Contrastive Re-localization and History Distillation in Federated CMR Segmentation
Medical Image Computing and Computer Assisted Intervention – MICCAI 2022(2022)
摘要
Federated learning (FL) has shown value in multi-center multi-sequence cardiac magnetic resonance (CMR) segmentation, due to imbalanced CMR distributions and privacy preservation in clinical practice. However, the larger heterogeneity among multi-center multi-sequence CMR brings challenges to the FL framework: (1) Representation bias in the model fusion. The FL server model, which is generated by an average fusion of heterogeneous client models, is biased to representation close to the mean distribution and away from the long-distance distribution. Hence, the FL has poor representation ability. (2) Optimization stop in the model replacing. The heterogeneous server model replacing client model in FL directly causes the long-distance clients to utilize worse optimization to replace the original optimization. The client has to recover the optimization with the worse initialization, hence it lacks the continuous optimization ability. In this work, a cross-center cross-sequence medical image segmentation FL framework (FedCRLD) is proposed for the first time to facilitate multi-center multi-sequence CMR segmentation. (1) The contrastive re-localization module (CRL) of FedCRLD enables the correct representation from the heterogeneous model by embedding a novel contrastive difference metric of mutual information into a cross-attention localization transformer to transfer client-correlated knowledge from server model without bias. (2) The momentum distillation strategy (MD) of FedCRLD enables continuous optimization by conducting self-training on a dynamically updated client momentum bank to refine optimization by local correct optimization history. FedCRLD is validated on 420 CMR images 6 clients from 2 public datasets scanned by different hospitals, devices and contrast agents. Our FedCRLD achieves superior performance on multi-center multi-sequence CMR segmentation (average dice 85.96%).
https://github.com/JerryQseu/FedCRLD.
更多查看译文
关键词
Federated learning, Contractive learning, Momentum distillation
AI 理解论文
溯源树
样例
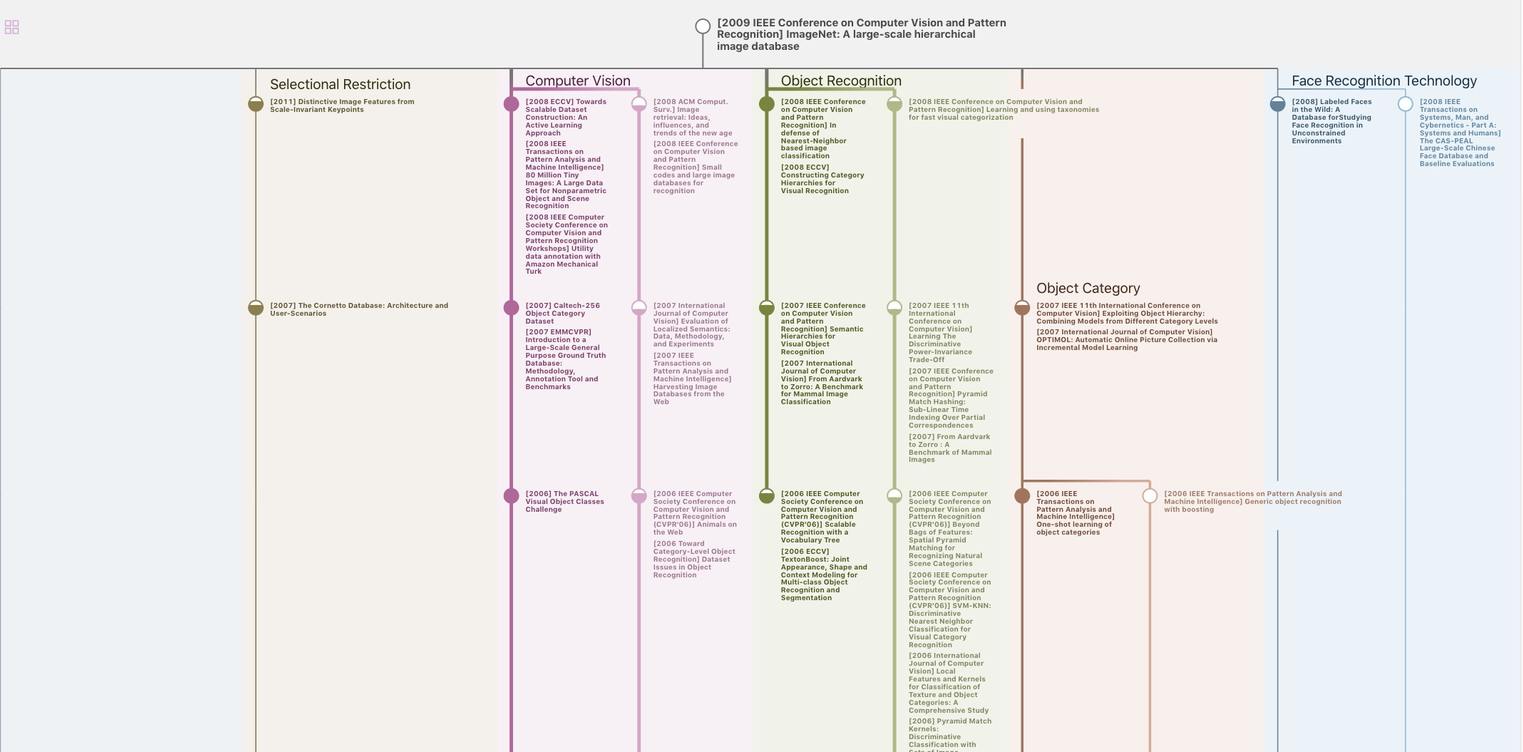
生成溯源树,研究论文发展脉络
Chat Paper
正在生成论文摘要