Architecture and Security Analysis of Federated Learning-Based Automatic Modulation Classification
Frontiers in Cyber Security(2022)
Abstract
Conducting effective deep learning for automatic modulation recognition (AMR) plays a key role in non-cooperative communication systems. Applying federated learning (FL) to train AMR-oriented deep neural networks (ADNN) models is promising in achieving high recognition accuracy while maintaining the data privacy and saving precious wireless transmission bandwidth. In this paper, a FL architecture for ADNN training is presented, and four ADNN models are implemented under the FL architecture. Moreover, to evaluate the security vulnerability of the FL architecture facing ADNN models, multiple adversarial attacks are deployed at the clients to attack the central model training. Besides, label poisoning attacks targeting the FL architecture are investigated. To evaluate the efficiency and vulnerability of the FL architecture for ADNN training, a series of experiments are conducted on an open RF dataset. Results show that ADNN models can achieve high recognition accuracy. Moreover, data or label poisoning attacks are more effective in degrading recognition accuracy than adversarial attacks under the FL architecture.
MoreTranslated text
Key words
Federated learning, Modulation, Classification, Security
AI Read Science
Must-Reading Tree
Example
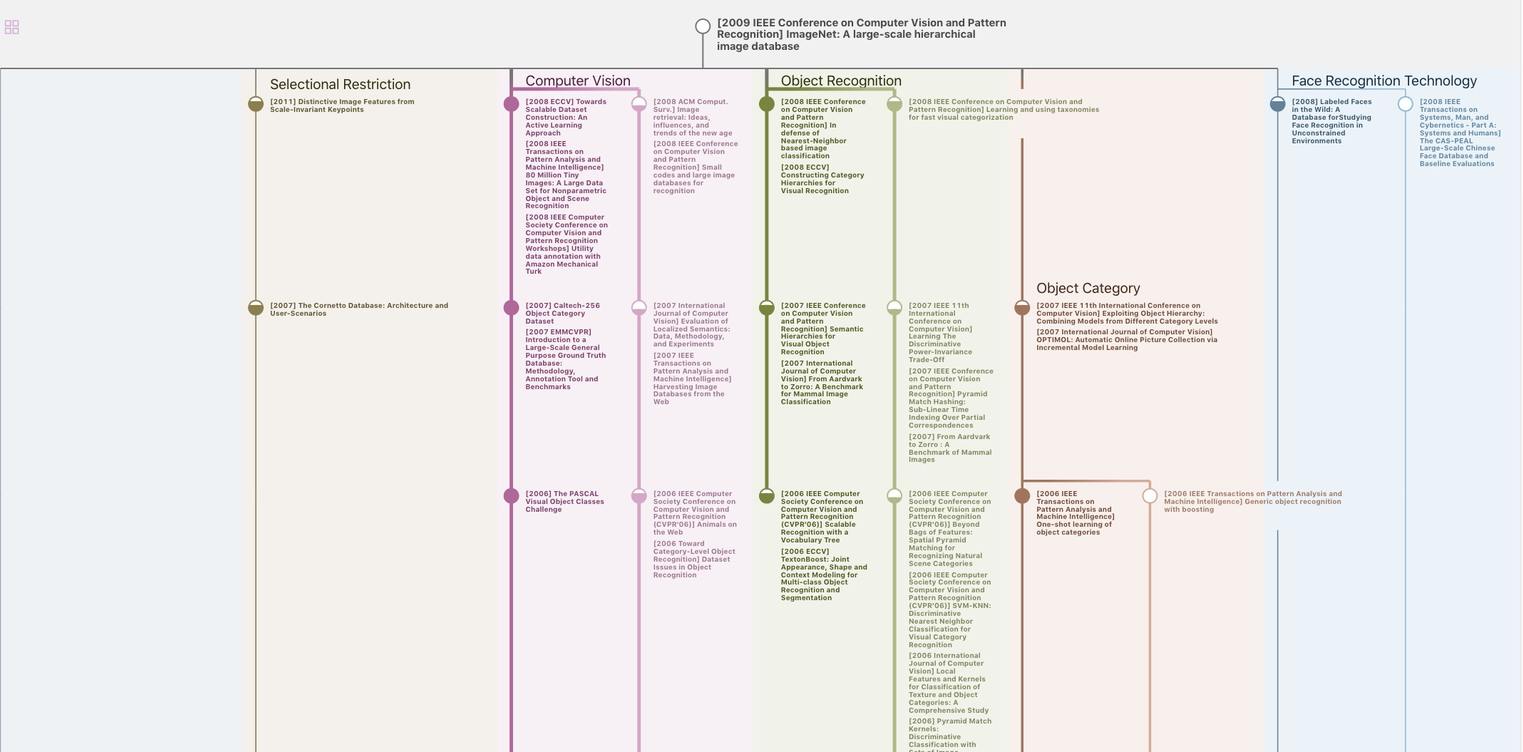
Generate MRT to find the research sequence of this paper
Chat Paper
Summary is being generated by the instructions you defined