Topic Modelling Based Semantic Search
Data Management, Analytics and Innovation(2022)
摘要
In today’s world, information is needed to be retrieved from plethora of publications and papers for scientific paper writing, paper reviewing. Retrieval of relevant data has become a very tedious job. The novelty of this paper is that we are providing a holistic approach to retrieve information or abstracts from a corpus of published papers and artifacts, by improving the conventional methods of searching abstracts. To get a better search result, reviewer can provide phrases, sentences and even a complete abstract. This brings to the reviewer the most relevant abstracts in any huge database of publications. To accomplish this, we have implemented topic modelling based semantic search. Various AI algorithms are used to find the closest and nearest abstracts to any given search abstract. For this paper we have extensively used the PUBMED data. Along with search abstracts we are also providing other inherent features which may get unnoticed in huge corpus, like similar words, relation between words and phrases.
更多查看译文
关键词
BERT, Bio-Bert, GENSIM, LDA, Network analysis, Nearest neighbor, NLP, Semantic search, Topic modelling
AI 理解论文
溯源树
样例
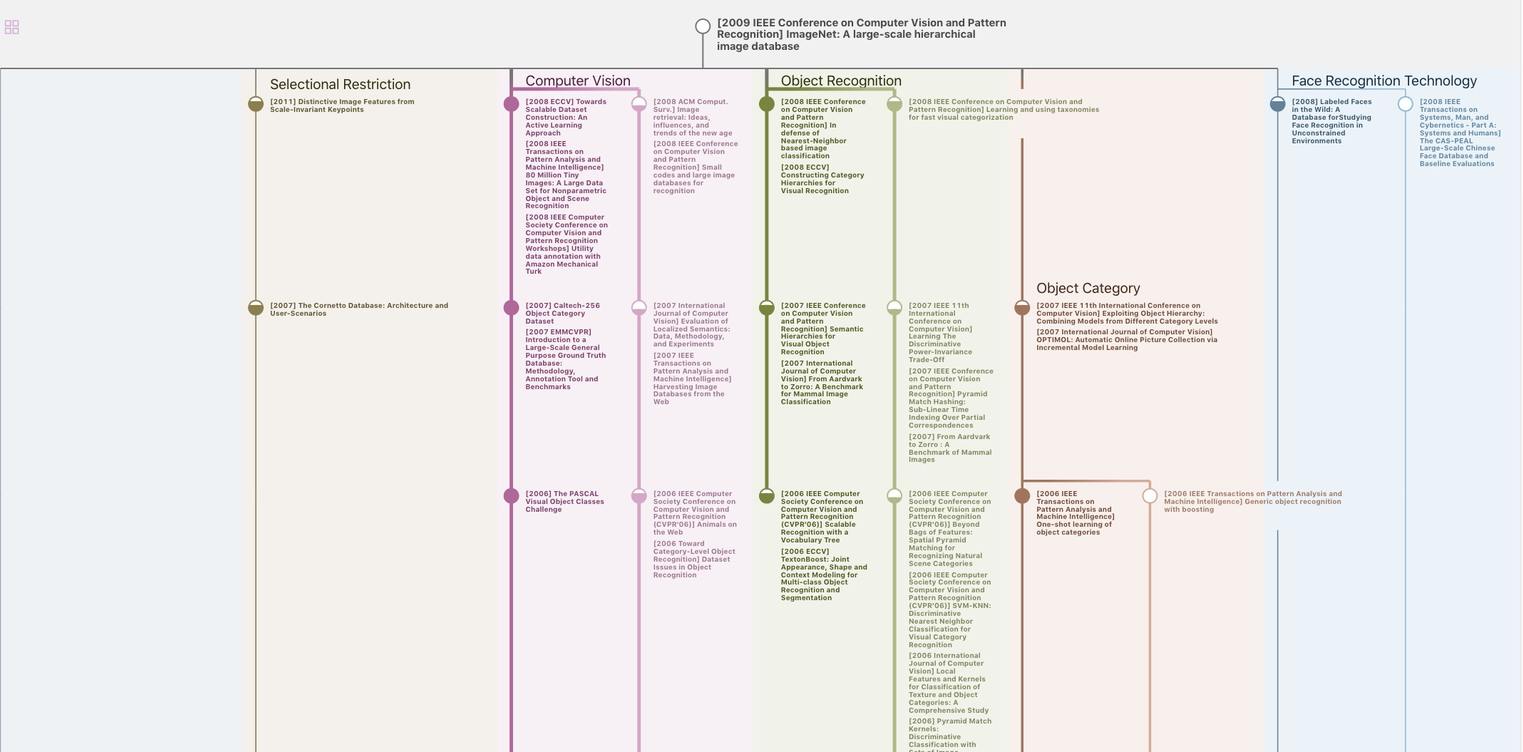
生成溯源树,研究论文发展脉络
Chat Paper
正在生成论文摘要