Video Segmentation and Characterisation to Support Learning
Educating for a New Future: Making Sense of Technology-Enhanced Learning Adoption(2022)
Abstract
The predominance of using videos for learning has become a phenomenon for generations to come. This leads to a prevalence of videos generating and using open learning platforms. However, learners may not be able to detect the main points in the video and relate them to the domain for their study. This can hinder the effectiveness of using videos for learning. To address these challenges, our research aims to develop automatic ways to segment videos, characterise them and finalise the segmentation work by aggregating adjacent segments within a video with the same focus of domain topic(s) or topic-concept(s). We present a framework for automated video segmenting and characterising to support learning (VISC-L). We assume that the domain we are processing videos from has been computationally presented (via ontology). We are using the Deep learning BERT-BASE-Uncased model with a binary classifier to identify the focus topic of each segment. Then we use a semantic tagging algorithm to identify the focus concept within the topic. The adjacent segments within a video with the same focus topic/concept are aggregated to generate the final characterised video segments. We have evaluated the usefulness of watching the identified segments and characterisations compared with video segmentation provided by Google.
MoreTranslated text
Key words
Video-based learning, Video transcript, Text analytics, Domain ontology, Video characterisation, Video aggregation
AI Read Science
Must-Reading Tree
Example
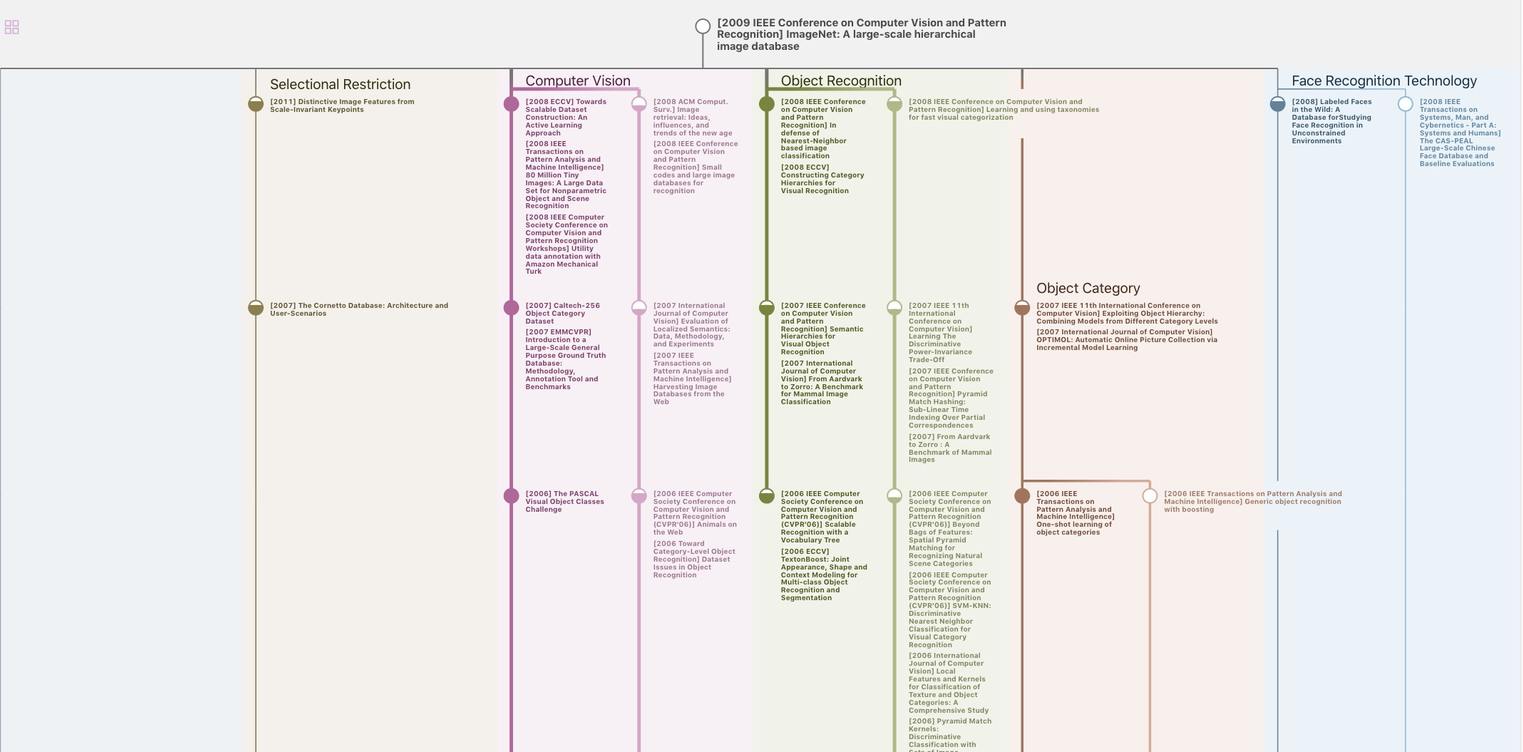
Generate MRT to find the research sequence of this paper
Chat Paper
Summary is being generated by the instructions you defined