Study of Different Classifiers and Multi-modal Sensors in Assessment of Workload
Augmented Cognition(2022)
摘要
Cognitive workload changes in the assessment of human performance have long been monitored through the use of subjective self-reports, physiological, or neuro-physiological measures. The purpose of this study is to use multi-modal sensors and machine learning (ML) approach as an assessment method and to investigate which set of biometrics serves as the best objective predictor of cognitive workload. Several biometrics, including prefrontal cortex oxygenation changes, heart rate, and respiratory rate, were collected in both 5-screen and 1-screen immersion conditions to induce high and low task load in simulated use of force (UOF) scenarios. These biometrics were then used to train logistic regression and feature mapping classifiers. Performance of these classifiers was assessed through measures of F1-score and accuracy in order to determine which feature set lends itself to the best prediction power. The classifier trained with left anterior medial prefrontal cortex oxygenation changes performed the best out of individual biometrics, with an accuracy of 0.68 and a f1-score of 0.67. The accuracy of this model was marginally improved to 0.69 when heart rate and oxygenation changes from the other prefrontal cortex quadrants were included, however, the f1-score decreased to 0.51. These results suggest that the logistic regression model underfit the data, necessitating an increase of training data set and exploration of alternative ML approaches in order to use these sensor-driven biometrics effectively and reliably to assess cognitive workload.
更多查看译文
关键词
Human performance, Training, Cognitive workload, Machine learning, fNIRS, Heart rate, Respiratory rate
AI 理解论文
溯源树
样例
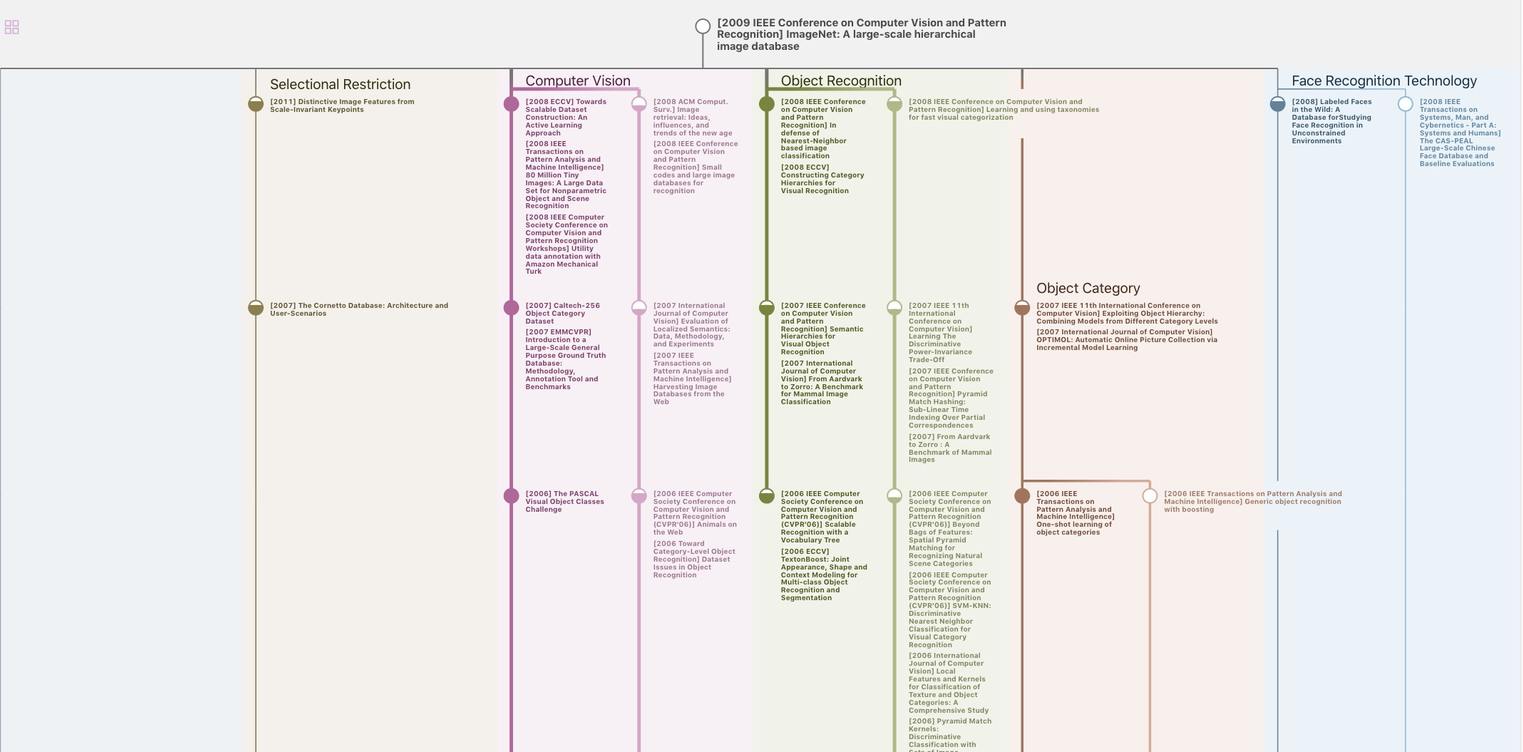
生成溯源树,研究论文发展脉络
Chat Paper
正在生成论文摘要