MDNCaching: A Strategy to Generate Quality Negatives for Knowledge Graph Embedding
Advances and Trends in Artificial Intelligence. Theory and Practices in Artificial Intelligence(2022)
摘要
Knowledge graph embedding (KGE) has become an integral part of AI as it enables knowledge construction and exploring missing information. KGE encodes the entities and relations (elements) in a knowledge graph into a low-dimensional vector space. The conventional KGE models are trained using positive and negative examples by discriminating the positives from the negatives. However, the existing knowledge graphs contain only the positives. Hence, it is required to generate negatives to train KGE models. This remains a key challenge in KGE due to various reasons. Among them, the quality of the negatives is a critical factor for KGE models to produce accurate embeddings of the observed facts. Therefore, researchers have introduced various strategies such as Bernoulli negative sampling to generate quality negatives that are hard to distinguish from positives. However, fixed negative sampling strategies are suffering from vanishing gradients and false negatives. Later, the dynamic negative sampling techniques were introduced to overcome the vanishing gradient, but the false negatives still remain as a challenge to the research community. The present research introduces a new strategy called MDNCaching (Matrix Decomposed Negative Caching), which generates negatives considering the dynamics of the embedding space while exploring the quality negatives with large similarity scores. Matrix decomposition is used to eliminate false negatives, and hence, the MDNCaching ensures the quality of the generated negatives. The performance of MDNCaching was compared with the existing state-of-art negative sampling strategies, and the results reflect that the proposed negative sampling strategy can produce a notable improvement in existing KGE models.
更多查看译文
关键词
Negative sampling, Knowledge graph embedding, Matrix decomposition
AI 理解论文
溯源树
样例
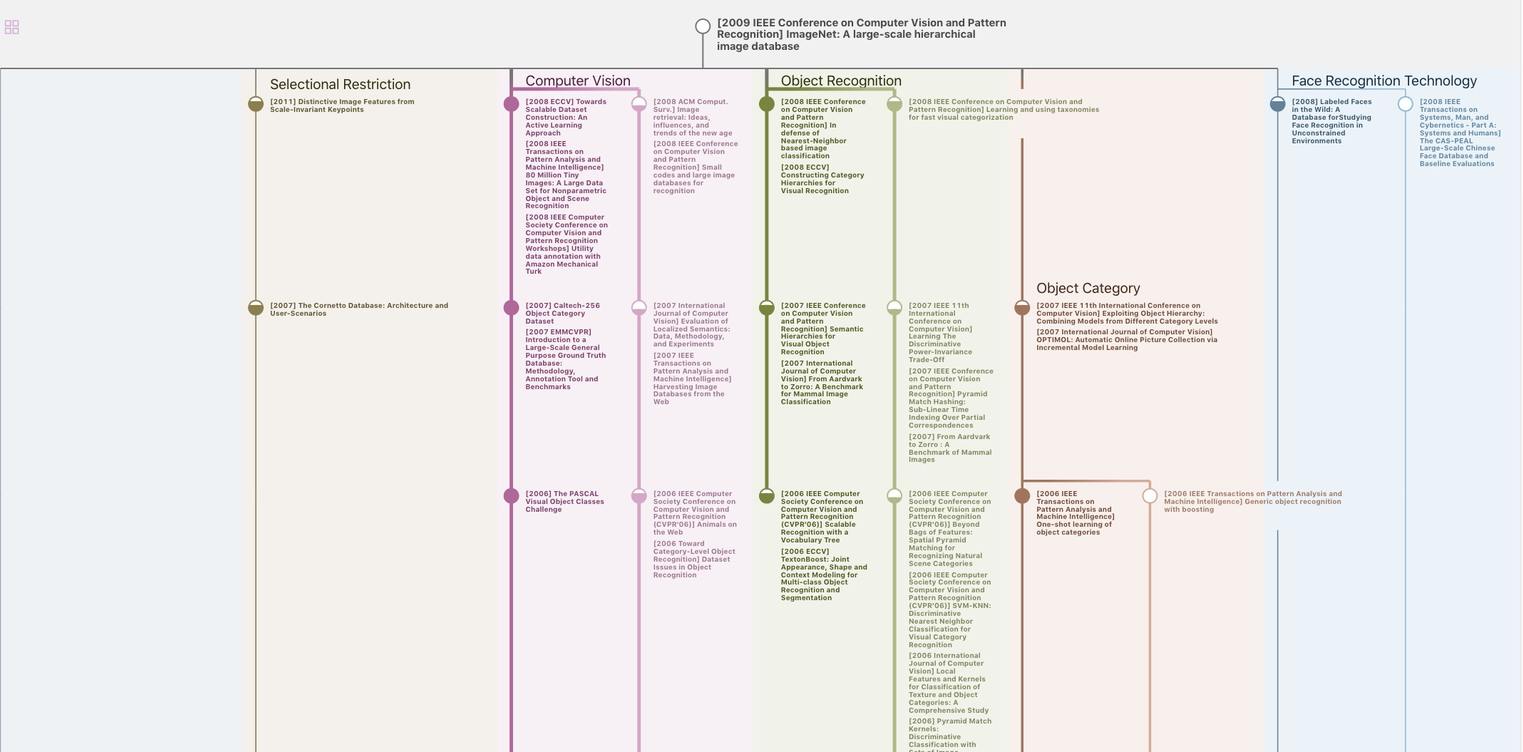
生成溯源树,研究论文发展脉络
Chat Paper
正在生成论文摘要