LSTM-Based Load Prediction for Communication Equipment
Advances in Intelligent Systems, Computer Science and Digital Economics III(2022)
摘要
To reduce the failure rate of communication equipment and to achieve proactive and preventive maintenance of communication equipment, it is very important to grasp the change of the load of communication equipment in a timely and accurate manner. In this paper, a multivariate LSTM neural network-based load prediction model for communication equipment units is proposed. The power influencing factors are extracted by correlation analysis, and the average absolute percentage error is used as the evaluation criterion for the model’s merit to predict the power output of communication equipment units in the future period, which in turn provides a reference basis for the scheduling of communication equipment among operation and maintenance personnel. The results of the case validation show that, compared with traditional deep learning methods, the error of multivariate LSTM neural network in predicting the power of communication equipment is as low as 0.94%, while the error rates of the RNN and GRU method are 3.35% and 2.24% respectively, which proves that the prediction method proposed in this paper is more effective and has universal applicability.
更多查看译文
关键词
Communication equipment, Output prediction, Deep learning, Multivariable long-short term memory
AI 理解论文
溯源树
样例
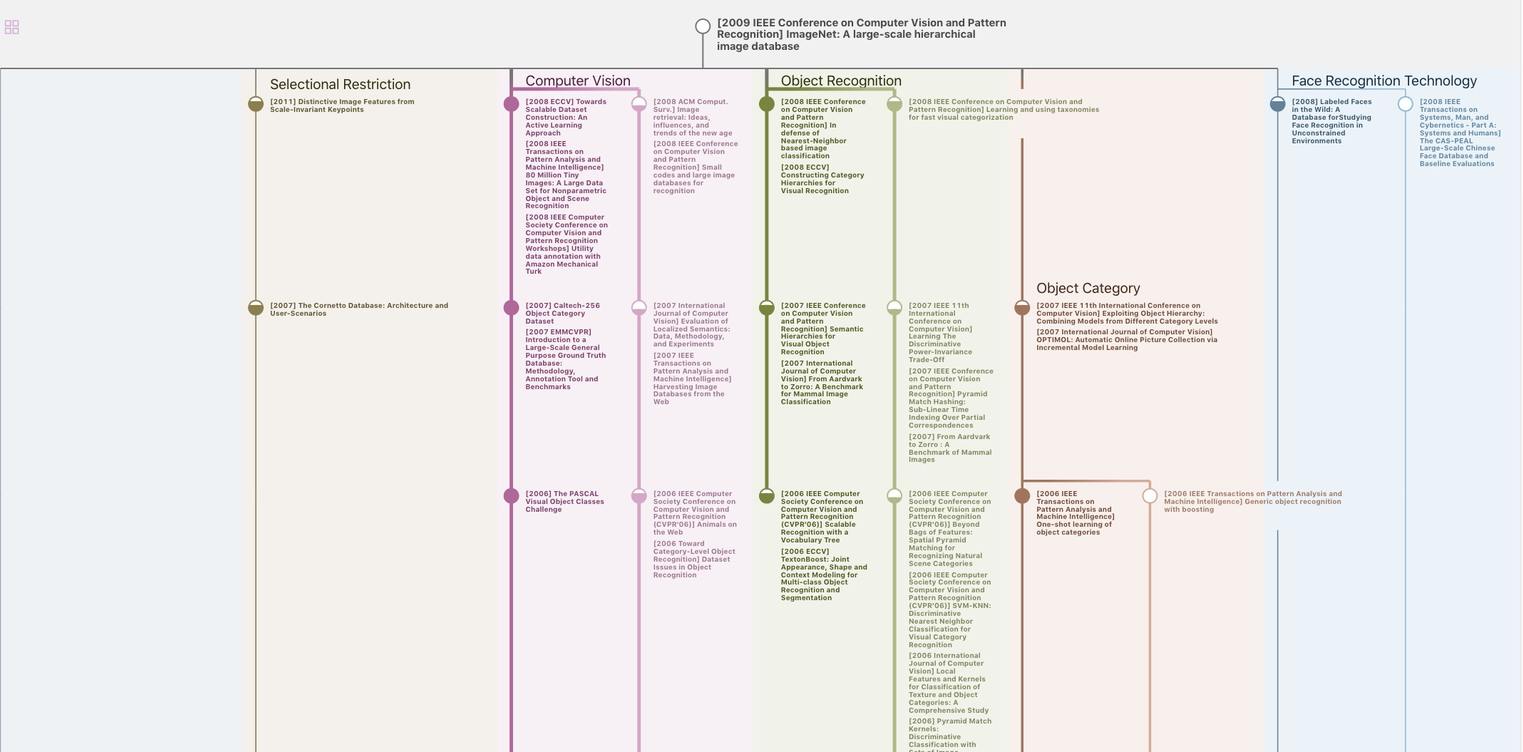
生成溯源树,研究论文发展脉络
Chat Paper
正在生成论文摘要