Research on Bearing Fault Recognition Based on PSO-MCKD and 1D-CNN
The Proceedings of the 2021 Asia-Pacific International Symposium on Aerospace Technology (APISAT 2021), Volume 1(2022)
摘要
This paper proposes a bearing fault feature extraction and recognition method based on particle swarm optimization optimization Maximum Correlated Kurtosis Deconvolution (MCKD) and one-dimensional convolutional neural network to solve the non-stationarity of rolling bearing fault signals, Non-linear and complex characteristics, as well as the problems of noise interference and unclear fault characteristics in the process of fault identification. First, the multi-channel signals of the rolling bearing is analyzed, in order to select the signal containing the impact component as the fault feature. Next, the signal containing the fault feature is filtered through MCKD, where the best parameters of MCKD are obtained by improving the particle swarm algorithm to achieve the feature enhancement of the main signal. Finally, a one-dimensional convolutional neural network (One Dimensional Convolutional Neural Network, 1D-CNN) is used to model the characteristic signals under different damage conditions in order to obtain the fault recognition model of the rolling bearing.The experimental results show that the method can effectively extract the main characteristic signals of the faulty bearing, and realize the accurate identification of the bearing fault in the noisy environment.
更多查看译文
关键词
Rolling bearings, Particle swarm optimization algorithm, Maximum correlation kurtosis deconvolution, One-dimensional convolutional neural network
AI 理解论文
溯源树
样例
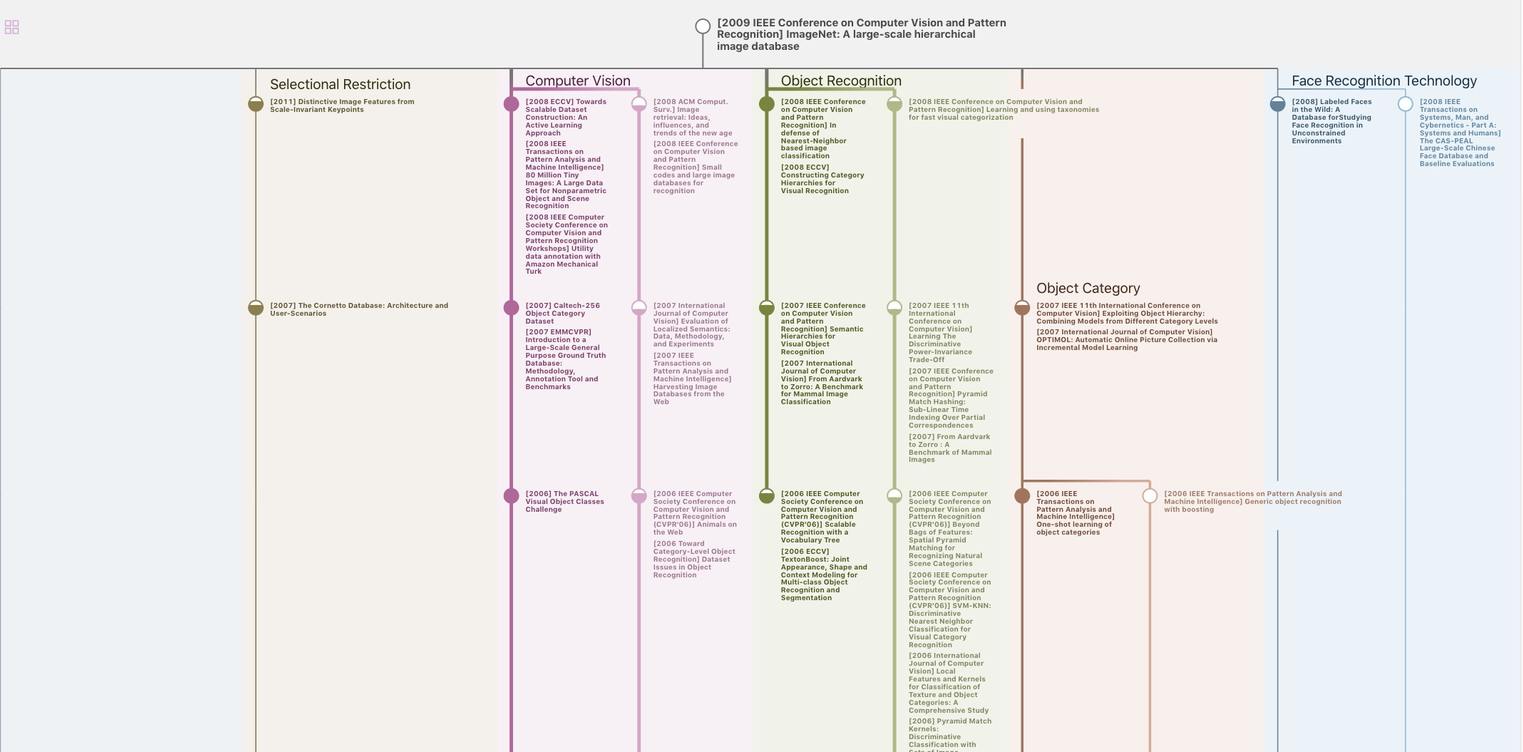
生成溯源树,研究论文发展脉络
Chat Paper
正在生成论文摘要