Comparison of Estimation Algorithms for Latent Dirichlet Allocation
Quantitative Psychology(2022)
摘要
Latent Dirichlet Allocation (LDA; Blei et al., J Mach Learn Res 3:993–1022, 2003) is a probabilistic topic model that has been used to detect the latent structure of examinees’ responses to constructed-response (CR) items. In general, LDA parameters are estimated using Gibbs sampling or variational expectation maximization (VEM). Relatively little evidence exists, however, regarding the accuracy of either algorithm in the context of educational research, such as small numbers of latent topics, small numbers of documents, short average lengths of documents, and small numbers of unique words. Thus, this simulation study evaluates and compares the accuracy of parameters estimates using Gibbs sampling and VEM in corpora typical of educational tests employing CR items. Simulated conditions include number of documents (300, 700, and 1000 documents), average answer length (20, 50, 100, and 180 words per document), vocabulary of unique words in a corpus (350 and 650 unique words), and number of latent topics (3, 4, 5, 6, and 7 topics). Accuracy of estimation was evaluated with root mean square error. Results indicate both Gibbs sampling and VEM recovered parameter estimates well but Gibbs sampling was more accurate when average text length was small.
更多查看译文
关键词
Gibbs sampling, Variational expectation maximization, Latent Dirichlet allocation
AI 理解论文
溯源树
样例
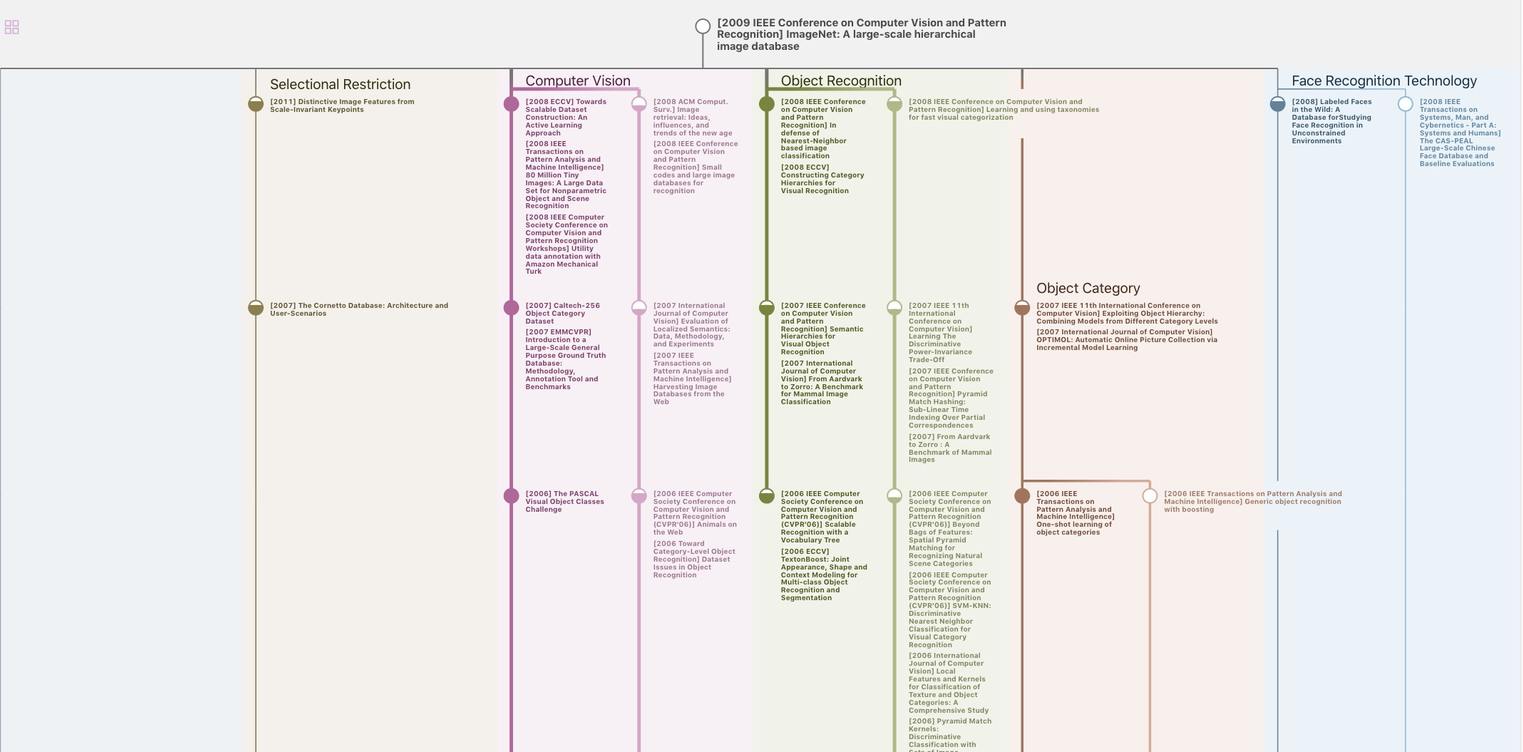
生成溯源树,研究论文发展脉络
Chat Paper
正在生成论文摘要