Estimating the Temporal Evolution of Synaptic Weights from Dynamic Functional Connectivity
Brain Informatics(2022)
摘要
How to capture the temporal evolution of synaptic weights from measures of dynamic functional connectivity (DFC) between the activity of different simultaneously recorded neurons is an important and open problem in systems neuroscience. To address this issue, we first simulated models of recurrent neural networks of spiking neurons that had a spike-timing-dependent plasticity mechanism generating time-varying synaptic and functional coupling. We then used these simulations to test analytical approaches that relate dynamic functional connectivity to time-varying synaptic connectivity. We investigated how to use different measures of directed DFC, such as cross-covariance and transfer entropy, to build algorithms that infer how synaptic weights evolve over time. We found that, while both cross-covariance and transfer entropy provide robust estimates of structural connectivity and communication delays, cross-covariance better captures the evolution of synaptic weights over time. We also established how leveraging estimates of connectivity derived from entire simulated recordings could further boost the estimation of time-varying synaptic weights from the DFC. These results provide useful information to estimate accurately time variations of synaptic strength from spiking activity measures.
更多查看译文
关键词
Dynamic functional connectivity, Spiking neural network, Communication delay, Transfer entropy, Cross-covariance
AI 理解论文
溯源树
样例
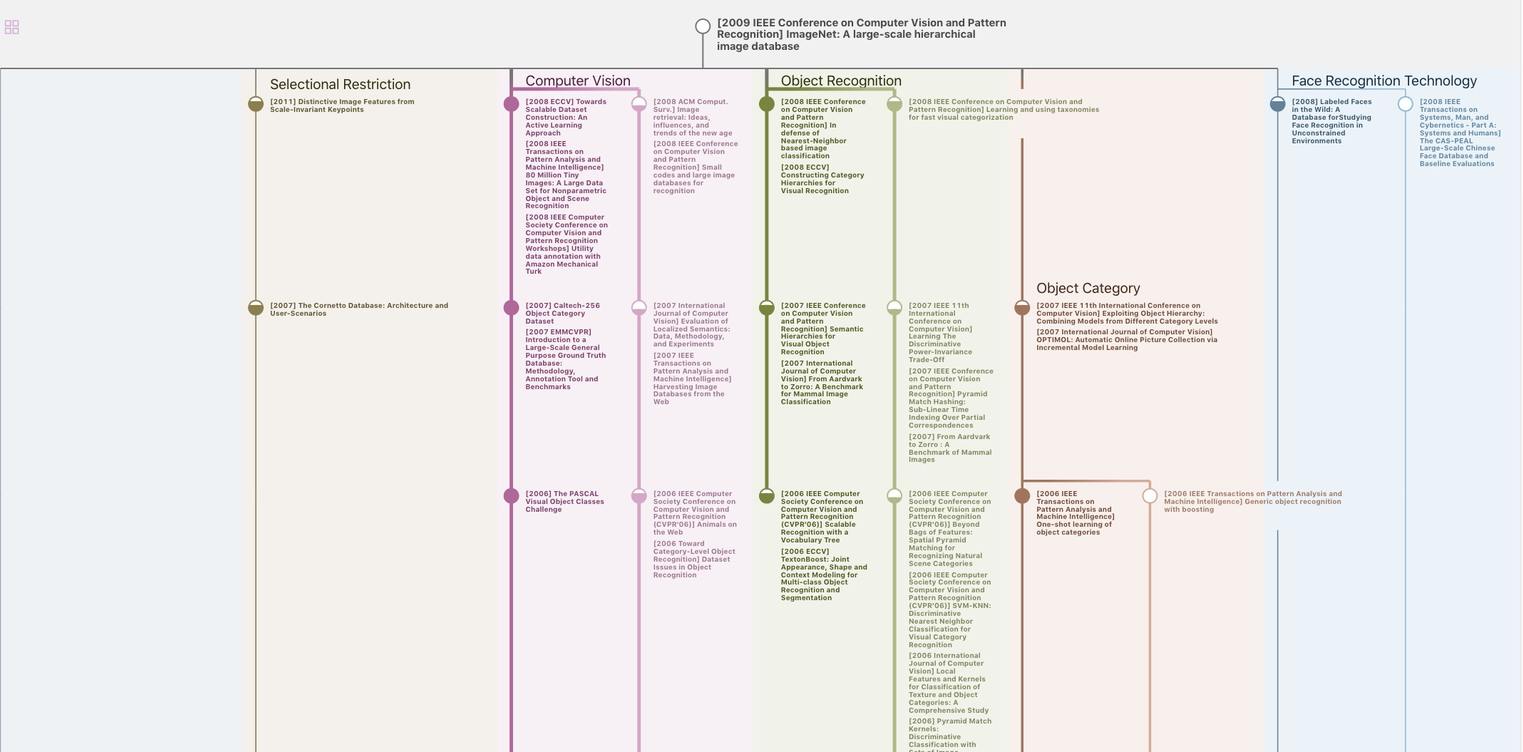
生成溯源树,研究论文发展脉络
Chat Paper
正在生成论文摘要