MBDSNet: Automatic segmentation of kidney ultrasound images using a multi-branch and deep supervision network
Digital Signal Processing(2022)
Abstract
Ultrasound imaging has been widely used in the screening of kidney diseases. The segmentation of the kidney in ultrasound images can aid in the diagnosis of clinical diseases. However, segmenting the kidney from ultrasound images is challenging, due to the effects of heterostructure, image quality, and other factors. In this paper, a multi-branch and deep supervision network (MBDSNet) is proposed to segment the kidney from ultrasound images. Specifically, the architecture consists of two parts: multi -branch encoding network and refined decoding network. The multi-branch encoding network consists of a multi-scale feature input pyramid and three branch encoders. The multi-branch encoding network can reduce the sensitivity of the network to the input data through the multi-scale feature inputs pyramid, and utilize the information exchange between the three branch encoders can improve the generalization ability of the network. In the refined decoding network, the introduction of the side-out deep supervision module and the kidney boundary detection module can further guide the network to effectively segment the kidney with complete contour. Objective comparisons with several state-of-the-art segmentation methods on six commonly used metrics are performed. The method proposed in this paper has achieved the best results in terms of evaluation indicators and segmentation results.(c) 2022 Elsevier Inc. All rights reserved.
MoreTranslated text
Key words
Kidney ultrasound,Automatic segmentation,Multi-branch,Deep supervision,Boundary detection
AI Read Science
Must-Reading Tree
Example
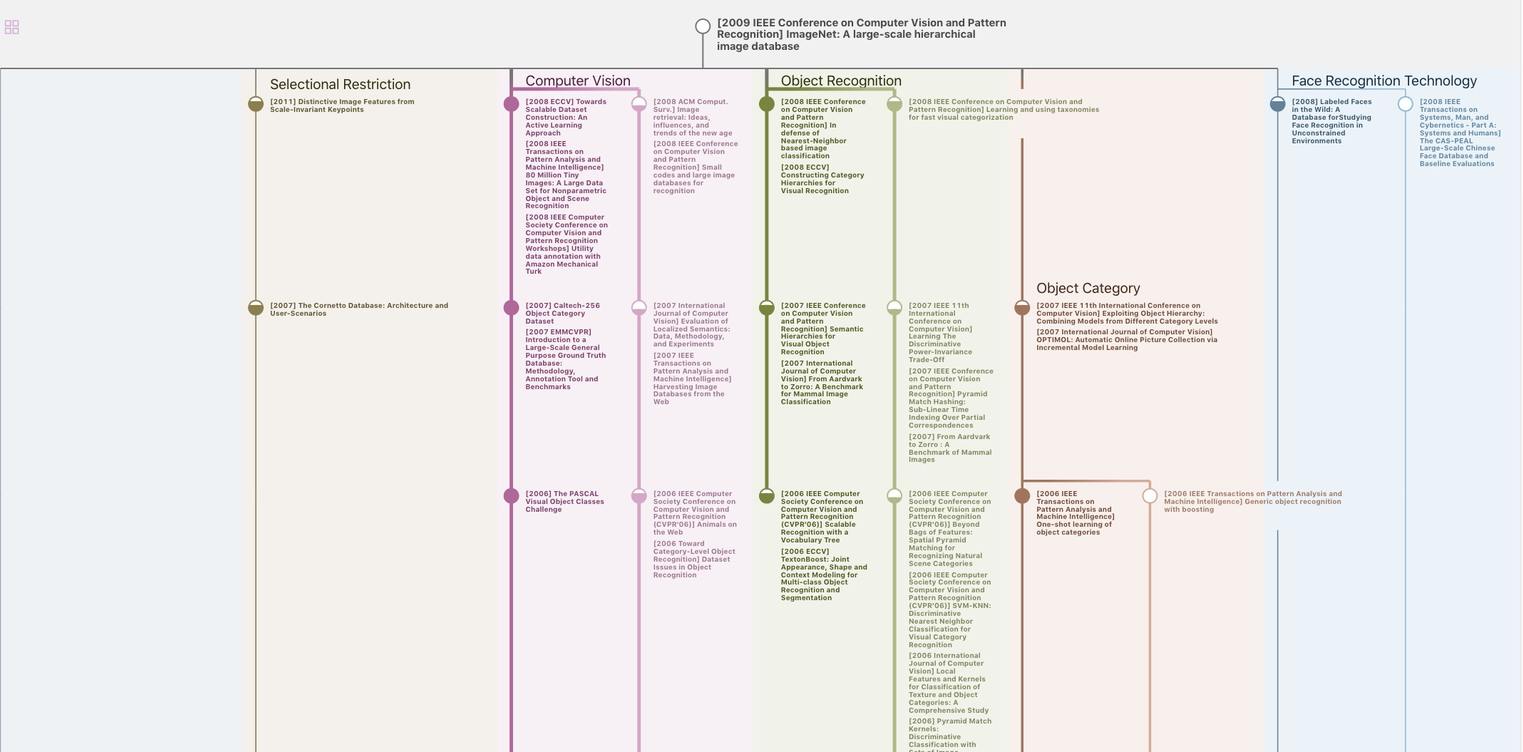
Generate MRT to find the research sequence of this paper
Chat Paper
Summary is being generated by the instructions you defined