of imagined speech. Two different views were used to characterize these signals, extracting Hjorth parameters and the average power of the signal. The results of six different approaches of MVL applied to classify the imagined speech of five different words are reported, showing an improvement up to 14.27% in accuracy average of classification compared with single view classification.
更多查看译文
关键词
Multi-view learning, Co-training, Co-regularization, EEG, Imagined speech
AI 理解论文
溯源树
样例
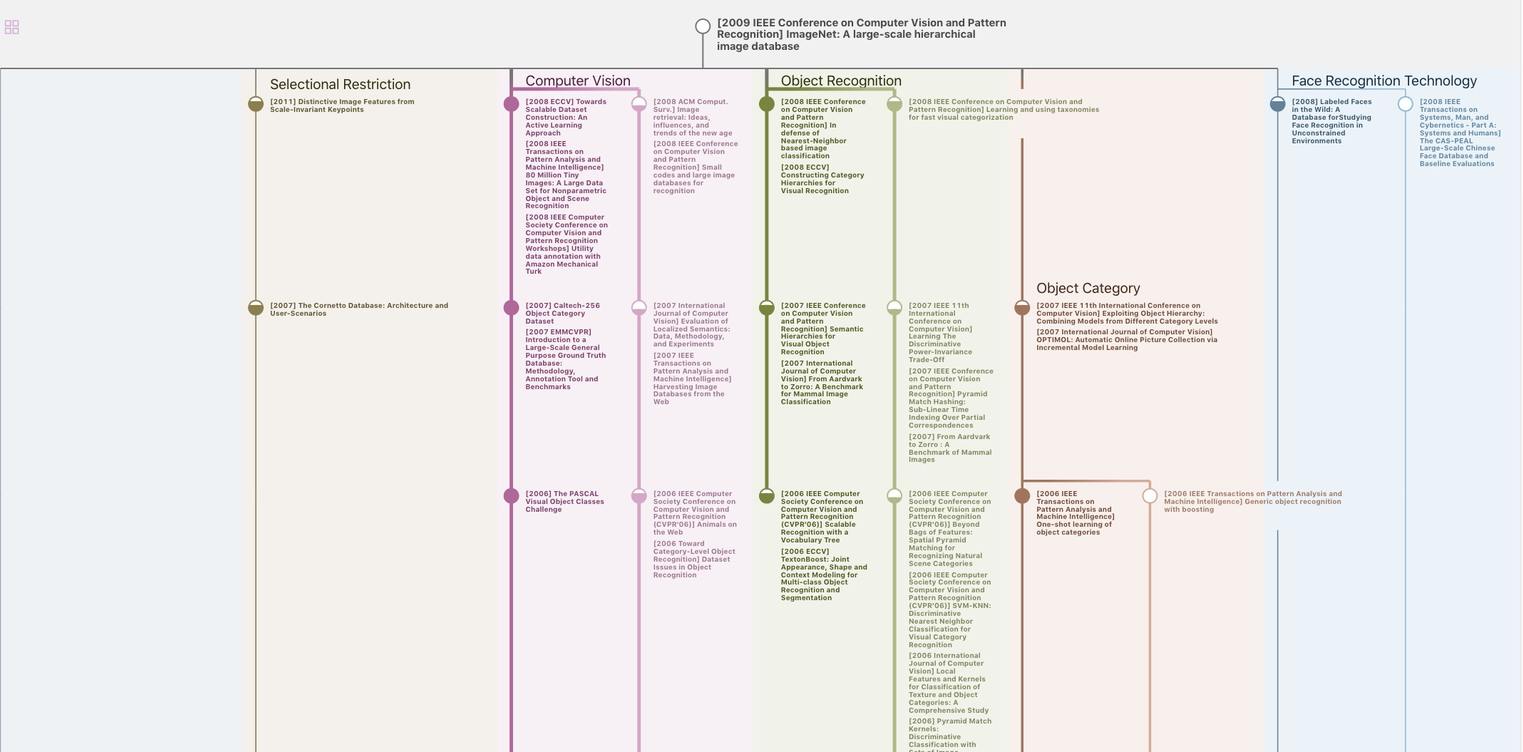
生成溯源树,研究论文发展脉络
Chat Paper
正在生成论文摘要